Semantic Segmentation Network Based on Lightweight Feature Pyramid Transformer
2022 37th Youth Academic Annual Conference of Chinese Association of Automation (YAC)(2022)
摘要
Transformer has excellent global expression ability. Recently, researchers have proposed many Transformer-based image semantic segmentation networks, and most of them have achieved considerable results. However, they ignore the multiscale modeling of the decoder, multi-scale feature representation is crucial for segmenting objects of different scales. To improve the decoder of the transformer network for semantic segmentation, we propose a lightweight feature pyramid Transformer. Specifically, an up-sampling method of feature pixel rearrangement is proposed to up-sample high-level features, and feature pyramid fusion is performed to form a rough multi-scale representation; secondly, a lightweight multi-head attention with multi-level feature fusion is proposed. The coarse multiscale features are refined, and the multi-head attention allows the model to focus on the differences between scales and learn the subspace information of each scale from each other. Therefore, a unified image semantic segmentation network is formed, which can capture contextual information at multiple feature scales with only a small increase in computational overhead. Our method is effectively validated on Cityscapes and ADE20K datasets and achieves good results.
更多查看译文
关键词
Semantic Segmentation,Transformer,Feature Pyramid,Multi-scale Representation
AI 理解论文
溯源树
样例
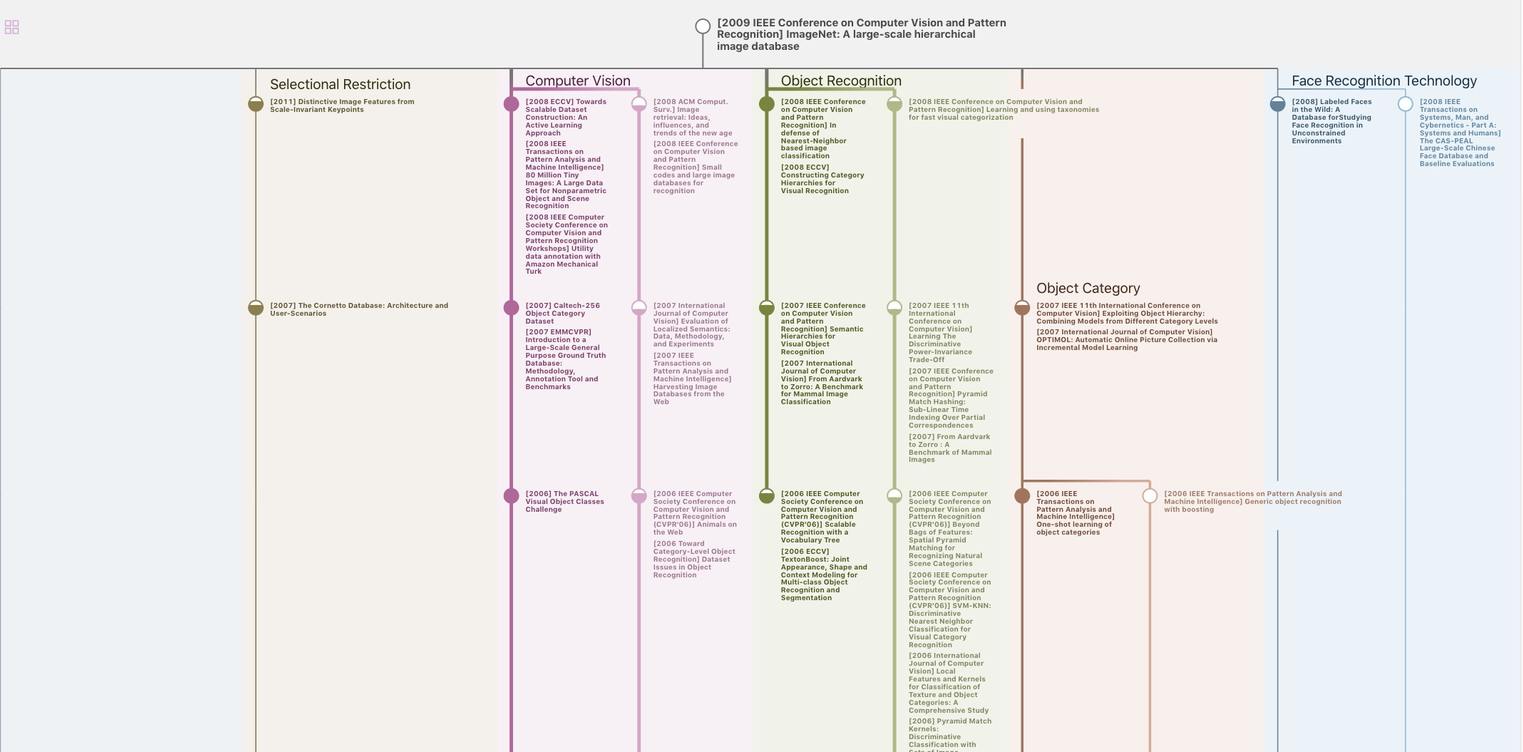
生成溯源树,研究论文发展脉络
Chat Paper
正在生成论文摘要