ReAFM: A Reconfigurable Nonlinear Activation Function Module for Neural Networks
IEEE Transactions on Circuits and Systems II: Express Briefs(2023)
摘要
Deep neural networks (DNNs) with various nonlinear activation functions (NAFs) have achieved unprecedented successes, sparking interest in efficient DNN hardware implementation. However, most existing NAF implementations focus on one type of functions with dedicated architectures, which is not suited for supporting versatile DNN accelerators. In this brief, based on a proposed reciprocal approximation optimization (RAO) method, an efficient reconfigurable nonlinear activation function module (ReAFM) is devised to implement various NAFs. The computational logic and dataflow of certain NAFs are merged and reused to minimize hardware consumption by leveraging the correlations among different NAFs. In addition, a precision adjustable exponential unit (PAEU) is developed to obtain a good tradeoff between the approximation accuracy and hardware cost. Compared to the prior art, the experimental results demonstrate that the proposed ReAFM can support many more NAF types with comparable or even better performance. Furthermore, evaluation results on some prevalent neural networks show that the proposed approximation method causes negligible accuracy loss (< 0.1%).
更多查看译文
关键词
neural networks,activation,function
AI 理解论文
溯源树
样例
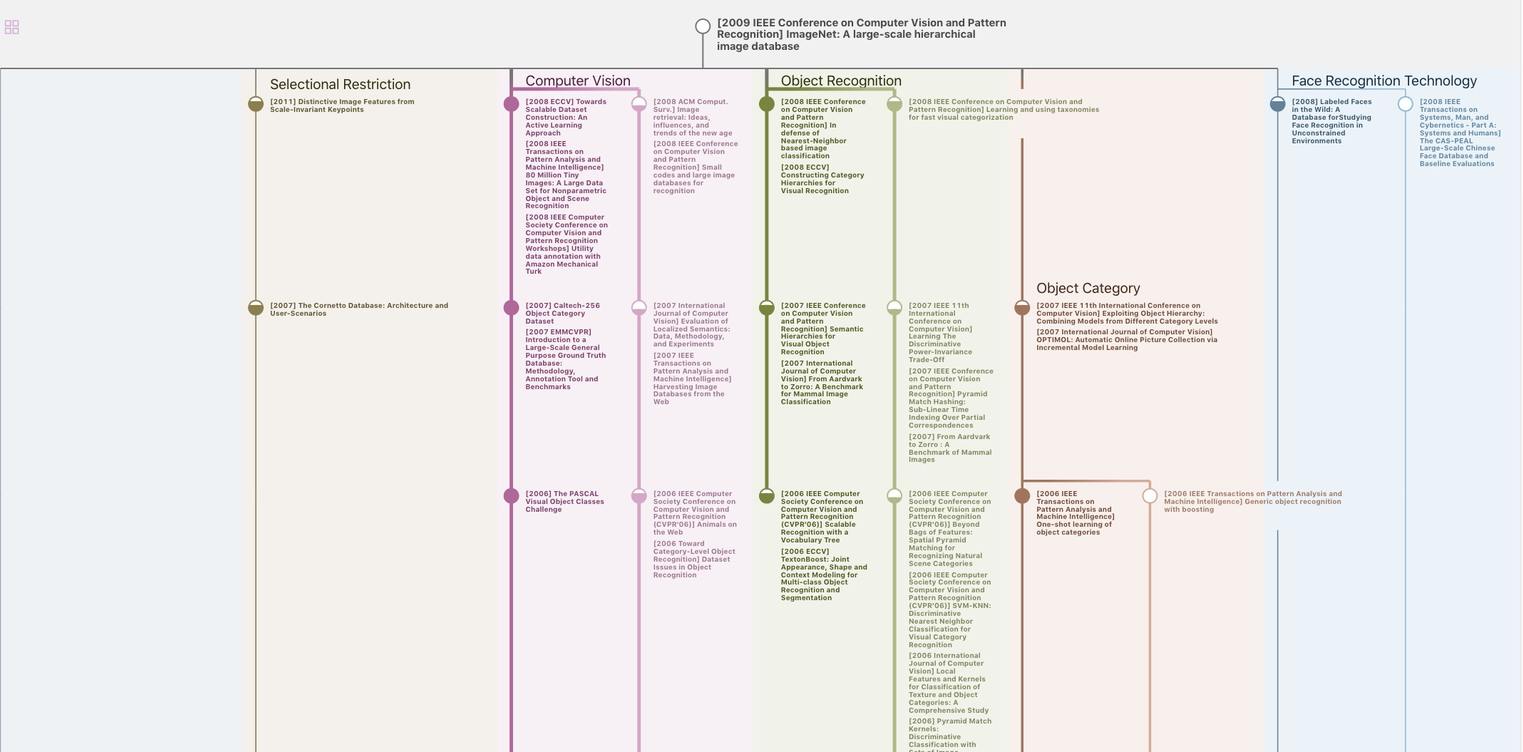
生成溯源树,研究论文发展脉络
Chat Paper
正在生成论文摘要