Dimension Reduction Applied to Laboratory Earthquake Acoustic Emissions for Visual Analysis and Machine Learning
crossref(2023)
摘要
<p>It has previously been shown that features calculated using rolling windows of acoustic emissions (AE) emitted from laboratory shear experiments give a robust basis to predict shear stress and time to failure (TTF) using machine learning (ML). Early studies used ensemble tree methods like random forests and gradient boosted trees for learning and inference. The upside of these methods is that they allow for relatively easy analysis of the behaviour of learned models. It is possible to go through every decision inside the trees to evaluate why the model made a certain prediction, based on the features fed into the model. These predictions, however, are only based on local time-window information. The recent AE inside the time window hold no information of the temporal evolution of the features. More recently, progress has been made in improving the quality of predictions by making use of for example Deep Neural Networks (DNN’s) containing techniques like long short-term memory (LSTM) which have the advantage of learning from temporal evolutions. These more advanced ML techniques act however, more like a black-box model and as a result it is more complicated, to nearly impossible, to explain why a certain prediction is made. Here dimension reduction techniques like Principal Component Analysis (PCA) and Uniform Manifold Approximation and Projection (UMAP) are used to visualize the temporal evolution of features. The embeddings of features can both be used for visual inspection and as the input for ML models. The embedded features are fed to ensemble learning methods like a random forest as additional input to predict shear stress and TTF. This technique provides the relatively simple ML method with temporal information, while still enabling the interpretation of predictions made by the model. This approach is applied to both earlier studied data of the double-direct shear apparatus located at the Pennsylvania State University and new data obtained by experiments in the Rotary Shear Apparatus located at the Utrecht University High Pressure and Temperature Laboratory. Visual inspection of the embedded features shows that different shear stress measurements map to distinct patterns, meaning that it is not only the local time windows of the feature that hold predictive information, but also their global temporal evolution, and that the patterns repeat for multiple laboratory earthquake cycles. Feeding the embedded features of variance and kurtosis (the features previously shown to be most influential on the final prediction) into a ML model additionally to the traditional time windows results in highly improved predictions (R2-scores in the order of 0.9), nearly as good as the state-of-the-art LSTM models. From this it can be concluded that the temporal evolution of features must be considered, because it holds additional information critical to making the best predictions possible. Besides improving the predictions, the embedded features also provide a quantitative data-driven framework to study the temporal evolution of features.</p>
更多查看译文
AI 理解论文
溯源树
样例
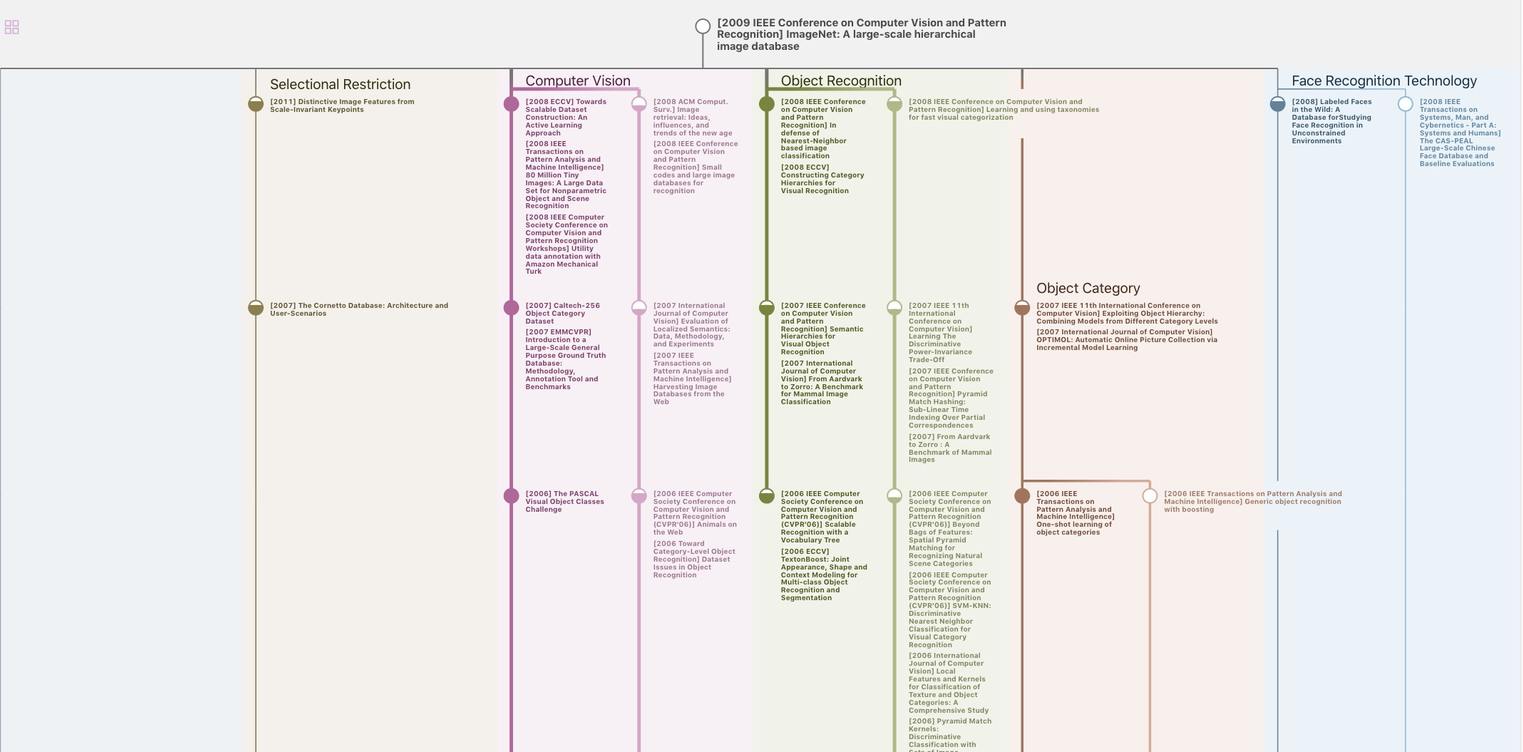
生成溯源树,研究论文发展脉络
Chat Paper
正在生成论文摘要