A Diversified Attention Model for Interpretable Multiple Clusterings
IEEE Transactions on Knowledge and Data Engineering(2023)
摘要
Multiple clusterings can explore the same set of data from different perspectives by discovering different and meaningful clusterings. However, most, if not all, of the existing approaches overwhelmingly focus on the diversity between clustering subspaces, and pay much less attention on the salience of the subspaces. As a consequence, the quality of the produced clusterings is an understudied aspect of the problem. Furthermore, existing methods cannot explain the unique internal subspace structure of each clustering, and cannot incorporate multi-facet knowledge to generate different clusterings. In this paper, we propose a solution named
iMClusts
(
i
nterpretable
M
ultiple
Clust
ering
s
by diversified attention). iMClusts makes use of the expressive representational power of deep autoencoders and multi-head attention to generate multiple salient embedding matrices, and multiple clusterings therein. In addition, it leverages multi-facet knowledge and enhances the diversity between heads to boost the quality and diversity of multiple clusterings. Experimental results on benchmark datasets show that iMClusts can generate multiple clusterings with quality, interpretability, and diversity.
更多查看译文
关键词
Terms-Multiple clusterings, multi-head attention, multi-facet knowledge, interpretability, quality and diversity
AI 理解论文
溯源树
样例
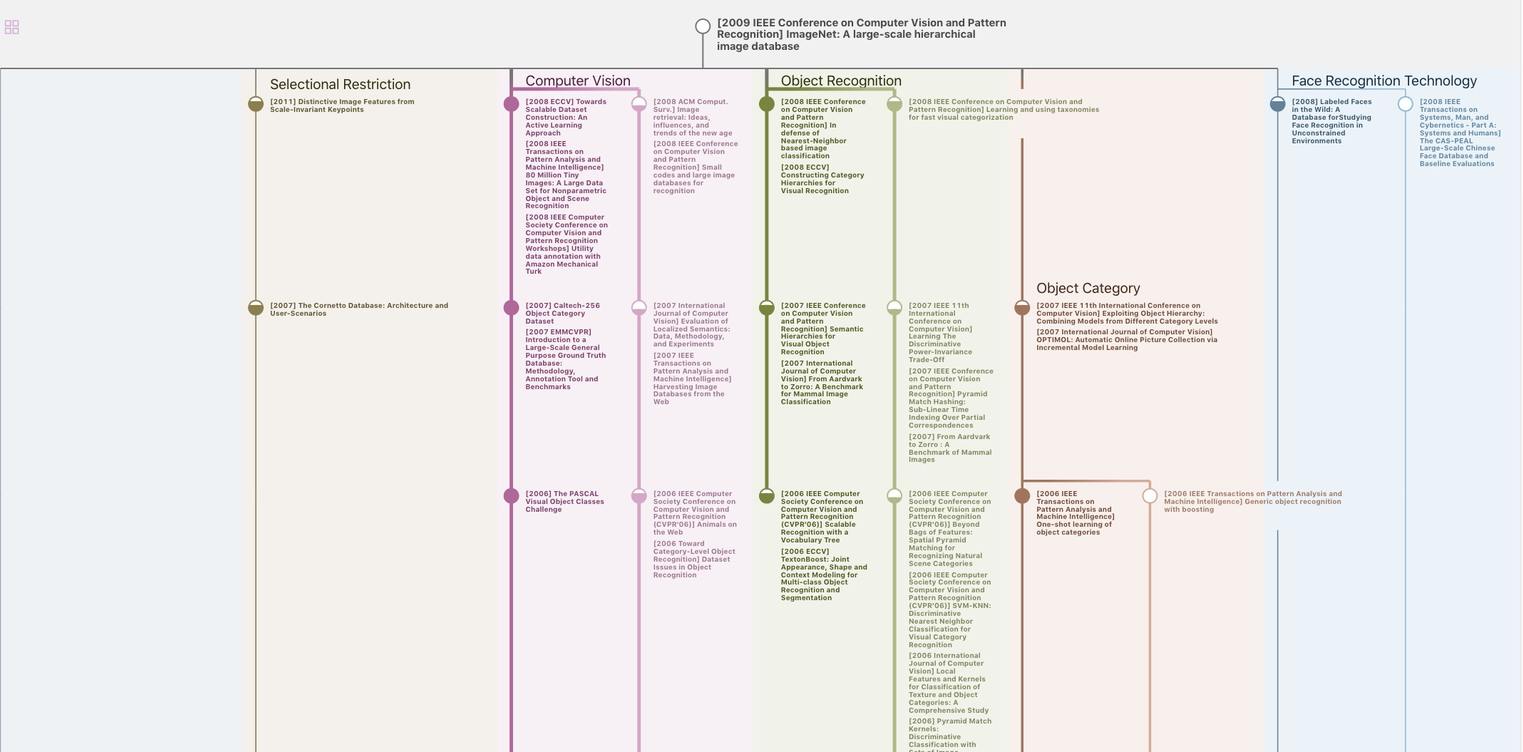
生成溯源树,研究论文发展脉络
Chat Paper
正在生成论文摘要