Deep Multiadversarial Conditional Domain Adaptation Networks for Fault Diagnostics of Industrial Equipment
IEEE Transactions on Industrial Informatics(2023)
摘要
Deep learning methods of fault diagnostics require the availability of a large amount of labeled data for training, i.e., signal values corresponding to known degradation and fault states. Furthermore, the distribution of the training data should be similar to that of the (test) data collected in the field. Since these conditions are typically not satisfied in most industrial applications, this article develops a deep multiadversarial conditional domain adaptation network. The main original contribution lies in a novel method to align, class by class, the weighted marginal data distributions using multiple domain discriminators. The network allows overtaking the classification underperformance caused by the problem of negative transfer, which is typically encountered when only few training data of some of the classes are available. The proposed method is shown to outperform other state-of-the-art methods on two cross-domain fault diagnostic case studies, verified by applying Friedman and Holm post-hoc tests.
更多查看译文
关键词
Automatic doors,bearings,conditional domain adaptation,deep transfer learning,distribution alignment,fault diagnostics,fleet of machines,multiadversarial domain adaptation,negative transfer,railway industry
AI 理解论文
溯源树
样例
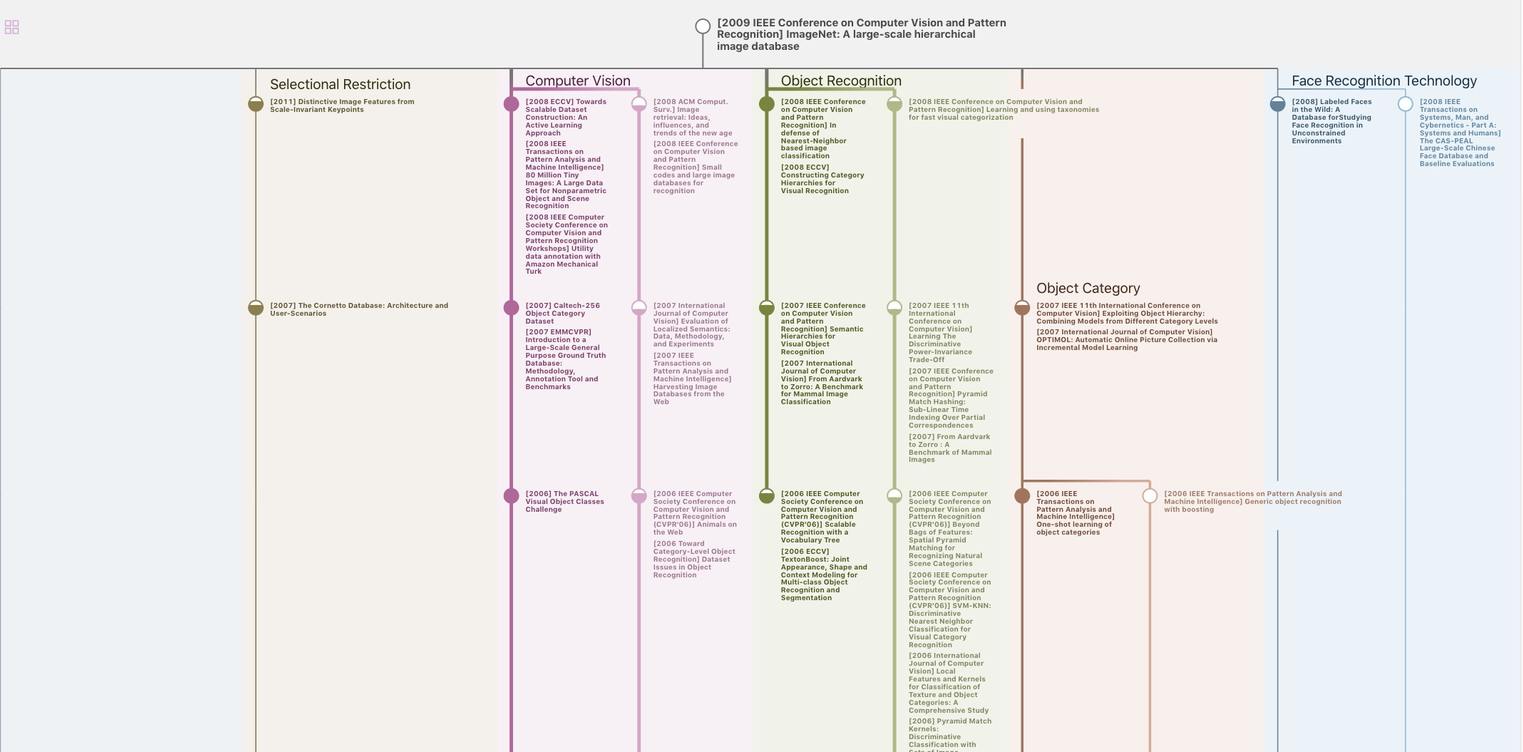
生成溯源树,研究论文发展脉络
Chat Paper
正在生成论文摘要