Generalizing Voice Presentation Attack Detection to Unseen Synthetic Attacks and Channel Variation
Advances in Computer Vision and Pattern Recognition(2023)
摘要
Automatic Speaker Verification (ASV) systems aim to verify a speaker's claimed identity through voice. However, voice can be easily forged with replay, text-to-speech (TTS), and voice conversion (VC) techniques, which may compromise ASV systems. Voice presentation attack detection (PAD) is developed to improve the reliability of speaker verification systems against such spoofing attacks. One main issue of voice PAD systems is its generalization ability to unseen synthetic attacks, i.e., synthesis methods that are not seen during training of the presentation attack detection models. We propose one-class learning, where the model compacts the distribution of learned representations of bona fide speech while pushing away spoofing attacks to improve the results. Another issue is the robustness to variations of acoustic and telecommunication channels. To alleviate this issue, we propose channel-robust training strategies, including data augmentation, multi-task learning, and adversarial learning. In this chapter, we analyze the two issues within the scope of synthetic attacks, i.e., TTS and VC, and demonstrate the effectiveness of our proposed methods.
更多查看译文
AI 理解论文
溯源树
样例
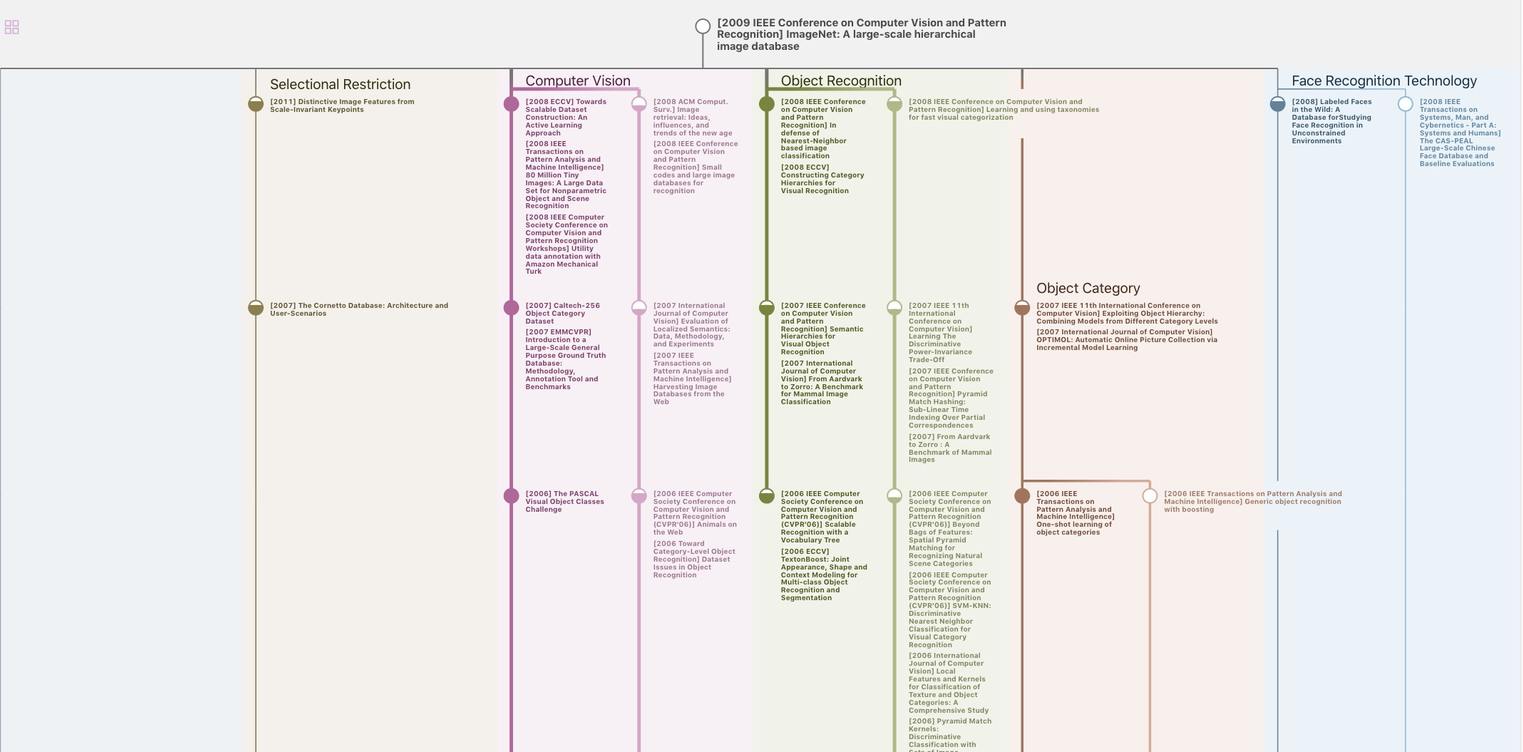
生成溯源树,研究论文发展脉络
Chat Paper
正在生成论文摘要