Multi-Level Context Fusion Network for 3D Object Tracking
crossref(2023)
摘要
Abstract Due to exhaustive proposals generation, conventional 3D object tracking methods based on template matching are time-consuming. Some recent works that leverage template clues to directly obtain 3D boxes are more efficient, but they don't take full advantage of the template context. In this work, we propose a novel multi-level context fusion network(MLCFNet) to track objects robustly . Our main idea is to fuse template context in multiple levels(point, local, and global features) into the search area and utilize the joint information to predict the final box. Specifically, a 3D Siamese Network firstly extracts multi-level features in the search area and template. Then, to promote the guidance of the template, a Context Fusion Network fuses these features into the search area and generates guided points. Finally, These points are used to regress potential object centers and cluster 3D object proposals. Experiments on KITTI and nuScenes tracking datasets demonstrate that MLCFNet outperforms other state-of-the-art methods by a large margin.
更多查看译文
AI 理解论文
溯源树
样例
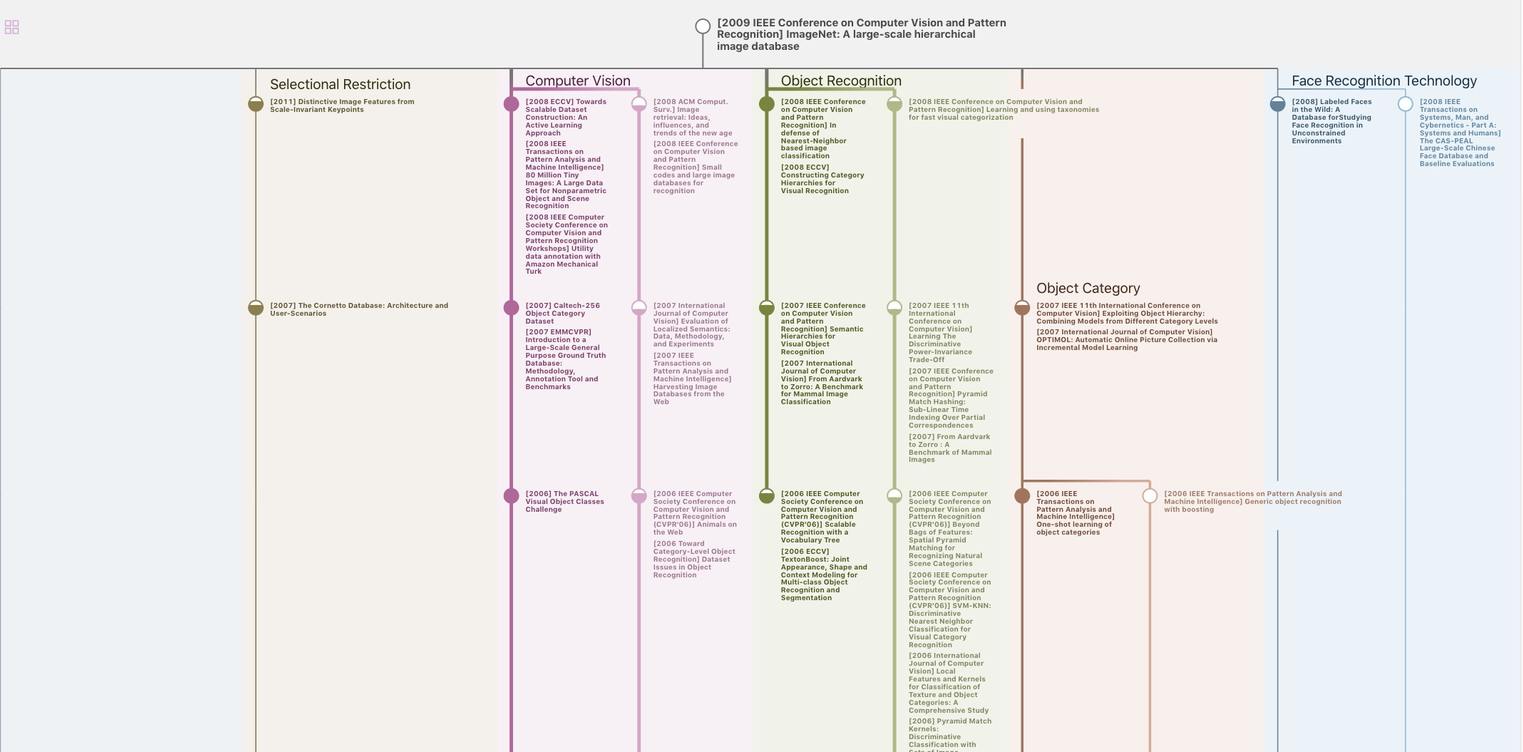
生成溯源树,研究论文发展脉络
Chat Paper
正在生成论文摘要