Machine learning investigation of gene expression datasets revealsTP53mutant-like AML with wild typeTP53and poor prognosis
bioRxiv (Cold Spring Harbor Laboratory)(2023)
摘要
AbstractAcute myeloid leukemia (AML) withTP53mutations (TP53Mut) has poor clinical outcomes with 1-year survival rates of less than 10%. We investigated whether this AML subtype harbors a distinct gene expression profiling (GEP), what this GEP reveals aboutTP53MutAML pathophysiology, and whether this GEP is prognostic inTP53wild type (TP53WT) AML.We applied a supervised machine-learning approach to assess whether a uniqueTP53MutGEP could be detected. Using the BEAT-AML dataset, we randomly divided the samples into training and testing datasets, while the TCGA dataset was reserved as a validation dataset. We trained a ridge regression machine learning model to classifyTP53MutandTP53WTcases. This model was highly accurate in distinguishingTP53MutversusTP53WTcases in both the test and validation data sets. Additionally, we noted a cohort ofTP53WTsamples with high ridge regression scores and poor overall survival, suggesting share clinical and GEP features withTP53MutAML. We defined theseTP53WTsamples asTP53mutant-like (TP53Mut-like) AMLs. We trained a second ridge regression model to specifically detectTP53Mut-like samples in the BEAT AML dataset and found that TCGA data also harborsTP53Mut-like samples. TheTP53Mut-like samples in the TCGA also have a worse OS rate thanTP53WTcases. Using drug sensitivity data from 122 small molecules in the BEAT AML dataset, we foundTP53Mut-like AMLs have distinct drug sensitivity patterns compared toTP53WT. Finally, we identified a 25 gene signature that can identifyTP53Mut-like cases. This signature could be used clinically to identify this novel subset of poor-prognosis AML.
更多查看译文
关键词
gene expression datasets,gene expression,machine learning,mutant-like
AI 理解论文
溯源树
样例
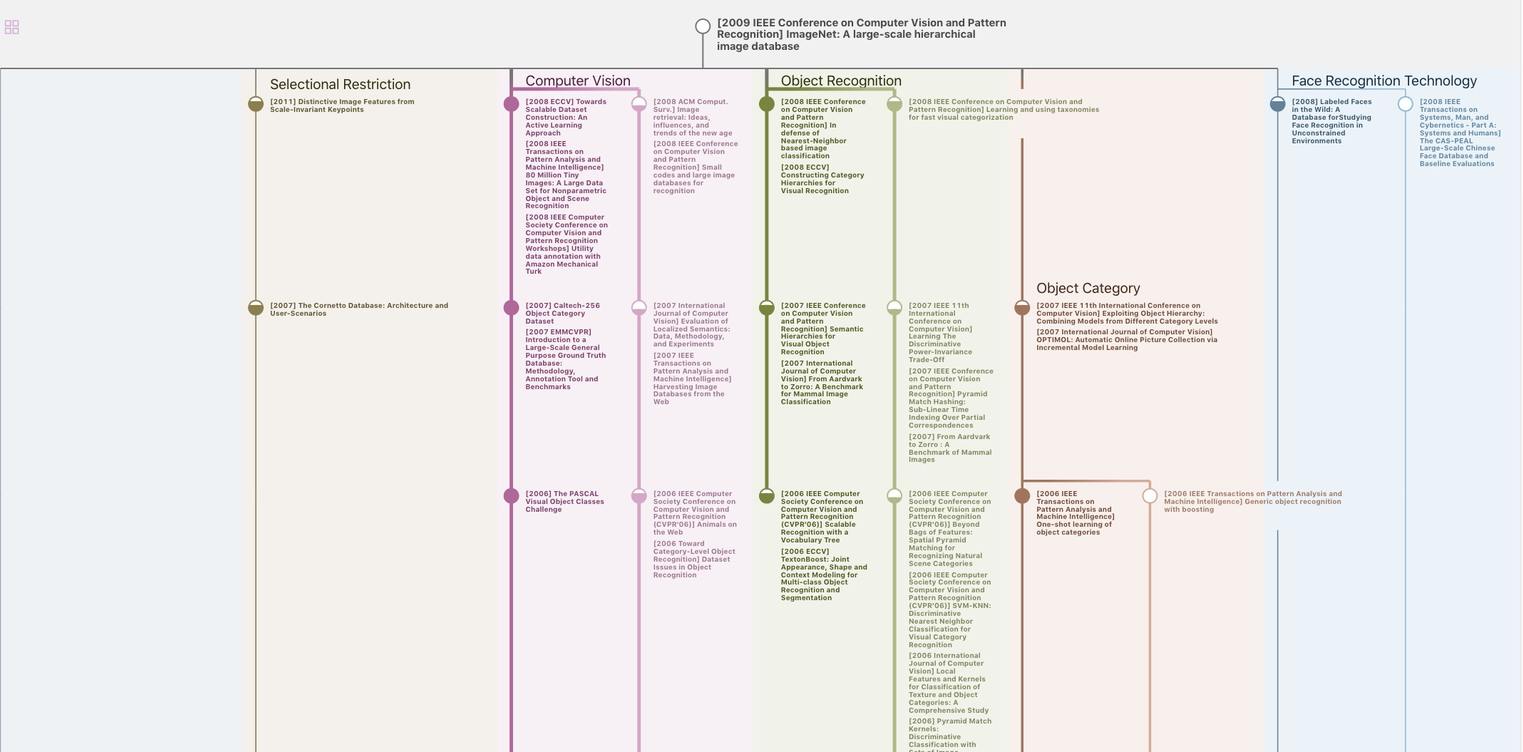
生成溯源树,研究论文发展脉络
Chat Paper
正在生成论文摘要