Depressive Symptoms and Their Mechanisms: An Investigation of Long-term Patterns of Interaction Through a Panel Network Approach
crossref(2023)
摘要
Within-person network dynamics on a monthly or yearly level have been difficult to study due to the lack of suitable analytic methods. In this study, a new method for estimating networks from panel data was used to investigate how symptoms of depression, mechanisms proposed in the meta-cognitive therapy (MCT) model, and loneliness interact across a nine-month period. Four data waves were delivered by a representative population sample of 4,361 participants during the COVID-19 pandemic in Norway. Networks were estimated using the panel graphical vector-autoregression (panelgvar) method. In the temporal network, use of substance to cope with negative feelings positively predicted threat monitoring and depressed mood. In turn, threat monitoring positively predicted suicidal ideation. Meta-cognitive beliefs that thoughts and feelings are dangerous positively predicted anhedonia. Suicidal ideation positively predicted sleep problems and worthlessness. Loneliness was positively predicted by depressed mood. In turn, more loneliness predicted more control of emotions. In the contemporaneous network, worry/rumination was positively related to depressed mood, worthlessness, and sleep problems. In conclusion, the findings indicate the importance of the theory-derived variables threat monitoring, beliefs that thoughts and feelings are dangerous, and substance use as potential targets for intervention to alleviate long-term depressive symptoms. Suicidal ideation may have a detrimental long-term effect on worthlessness. The results also suggest that depressed mood leads to loneliness in the long run but does not support that loneliness leads to depressive symptoms.
更多查看译文
AI 理解论文
溯源树
样例
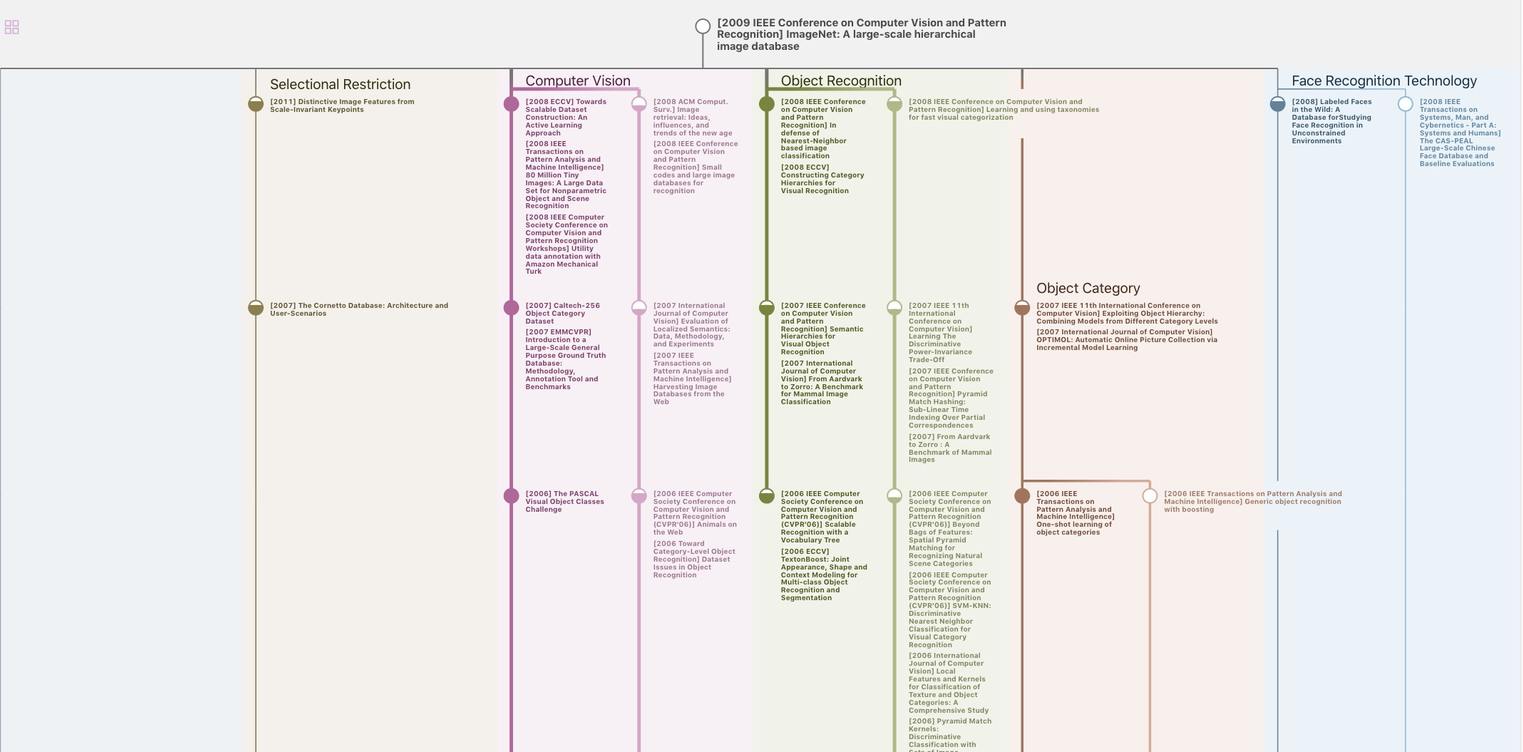
生成溯源树,研究论文发展脉络
Chat Paper
正在生成论文摘要