Identification of Genetically Closely Related Peanut Varieties Using Deep Learning: The Case of Flower-Related Varieties 11
Proceedings of the International Conference on Paradigms of Computing, Communication and Data SciencesAlgorithms for Intelligent Systems(2023)
摘要
Senegal's groundnut seed needs are estimated at 10,000 tons per year, but only 30% of this is provided cited in WAAPP ( http://www.waapp-ppaao.org/senegal/index.php/actualit%C3%A9s/240-seuls-30-des-besoins-en-semences-d%E2%80%99arachide-assur%C3%A9s-expert.html ). Faced with a worrying national seed situation combined with the problem of climate change, the Senegalese government, in its regional agricultural development strategy, recommends the production of selected seeds (good varietal purity and good seed value), which is essential for the revival of quality production. Thus, within the framework of the West African Agricultural Productivity Program (WAAPP), the Senegalese Agricultural Research Institute (ISRA) has just released new groundnut varieties from flower 11 quoted in TALKAG ( https://www.talkag.com/blogafrique/?p ). These varieties were obtained by the National Center for Agricultural Research (CNRA) of Bambey WAAPP ( http://www.waapp-ppaao.org/senegal/index.php/actualit%C3%A9s/240-seuls-30-des-besoins-en-semences-d%E2%80%99arachide-assur%C3%A9s-expert.html ). Adapted to dry areas (300–500 mm), they are designed to respond to the problem of climate change and are characterized by yields that are three times higher than the old seeds cultivated until now in Senegal. However, we note a problem in identifying these groundnut varieties with the naked eye, given that they are genetically very similar. The means of identification currently used are too limited in terms of taking into account all the identification characteristics. In this paper, we use the deep learning approach of the VGG16 model, a convolutional neural network model, to efficiently and reliably identify genetically closely related peanut varieties from the same parent variety, Flower 11. For this, the VGG16 model is used on a dataset of 06 varieties (“Jambar”, “Tosset”, “Raw Gadu”, “Taaru”, “Kom Kom”, “Yaakar”). The aim of this paper is to propose a Deep Learning model capable of identifying the distinctive characteristics of a shell or seed image and to calculate the probability of similarity of the image to each of these varieties mentioned above. Therefore, the model is based on a categorical classification and will have six (06) types of answers. Thus we have intentionally taken in a first step a small dataset (1102 images) chosen so that the model is not optimal and then we will use the technique of data augmentation to improve and make our model more efficient. Based on the distinctive parameters of the seeds and pods, we first trained our model which ran from start to finish taking into account all the features. Afterwards, we applied data augmentation to train the model again. After the data augmentation, the test results are satisfactory and can be differentiated according to the image of the tested variety. The performance evaluation of the model shows an accuracy rate of 0.72 and a loss rate of 0.54. For the test results, we tested an image “kom kom” with an accuracy of 60% for the variety “kom kom” which is the variety passed as a parameter. For the same test, the other varieties have an accuracy below the accuracy of “kom kom”.
更多查看译文
关键词
peanut varieties,deep learning,genetically,flower-related
AI 理解论文
溯源树
样例
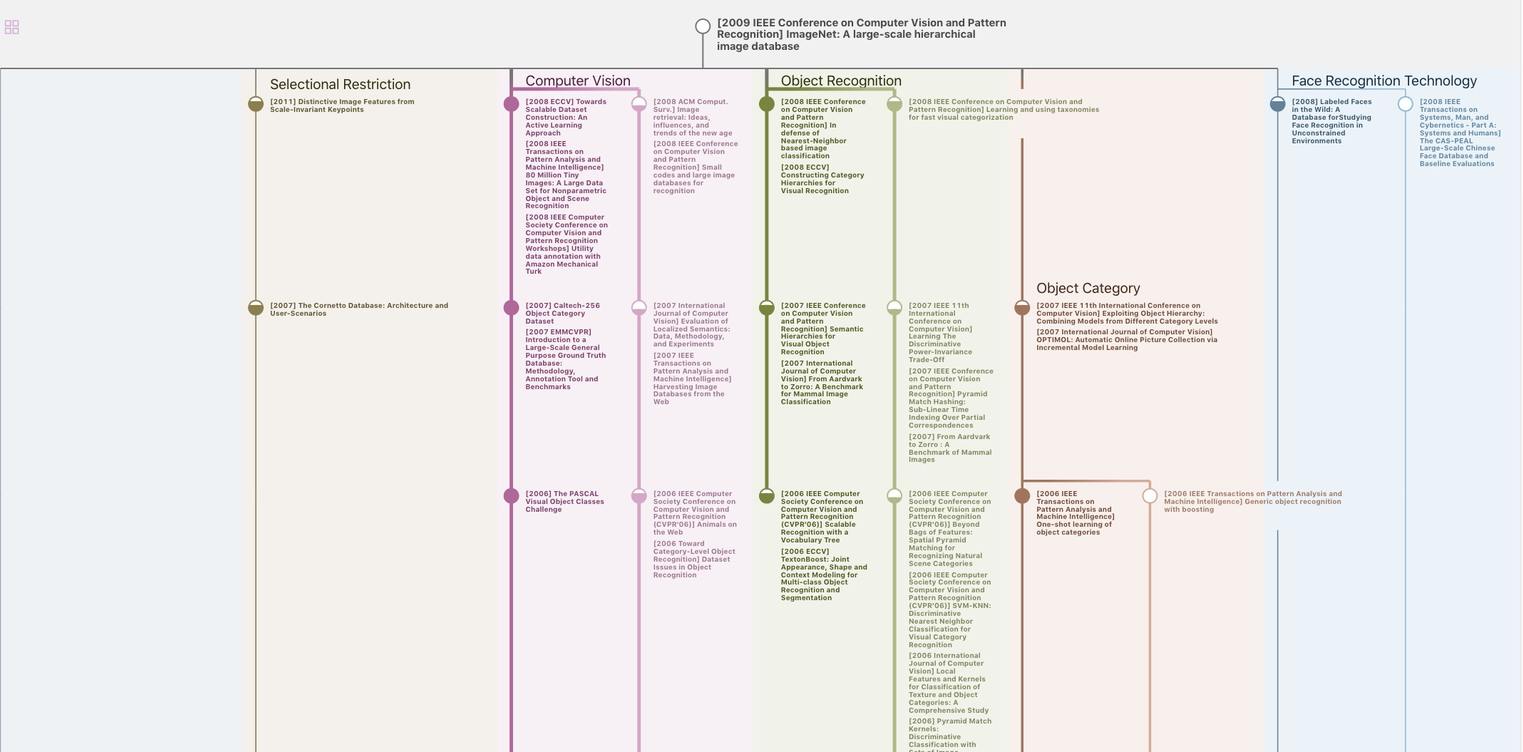
生成溯源树,研究论文发展脉络
Chat Paper
正在生成论文摘要