Combining Feature Learning and Transfer Learning in Balancing Anomaly Detection for Gas Turbine Engine Vibration Analysis
Volume 2: 42nd Computers and Information in Engineering Conference (CIE)(2022)
摘要
Abstract Rotor imbalance is a vital measure that indicates the health state of a gas turbine (GT). Abnormal balancing patterns will lead to excessive vibration and gradually compromise structural integrity. This paper presents the construction of anomaly detection (AD) models that recognize abnormal balancing patterns for two aeroderivative GTs, AGT-A and AGT-B, from Siemens Energy. Such a diagnostic tool can predict at an early stage whether a high vibration would occur during the vibration test and avoid engine reject for re-balance. Machine learning (ML) algorithms have been extensively utilized to conduct GT design space exploration and condition monitoring. However, ML has not been implemented to improve the efficiency of GT manufacturing processes, mainly due to data scarcity. The authors propose a combined feature learning and transfer learning technique to leverage the data resources of GT manufacturing processes. The physical and operational similarities between GTs belonging to the same series imply the transferability of features between models. The normal balancing patterns of the data-rich AGT-A were first learned by a sparse autoencoder to detect balancing anomalies. Then, the learned features were used to initialize the balancing AD model for the data-poor AGT-B. The test accuracy of the AGT-B AD model was increased from 75% to 92% with transfer learning. The presented methodology can facilitate and enable various data-driven analysis tasks for the manufacturing processes of original equipment manufacturers.
更多查看译文
AI 理解论文
溯源树
样例
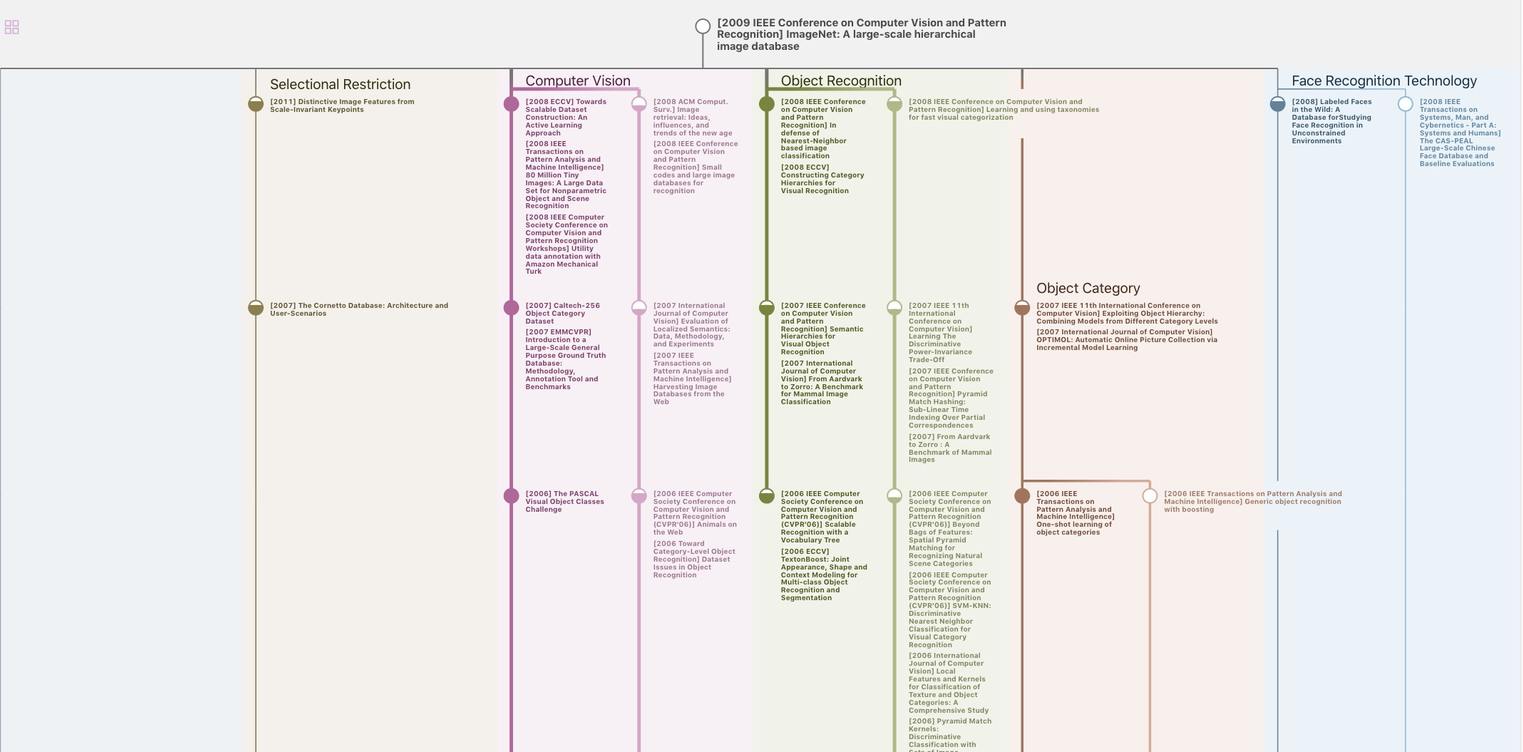
生成溯源树,研究论文发展脉络
Chat Paper
正在生成论文摘要