Machine learning detection of dust impact signals observed by the Solar Orbiter
crossref(2023)
摘要
<p>At present, ongoing space missions (PSP, SolO) provide the opportunity to closely observe the dust distribution in the inner solar system. It is however challenging to automatically detect and separate dust impact signals from other observed features for two main reasons. Firstly, since the spacecraft charging causes variable shapes of the dust signals, and secondly because electromagnetic waves (such as solitary waves) may induce resembling electric field signals.</p> <p>In this presentation, we propose a novel method, based on artificial intelligence, for detecting dust impacts in Solar Orbiter observations with high accuracy. Two supervised machine learning approaches are considered: the support vector machine (SVM) classifier and the convolutional neural network (CNN) classifier. Furthermore, we compare the performance of the machine learning classifiers to the currently used on-board classification algorithm and analyze 2 years of Solar Orbiter data.</p> <p>Overall, we conclude that detection of dust signals is a suitable task for machine learning techniques. The convolutional neural network achieves the highest performance with 96% ± 1% overall classification accuracy and 94% ± 2% dust detection precision, a significant improvement to the currently used on-board classifier with 85% overall classification accuracy and 75% dust detection precision. In addition, both the support vector machine and the convolutional neural network detect more dust particles (on average) than the on-board classification algorithm, with 16% ± 1% and 18% ± 8% detection enhancement respectively.</p>
更多查看译文
AI 理解论文
溯源树
样例
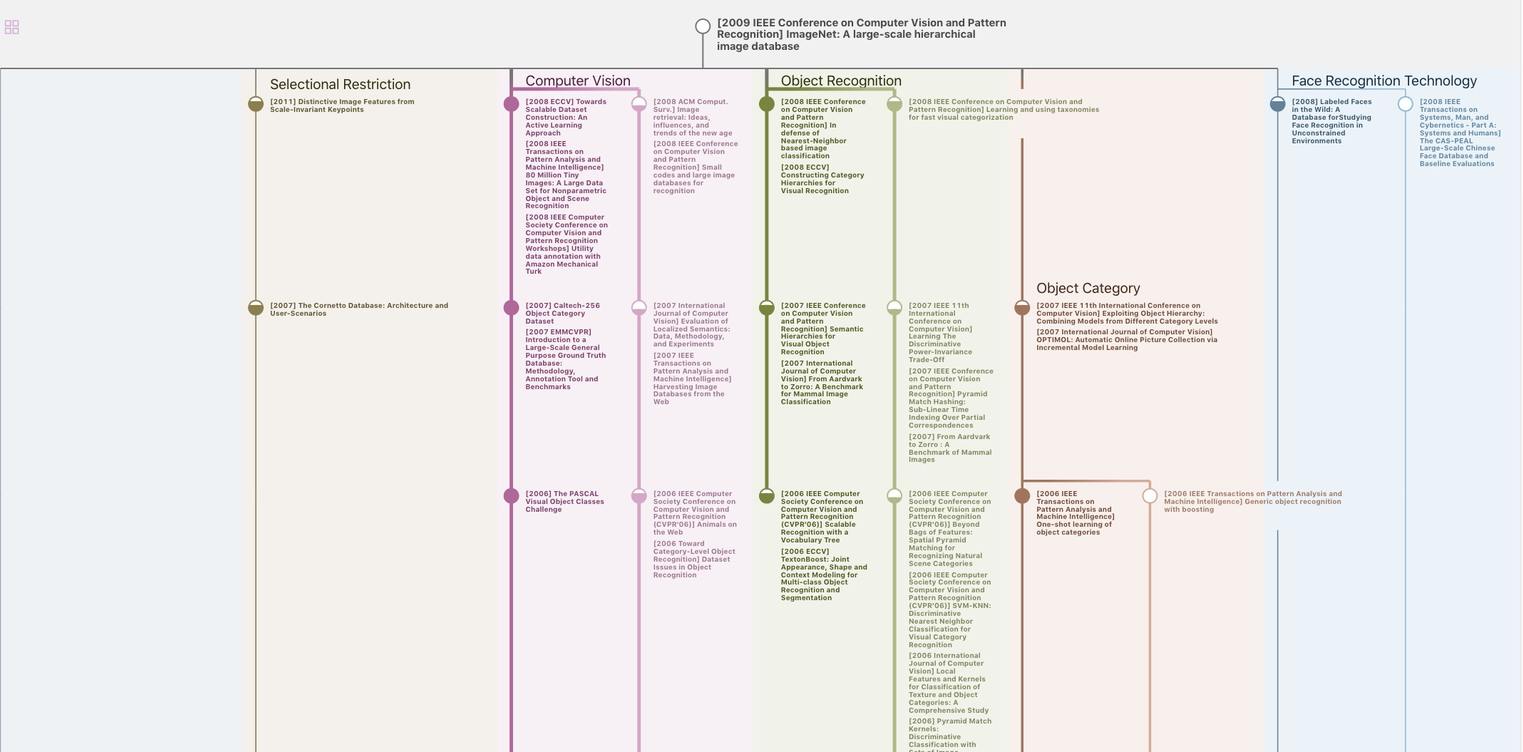
生成溯源树,研究论文发展脉络
Chat Paper
正在生成论文摘要