Remote Sensing Image Change Detection Network Based on Twin High-Resolution Representation
Mobile Information Systems(2023)
摘要
With the increase of spatial resolution of remote sensing images, features of feature imaging become more and more complex, and the change detection methods based on techniques such as texture representation and local semantics are difficult to meet the demand. Most change detection methods usually focus on extracting semantic features and ignore the importance of high-resolution shallow information and fine-grained features, which often lead to uncertainty in edge detection and small target detection. For single-input networks when two temporal images are connected, the shallow layer of the network cannot provide the information of the individual original image to the deep layer features to help reconstruct the image, and therefore, the change detection results may be missing in detail and feature compactness. For this purpose, a twins context aggregation network (TCANet) is proposed to perform change detection on remote sensing images. In order to reduce the loss of spatial accuracy of remote sensing images and maintain high-resolution representation, we introduce HRNet as our backbone network to initially extract the features of interest. Our proposed context aggregation module (CAM) can amplify the convolutional neural network receptive field to obtain more detailed contextual information without significantly increasing the computational effort. The side output embedding module (SOEM) is proposed to improve the accuracy of small volume target change detection as well as to shorten the training process and speed up the detection while ensuring the performance. The method has experimented on the publicly available CDD dataset, the SYSU-CD dataset, and a challenging DSIFN dataset. With significant improvements in precision, recall, F1 score, and overall accuracy, the method outperforms the five methods mentioned in the literature.
更多查看译文
关键词
remote sensing,high-resolution high-resolution,detection
AI 理解论文
溯源树
样例
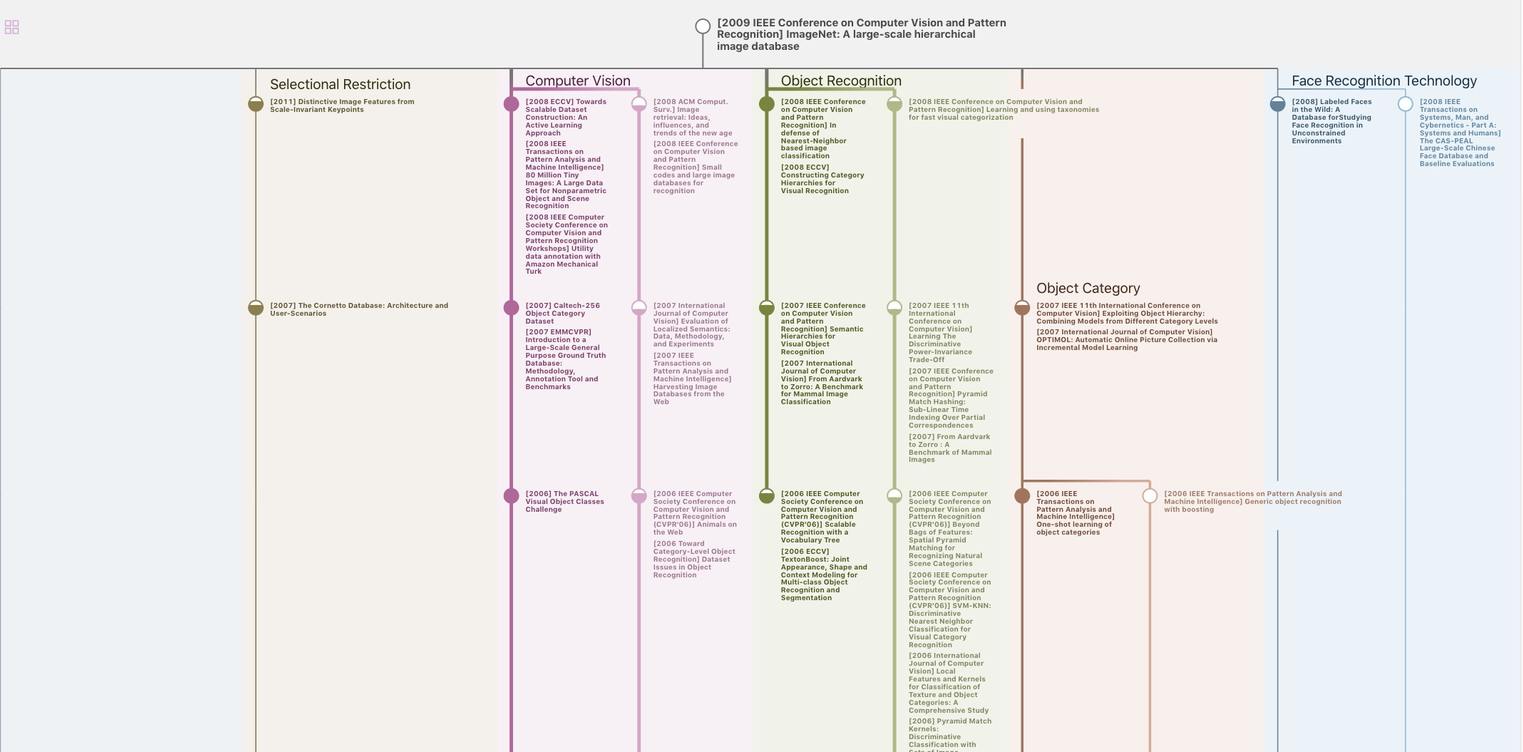
生成溯源树,研究论文发展脉络
Chat Paper
正在生成论文摘要