Raw channel data-based phase aberration correction for ultrasound localization microscopy using conditional generative adversarial networks
The Journal of the Acoustical Society of America(2022)
摘要
Phase aberration (PA) significantly deteriorates imaging quality for transcranial imaging because of large speed of sound discrepancy and complex geometry of the skull. Recently, ultrasound localization microscopy (ULM) has demonstrated promising transcranial imaging results of deep brain microvasculature through intact skull. Robust PA correction is essential for high-fidelity transcranial ULM imaging because microbubble localization is highly susceptible to PA. Most existing PA correction methods rely on “guide stars,” which may not be practical for ULM because the microbubble concentration may be too high to serve as guide stars. Furthermore, most conventional PA correction techniques assume globally or locally homogeneous delay law, thus resulting in suboptimal correction performance. To address these issues, we propose a deep learning-based PA correction approach that directly operates on the pre-beamformed, raw channel data. By taking advantage of the structured microbubble signals and using conditional generative adversarial networks to utilize specific aberrated channel data, our proposed method achieved pixel-wise PA correction. In silico studies using micro-CT scanned mouse skulls demonstrate that the lateral beam width at −20 dB is improved from 0.82 ±0.33 mm to 0.48 ± 0.12 mm at 20 MHz applying proposed method, and the mean localization error is reduced from 67.99 ± 21.50 μm to 21.45 ± 14.84 μm.
更多查看译文
关键词
ultrasound localization microscopy,conditional generative adversarial networks,phase aberration correction,data-based
AI 理解论文
溯源树
样例
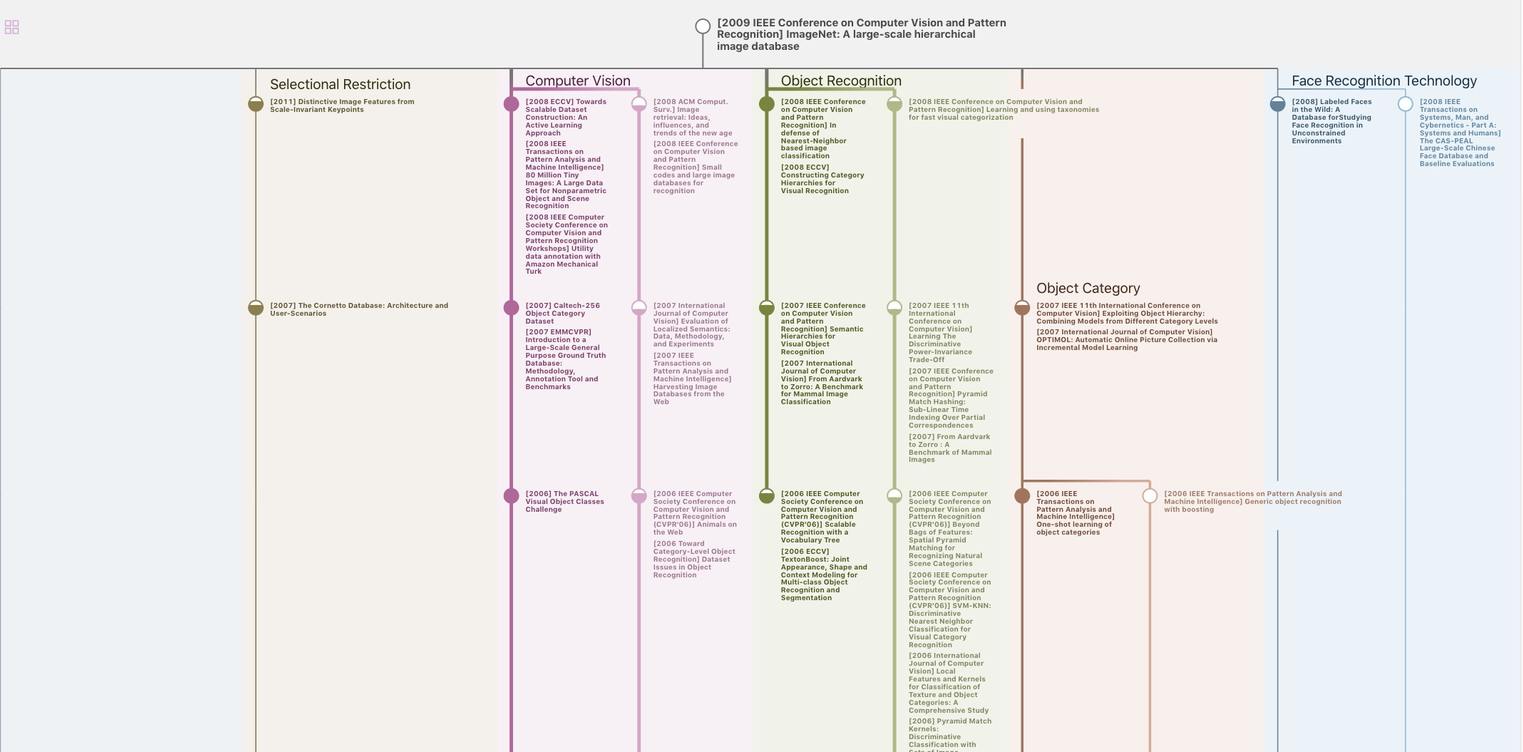
生成溯源树,研究论文发展脉络
Chat Paper
正在生成论文摘要