Joint ocean physics-acoustics inversion and mutual-information based experiment design in realistic ocean fields
The Journal of the Acoustical Society of America(2022)
摘要
Reliable acoustic exploration and navigation in the ocean requires precise knowledge of the environmental fields (e.g. ocean physics, bathymetry, seabed) and acoustic parameters (e.g. source location and frequencies). However, such knowledge is typically incomplete due to the sparse and heterogeneous observations, and to the complex high-dimensional multiscale dynamics. In this work, we use our stochastic Dynamically Orthogonal Parabolic Equation (DO-ParEq) framework to: (i) model the ocean environment uncertainties computed from a large realistic ocean ensemble forecast, and (ii) predict the resulting stochastic acoustic fields and their probability distributions. We then employ new nonlinear Bayesian learning algorithms that use Gaussian Mixture Models to assimilate ocean-acoustic measurements and jointly infer the unknown properties of the ocean environment, the bathymetry, the acoustic field and parameters, and even the model parameterizations themselves. We showcase the developed techniques in realistic data-assimilative sea experiments in the New York Bight Region and the Mediterranean Sea. Finally, we demonstrate the impact of selecting measurement locations using a mutual-information-based algorithm that maximizes the information content in the learned fields based on the measurement constraints.
更多查看译文
关键词
realistic ocean fields,inversion,physics-acoustics,mutual-information
AI 理解论文
溯源树
样例
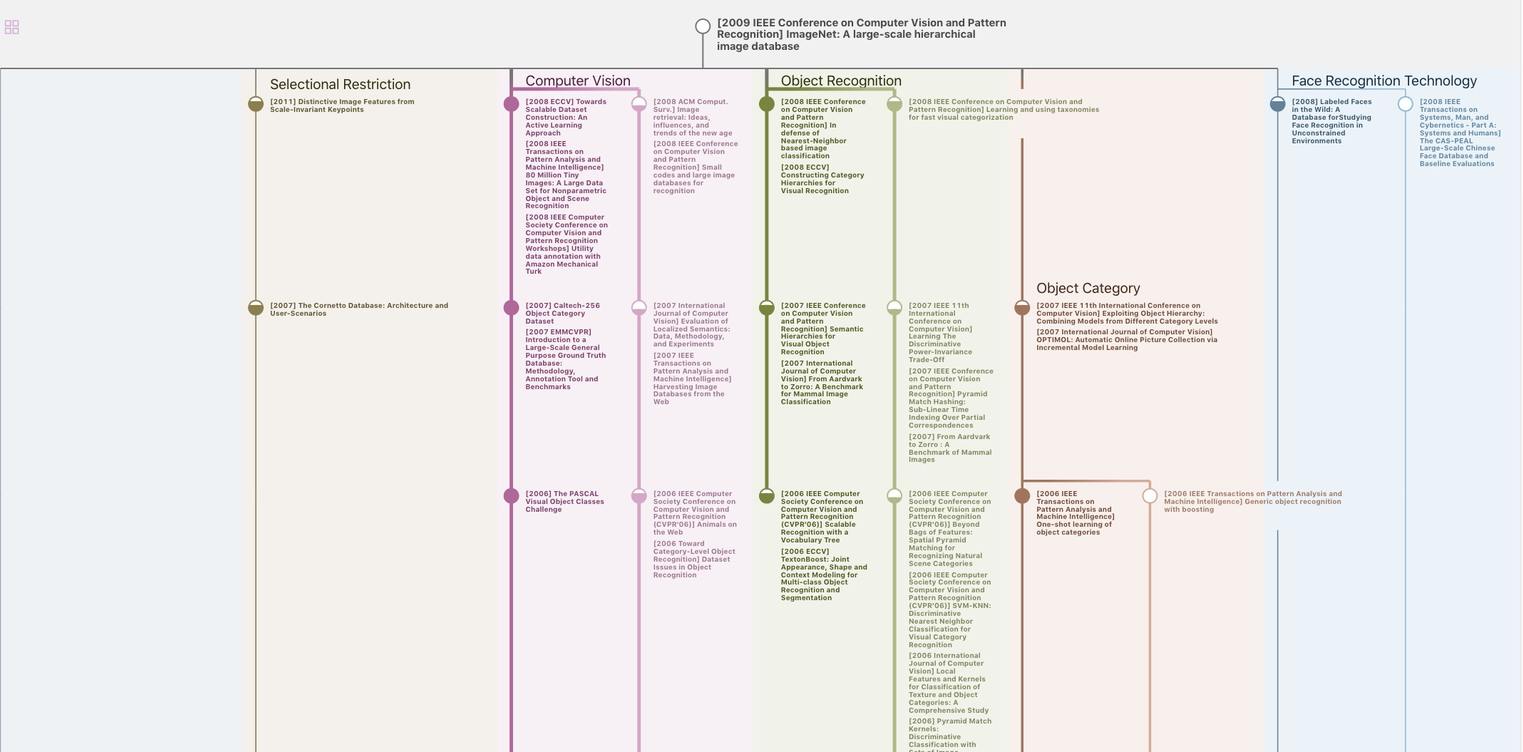
生成溯源树,研究论文发展脉络
Chat Paper
正在生成论文摘要