ADNet: Rethinking the Shrunk Polygon-Based Approach in Scene Text Detection
IEEE TRANSACTIONS ON MULTIMEDIA(2023)
摘要
To localize text regions and separate close instances, the shrunk polygon is widely used in recent scene text detection methods. However, there exist two problems: 1) Existing methods fail to consider the aspect ratio sensitive problem when reconstructing the text instance from shrunk polygon. 2) Texts with extreme aspect ratios will lead to the fracture of shrunk polygons. To handle these two problems, in this paper, we propose a novel Adaptive Dilation Network (ADNet) to focus on the reconstruction process from shrunk polygon, which aims to provide a tight and complete text representation. Firstly, instead of using a fixed dilation factor, ADNet uses an aspect ratio-wise dilation factor to reconstruct the text region from shrunk polygon for each text instance. Such an instance-wise dilation factor considers the scale correlation between the original and shrunk polygon, and thus can guide an adaptive text region reconstruction for texts with large aspect ratio variance. Secondly, to deal with the fracture of detection results, a new Efficient Spatial Relationship Module (ESRM) is devised to capture long-range dependencies with low computation cost. ESRM uses a novel Weighted Pooling to reduce the resolution of feature maps without much information loss. Compared with the existing methods, ADNet further explores the potential of shrunk polygon-based approaches and obtains excellent detection results at an impressive speed. Extensive experiments on several datasets (Total-Text, CTW1500, MSRA-TD500 and ICDAR2015) verify the superiority of our method.
更多查看译文
关键词
Kernel,Shape,Costs,Convolution,Adaptive systems,Text recognition,Synthetic aperture sonar,Scene text detection,shrunk polygon,aspect ratio,adaptive dilation factor
AI 理解论文
溯源树
样例
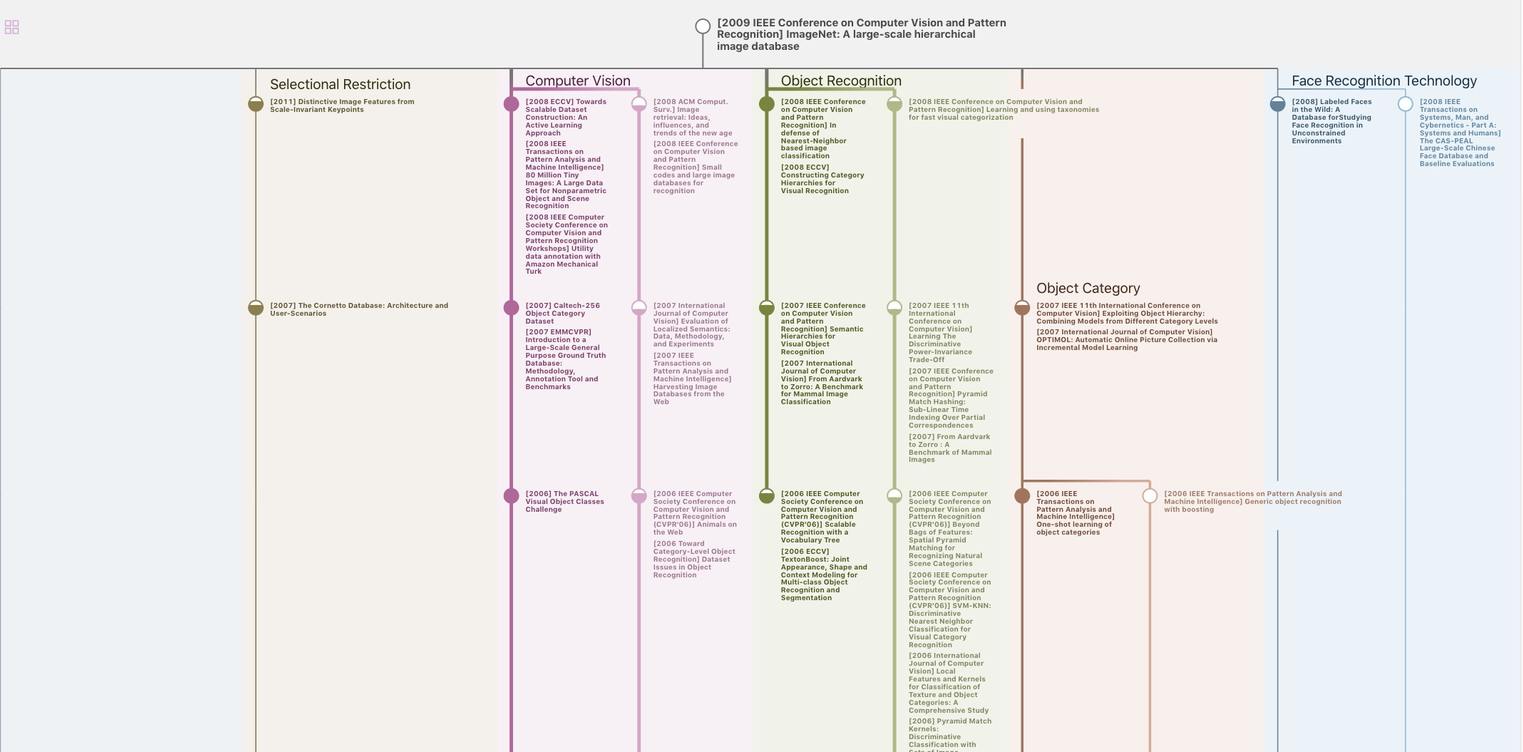
生成溯源树,研究论文发展脉络
Chat Paper
正在生成论文摘要