Very short-term solar ultraviolet-A radiation forecasting system with cloud cover images and a Bayesian optimized interpretable artificial intelligence model
EXPERT SYSTEMS WITH APPLICATIONS(2024)
摘要
High-dose single exposures of long-wavelength ultraviolet-A (UV-A) radiation may trigger severe biological and skin tissue damage in humans and animals, as well as photosynthetic damage in plants. In humans, the highly abundant UV-A is also linked to an increased risk of skin cancer. This paper develops a new forecasting framework tailored for very short-term UV-A predictions using a Bayesian optimized ensemble Neural Basis Expansion Analysis for Interpretable Time Series (B-E-NBEATS) method, integrated with cloud cover predictors and the solar zenith angle. The design phase of the proposed model entails feature selection using an efficient neighborhood component analysis algorithm and hyperparameter tuning with a Bayesian optimizer. To further enhance the predictive performance and reliability, the B-E-NBEATS model is calibrated via uncertainty quantification by adopting an ensemble approach with single-point models trained on three key loss functions. The prescribed model generates interpretable forecasts of 20-minute ahead UV-A by empowering the ensemble members to learn seasonal and trend components in UV-A datasets. With respect to the four benchmark models, the statistical metrics and visual infographics of predicted and observed UV-A reveal a superior forecasting capability of the proposed B-E-NBEATS model. The model yields a comparatively high correlation coefficient of 0.910, 0.869, 0.933, and 0.900 for the spring, summer, autumn, and winter testing phases, respectively. The superior performance by the newly designed hybrid ensemble model ascertains its potential utility in UV-A monitoring and mitigating subsequent harmful exposure risk for the general public, and animal and plant life.
更多查看译文
关键词
UV-A forecast,Cloud cover,Deep ensemble,Uncertainty quantification,N-BEATS model
AI 理解论文
溯源树
样例
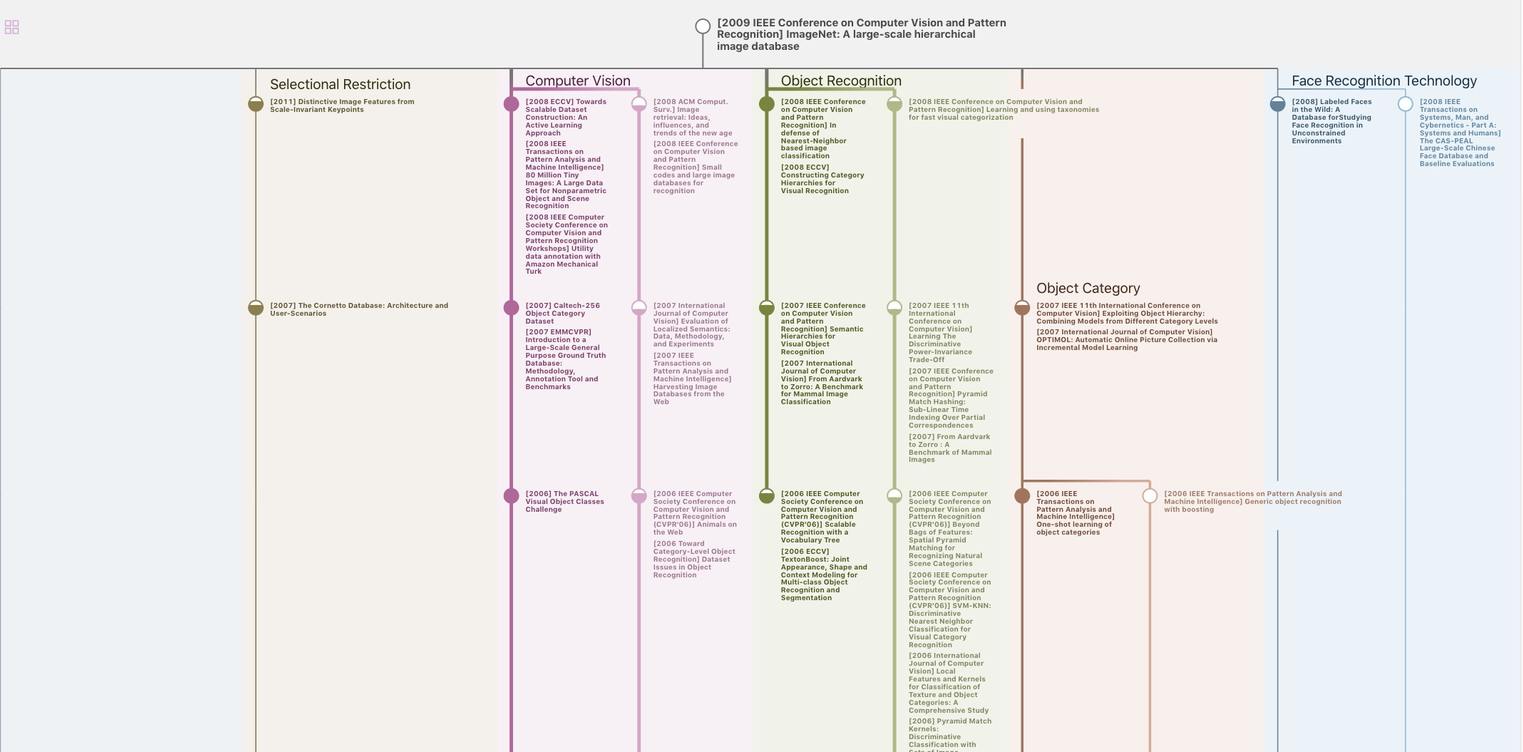
生成溯源树,研究论文发展脉络
Chat Paper
正在生成论文摘要