Deep reinforcement learning based trajectory design and resource allocation for task-aware multi-UAV enabled MEC networks
COMPUTER COMMUNICATIONS(2024)
摘要
Computing tasks in the air is an important form of mobile edge computing (MEC) to improve the quality of service and enhance network coverage. In this paper, we investigate a multi-UAV cooperative computing model and massive devices access scenario in a service area without infrastructure. There are various types of ground devices with different tasks. Moreover, we consider that the UAV executing tasks of devices need to cache the content that task required. Therefore, we propose a multi-UAV enabled MEC network based on task awareness where each UAV caches some programs to execute tasks offloaded from devices. To minimize completion time, a joint UAV trajectory design, access decision and resource allocation problem is formulated. To address this intractable mixed integer non-linear programming problem, a multi-agent trajectory design and resource allocation (MATR) algorithm is proposed, where the multi-agent deep deterministic policy gradient (MADDPG) is applied. Considering the complexity of high-dimensional continuous action space, we introduce the particle swarm optimization (PSO) algorithm to jointly optimize access decisions, and computation resource allocation to reduce action space. In addition, we discuss the impact of the size of UAV cache space and the location of ground devices on the completion time. Simulation results show that the MATR algorithm can significantly reduce the completion time compared to the baselines.
更多查看译文
关键词
UAV,Cooperative computing,Deep reinforcement learning,Trajectory design,Resource allocation
AI 理解论文
溯源树
样例
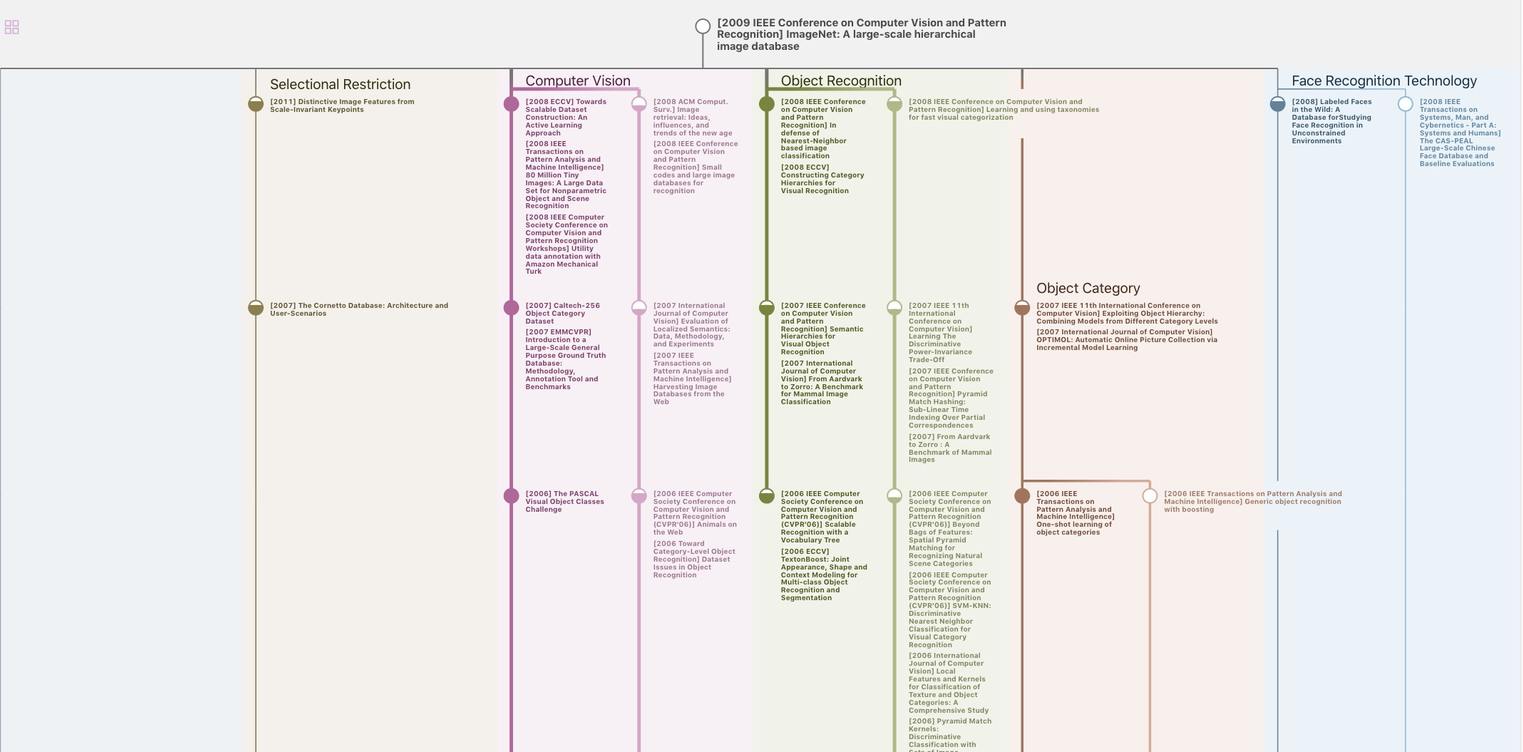
生成溯源树,研究论文发展脉络
Chat Paper
正在生成论文摘要