Semi-Supervised Crowd Counting With Spatial Temporal Consistency and Pseudo-Label Filter
IEEE Transactions on Circuits and Systems for Video Technology(2023)
摘要
Semi-supervised crowd counting (SSCC) aims to learn a crowd counting model with limited labeled images and a large number of unlabeled images. Previous works leverage unlabeled images by pseudo-labeling and spatial consistency regularization paradigms, which frequently adopt teacher-student frameworks. However, their performances are readily degraded due to the inconsistent and unreliable pseudo density map in complex crowd scenes. Here, we argue that the SSCC performance can be significantly improved by reducing the over-fitting of the incorrect pseudo labels, and a novel spatial-temporal consistency framework, named STC-Crowd, is proposed. Under different spatial perturbations, spatial consistency enables the counting model to output consistent predictions for the same crowd image. Temporal consistency generates similar feature embedding over adjacent training stages, alleviating the inconsistent issues of pseudo density maps generated for the same image over time. To store the temporal feature embedding of different density levels for temporal consistency, a dynamic temporal knowledge memory (DTKM) is deliberately designed, and considerably reduces the storage cost. Besides, a pseudo-label filter (PLF) mechanism is used to alleviate the negative impact of incorrect pseudo density maps, by reducing the supervision weights of unreliable pseudo labels with high uncertainty. Extensive experiments on four benchmark datasets show that our method obtains competent performance against leading SSCC methods, and especially works better on limited labeled images.
更多查看译文
关键词
Semi-supervised crowd counting,spatial-temporal consistency,temporal knowledge memory,pseudo-label filter
AI 理解论文
溯源树
样例
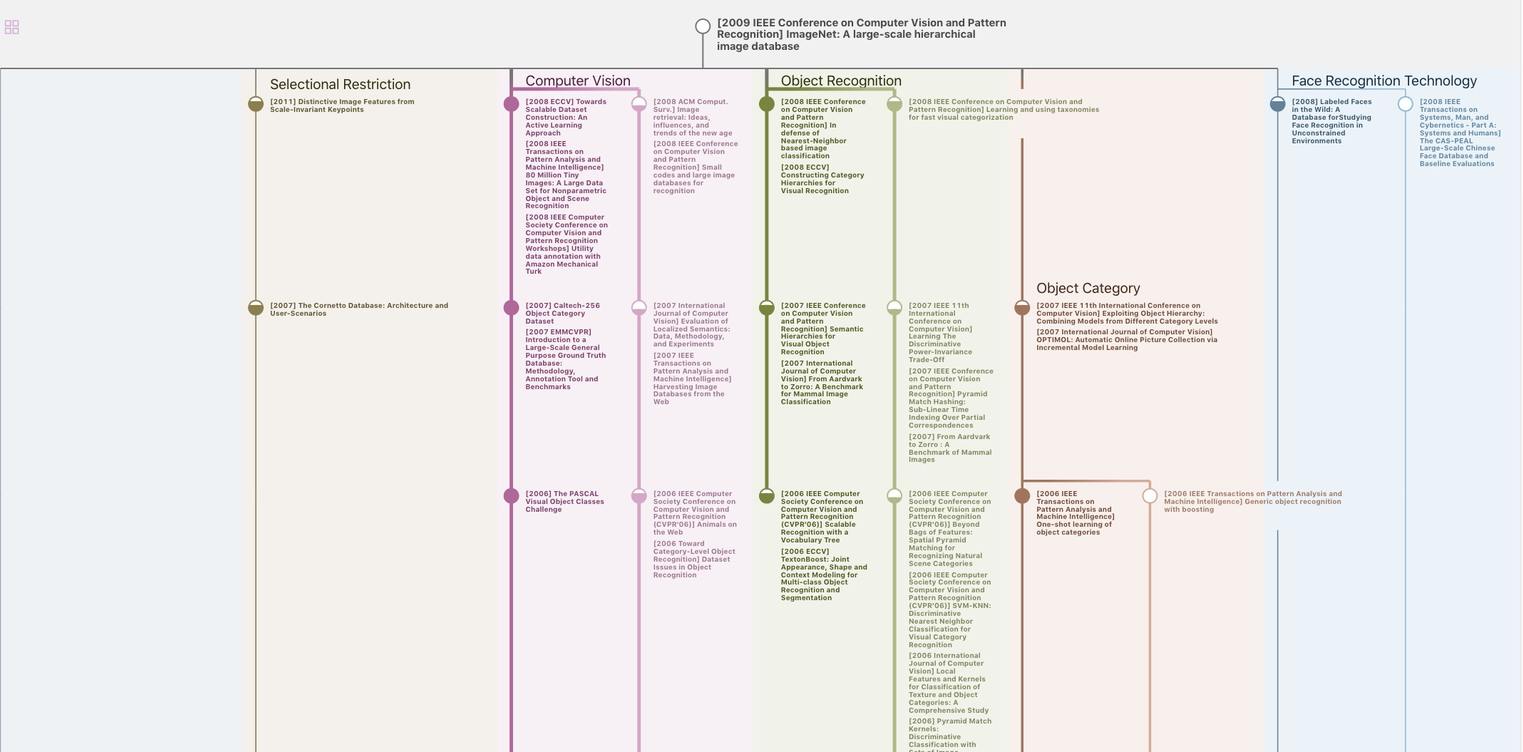
生成溯源树,研究论文发展脉络
Chat Paper
正在生成论文摘要