L R F : A logically randomized forest algorithm for classification and regression problems
Expert Systems with Applications(2023)
摘要
Tree-based ensemble algorithms (TEAs) have made significant advances in recent years due to their simple algorithmic design. However, when the proportion of the ‘most informative’ features is low, the performance of conventional TEAs degrades significantly. The primary rationale for performance degradation is that traditional algorithmic design appears to be biased toward the least informative features, and the sub-space selection procedure contains uninformative features. This paper proposes a logically randomized forest (LRF) algorithm by incorporating two different enhancements into existing TEAs. The first enhancement is made to address the issue of biasness by performing feature-level engineering. The second enhancement is the approach by which individual feature sub-spaces are selected. To derive the first enhancement, we use the graph-theoretic principle of minimal vertex cover to construct a relevant assemblage of features. Following that, the permutation-based feature importance technique is employed to calculate the ‘informativeness’ of the relevant features in order to infuse logical randomness into the individual trees in the forest. For the second enhancement, the stratified sampling method is used to ensure that the most informative features are present in all newly created feature sub-spaces. Consequently, individual trees are generated using the Roulette wheel-based selection (RWS) algorithm. The proposed algorithm has been evaluated on two real-world genomic data sets, ten hybrid-synthetic classification data sets, and twenty multidisciplinary benchmark data sets with varying characteristics. The experimental findings demonstrate that the LRF outperforms the existing benchmark and cutting-edge TEAs.
更多查看译文
关键词
forest algorithm,classification,xmlnsmml=http//wwww3org/1998/math/mathml
AI 理解论文
溯源树
样例
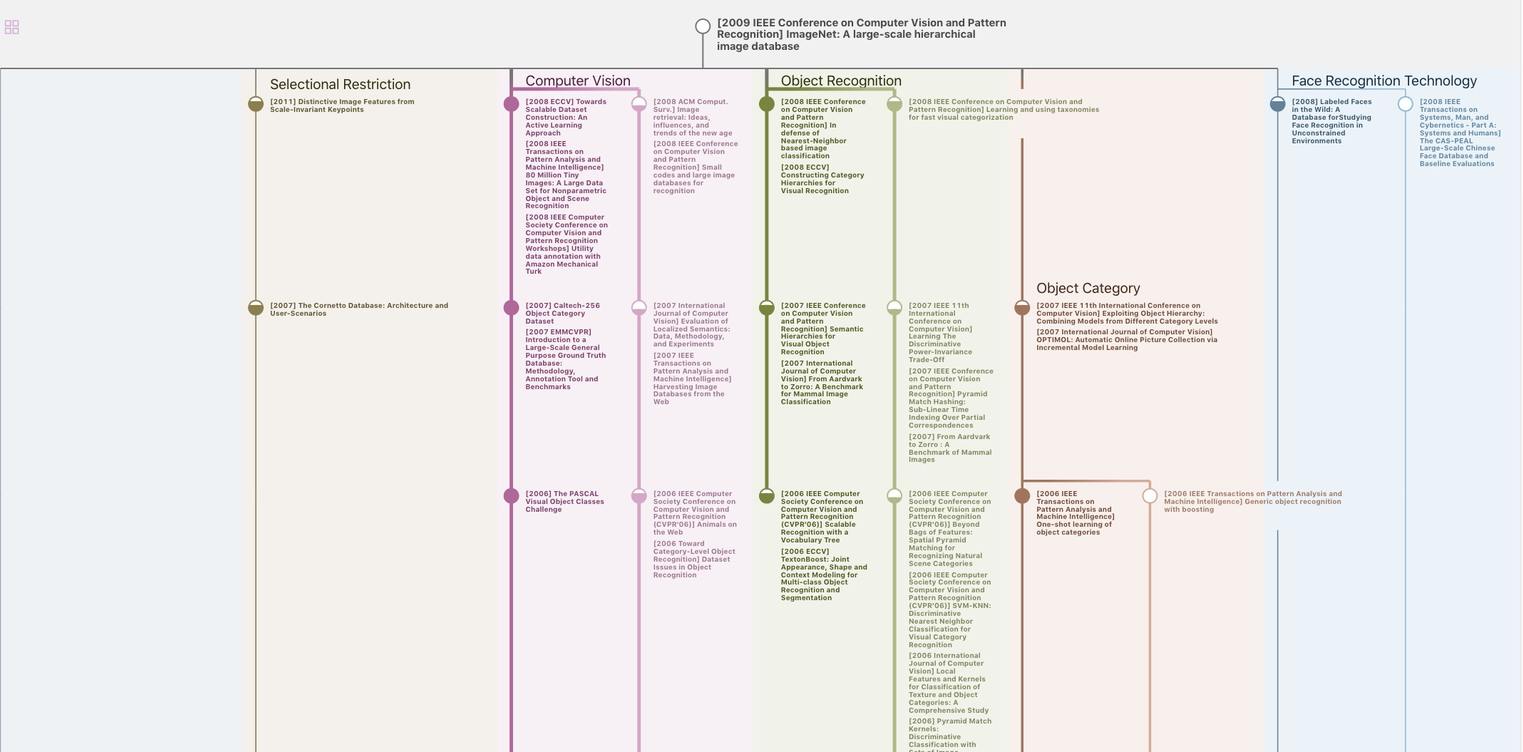
生成溯源树,研究论文发展脉络
Chat Paper
正在生成论文摘要