Using Ensemble Convolutional Neural Network to Detect Deepfakes Using Periocular Data
crossref(2023)
摘要
Deepfakes are manipulated or altered images, or video, that are created using deep learning models with high levels of photorealism. The two popular methods of producing a deepfake are based on either convolutional neural networks (CNN), or autoencoders. Deepfakes created using CNN comparatively show higher qualities of realism, yet oftentimes leave artifacts and distortions in the generated media that can be detected using machine learning and deep learning algorithms. In recent years, there has been an influx of periocular image and video data because of the increase usage of face masks. By wearing masks, much of what is used for facial recognition is hidden, leaving only the periocular region visible to an observer. This loss of vital information leads to easier misidentification of media, allowing deepfakes to less likely be identified as fake. In this work, feature extraction methods, such as Scale-Invariant Feature Transform (SIFT), Histogram of Oriented Gradients (HOG), and CNN, are used to train an ensemble deep learning model to detect deepfakes in videos on a frame-by-frame level based on the periocular region. Our proposed model is able to distinguish original and manipulated images with accuracies around 98.9 percent, which is an improvement to previous works by combining SIFT and HOG for deepfake detection in convolutional neural networks.
更多查看译文
AI 理解论文
溯源树
样例
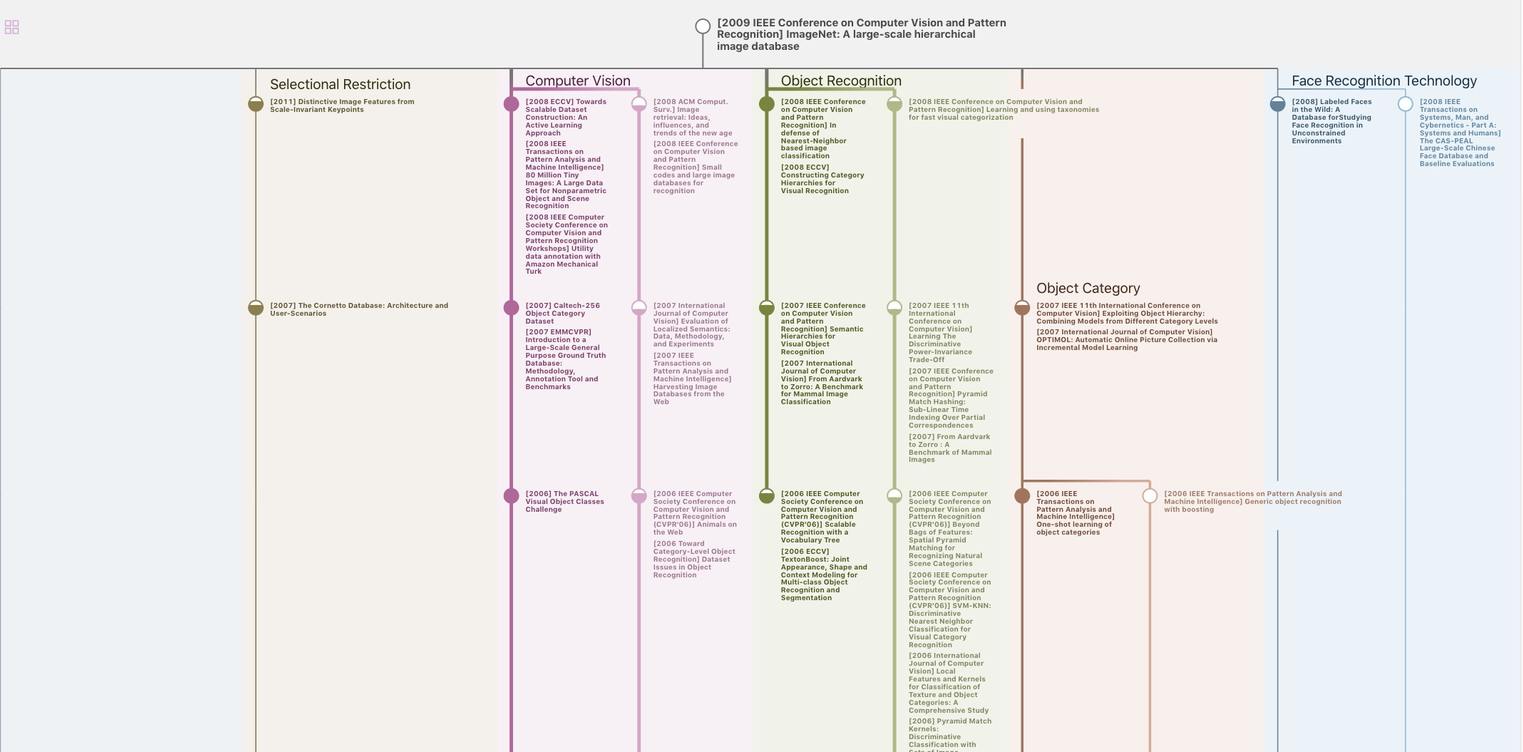
生成溯源树,研究论文发展脉络
Chat Paper
正在生成论文摘要