Curation of High-level Molecular Atmospheric Data for Machine Learning Purposes
crossref(2023)
摘要
<div> <div> <p><span data-contrast="auto">As cloud and aerosol interactions remain large uncertainties in current climate models (IPCC) they are of special interest for atmospheric science. It is estimated that more than 70% of all cloud condensation nuclei origin from so-called New Particle Formation, which is the process of gaseous precursors clustering together in the atmosphere and subsequent growth into particles and aerosols. After initial clustering this growth is driven strongly by condensation of low volatile organic compounds (LVOC), that is molecules with saturation vapor pressures (</span><span data-contrast="auto">p<sub>Sat</sub>) below 10</span><sup><span data-contrast="auto">-6</span></sup><span data-contrast="auto"> mbar [1]. These origin from organic molecules emitted by vegetation that are subsequently rapidly oxidized in the air, so-called Biogenic LVOC (BLVOC).</span></p> </div> <div> <p><span data-contrast="auto">We have created a big data set of BLVOC using <em>high-throughput computing</em> and <em>Density Functional Theory</em> (DFT), and use it to train M</span><span data-contrast="auto">achine Learning models to predict p<sub>Sat</sub> of previously unseen BLVOC.</span><span data-ccp-props="{"> Figure 1 illustrates some sample molecules form the data.<br /></span></p> <p><span data-ccp-props="{"><img src="" alt="" width="386" height="386" /></span><span data-ccp-props="{"><img src="" alt="" /></span></p> </div> <div> <p><span data-contrast="auto">Figure 1: Sample molecules, for small, medium large sizes.     Figure 2: Histogram of the calculated saturation vapor pressures.</span></p> <p><span data-contrast="auto">Initially the chemical mechanism GECKO-A provides possible BLVOC molecules in the form of SMILES strings. In a first step the COSMOconf program finds and optimizes structures of possible conformers and provides their energies for the liquid phase on a DFT level of theory. After an additional calculation of the gas phase energies with Turbomole, COSMOtherm calculates thermodynamical properties, such as the </span><span data-contrast="auto">p<sub>Sat</sub>, using the COSMO-RS [1] model. We compressed all these computations together in a highly parallelised high-throughput workflow to calculate <strong>32k</strong> BLVOC, that include over <strong>7 Mio.</strong> molecular conformers. See a histogram of the calculated p<sub>Sat </sub>in Figure 2.</span><span data-ccp-props="{"><br /></span></p> </div> <div> <p><span data-contrast="auto">We use the calculated p</span><span data-contrast="auto">Sat</span><span data-contrast="auto"> to train a <em>Gaussian Process Regression</em> (GPR) machine learning model with the <em>Topological Fingerprint</em> as descriptor for molecular structures.</span><span data-ccp-props="{"> The GPR incorporates noise and outputs uncertainties for predictions on the p<sub>Sat</sub>. T</span><span data-contrast="auto">hese uncertainties and data cluster techniques allow for the active choosing of molecules to include in the training data,<em> </em>so-called <em>Active Learning. </em>Further, we explore using <em><span class="u-small-caps">SLISEMAP</span></em> [2] explainable AI methods to correlate Machine Learning predictions, the high-dimensional descriptors and human-readable properties, such as functional groups.<em> </em></span></p> <p><span data-contrast="auto"><span dir="ltr" role="presentation">[1] Metzger, A. et al. Evidence for the role of organics in aerosol particle formation under atmospheric conditions. <em>Proc. Natl. Acad. Sci.</em> 107, 6646–6651, 10.1073/pnas.0911330107 (2010)<br />[2] Klamt, A. & Schüürmann, G. Cosmo: a new approach to dielectric screening in solvents with explicit expressions for the </span><span dir="ltr" role="presentation">screening energy and its gradient.</span><em> </em><span dir="ltr" role="presentation"><em>J. Chem. Soc.</em>, Perkin Trans. 2</span> <span dir="ltr" role="presentation">799–805, 10.1039/P29930000799 (1993).<br />[3] Björklund, A., Mäkelä, J. & Puolamäki, K. <span class="u-small-caps">SLISEMAP</span>: supervised dimensionality reduction through local explanations. <em>Mach Learn</em> (2022). https://doi.org/10.1007/s10994-022-06261-1<br /></span></span></p> </div> </div>
更多查看译文
AI 理解论文
溯源树
样例
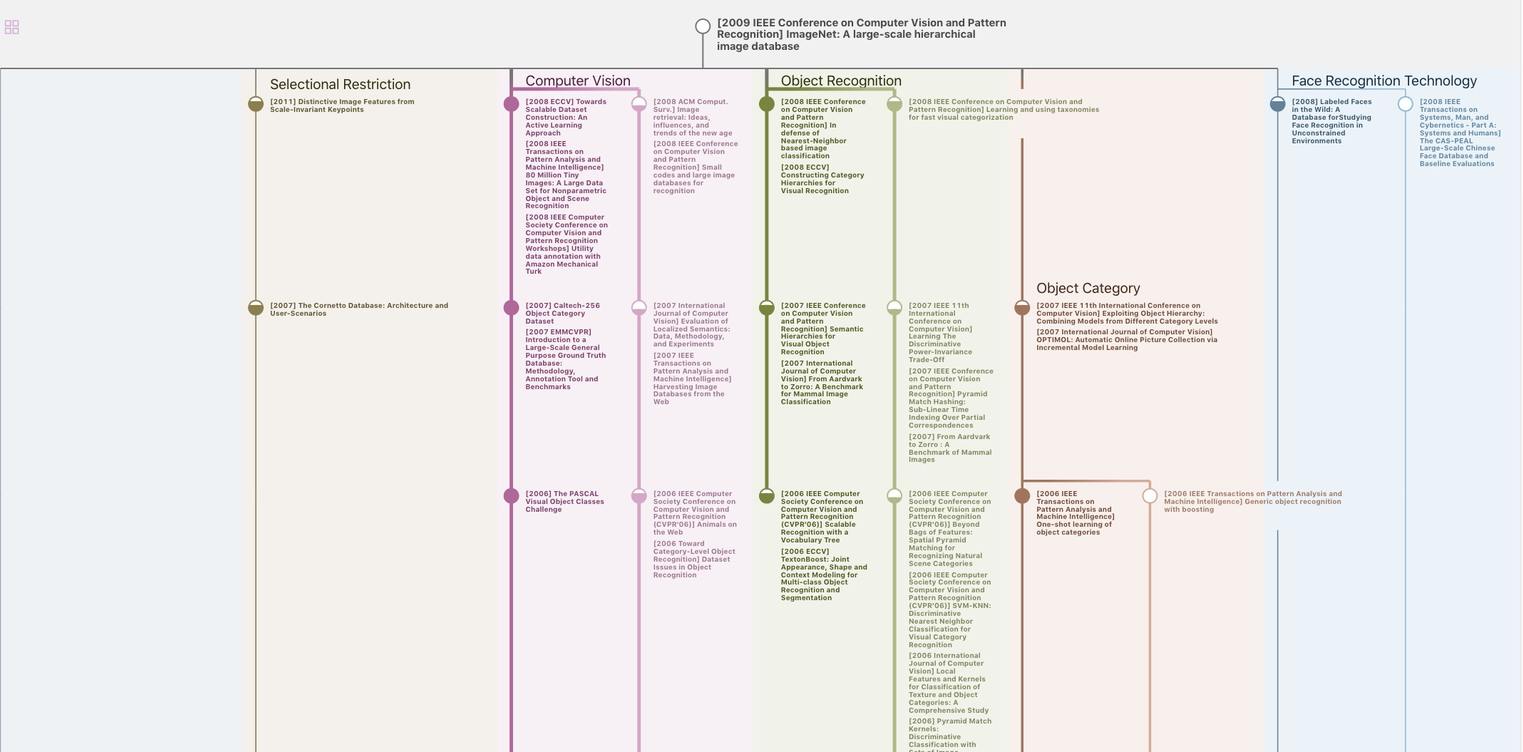
生成溯源树,研究论文发展脉络
Chat Paper
正在生成论文摘要