Scalable Kernel $k$ -Means With Randomized Sketching: From Theory to Algorithm
IEEE Transactions on Knowledge and Data Engineering(2023)
摘要
Kernel
$k$
-means is a fundamental unsupervised learning in data mining. Its computational requirements are typically at least quadratic in the number of data, which are prohibitive for large-scale scenarios. To address these issues, we propose a novel randomized sketching approach SKK based on the circulant matrix. SKK projects the kernel matrix left and right according to the proposed sketch matrices to obtain a smaller one and accelerates the matrix-matrix product by the fast Fourier transform based on the circulant matrix, which can greatly reduce the computational requirements of the approximate kernel
$k$
-means estimator with the same generalization bound as the exact kernel
$k$
-means in the statistical setting. In particular, theoretical analysis shows that taking the sketch dimension of
$\sqrt{n}$
is sufficient for SKK to achieve the optimal excess risk bound with only a fraction of computations, where
$n$
is the number of data. The extensive experiments verify our theoretical analysis, and SKK achieves the state-of-the-art performances on 12 real-world datasets. To the best of our knowledge, in randomized sketching, this is the first time that unsupervised learning makes such a significant breakthrough.
更多查看译文
关键词
randomized sketching
AI 理解论文
溯源树
样例
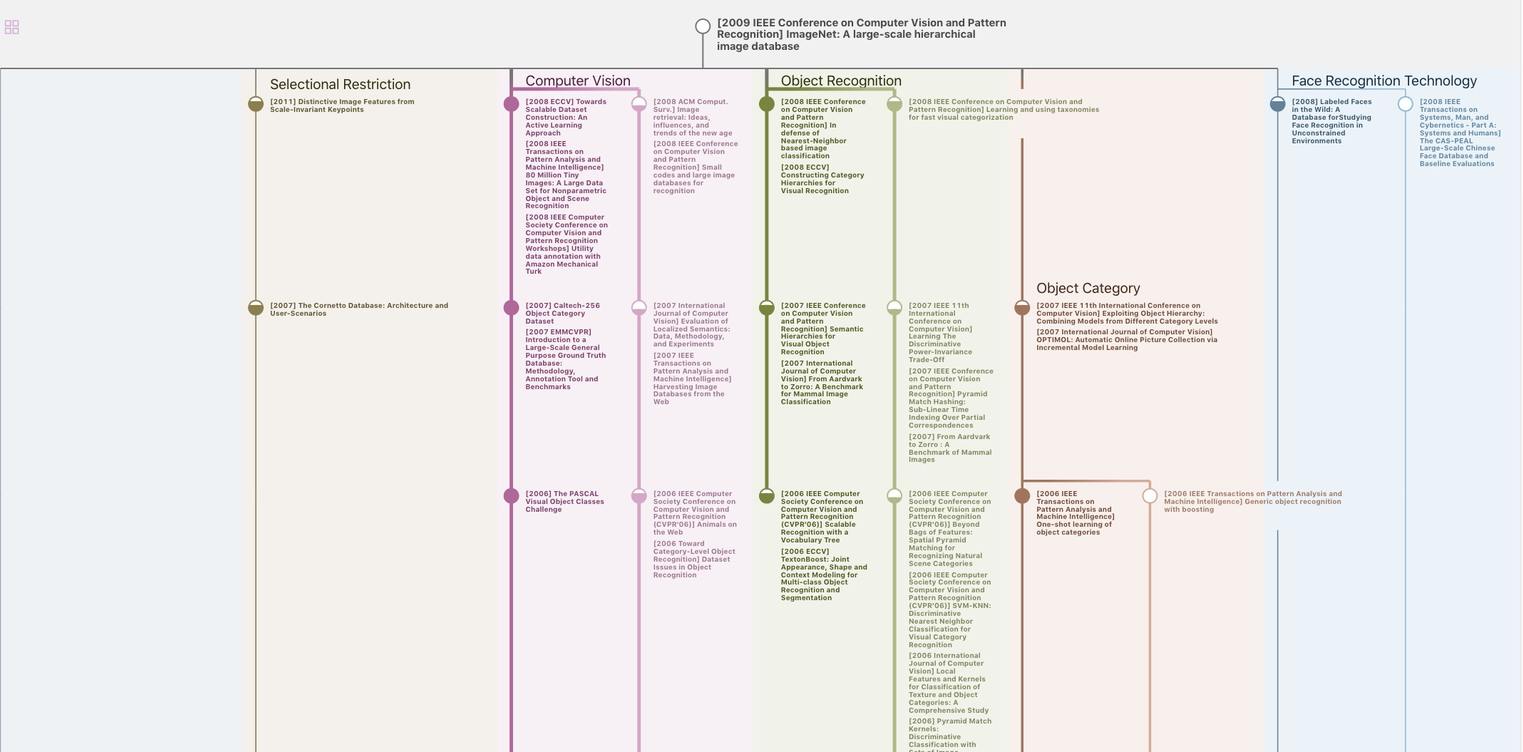
生成溯源树,研究论文发展脉络
Chat Paper
正在生成论文摘要