Deep Learning Based Detachment Segmentation: the MIRET Approach
2022 IEEE International Conference on Metrology for Extended Reality, Artificial Intelligence and Neural Engineering (MetroXRAINE)(2022)
摘要
Among infrastructure diagnostics, the maintenance of Transportation Tunnel (TT) is of paramount importance. On the one hand, a predictive maintenance process would allow for safety compliance, preventive corrective actions, and increased lifetime for the asset, which results in improved efficiency of the overall infrastructure. On the other hand, existing methods to perform diagnosis and detection of these anomalies are inexpensive and time-consuming. Thus, ETS and RMT foresee the development of deep learning-based methods for the segmentation of defects on TT images. This stands as the technological pillar of two innovative ETS projects: the innovative multi-dimensional survey system (ARCHITA), and a new approach for the Management and Identification of the Risk for Existing Tunnels (MIRET). The focus of this communication is the segmentation of superficial detachments on data of concrete type Transportation Tunnels, using convolutional neural networks trained in an adversarial fashion.
更多查看译文
关键词
predictive maintenance, convolutional neural network, semantic segmentation, generative adversarial network
AI 理解论文
溯源树
样例
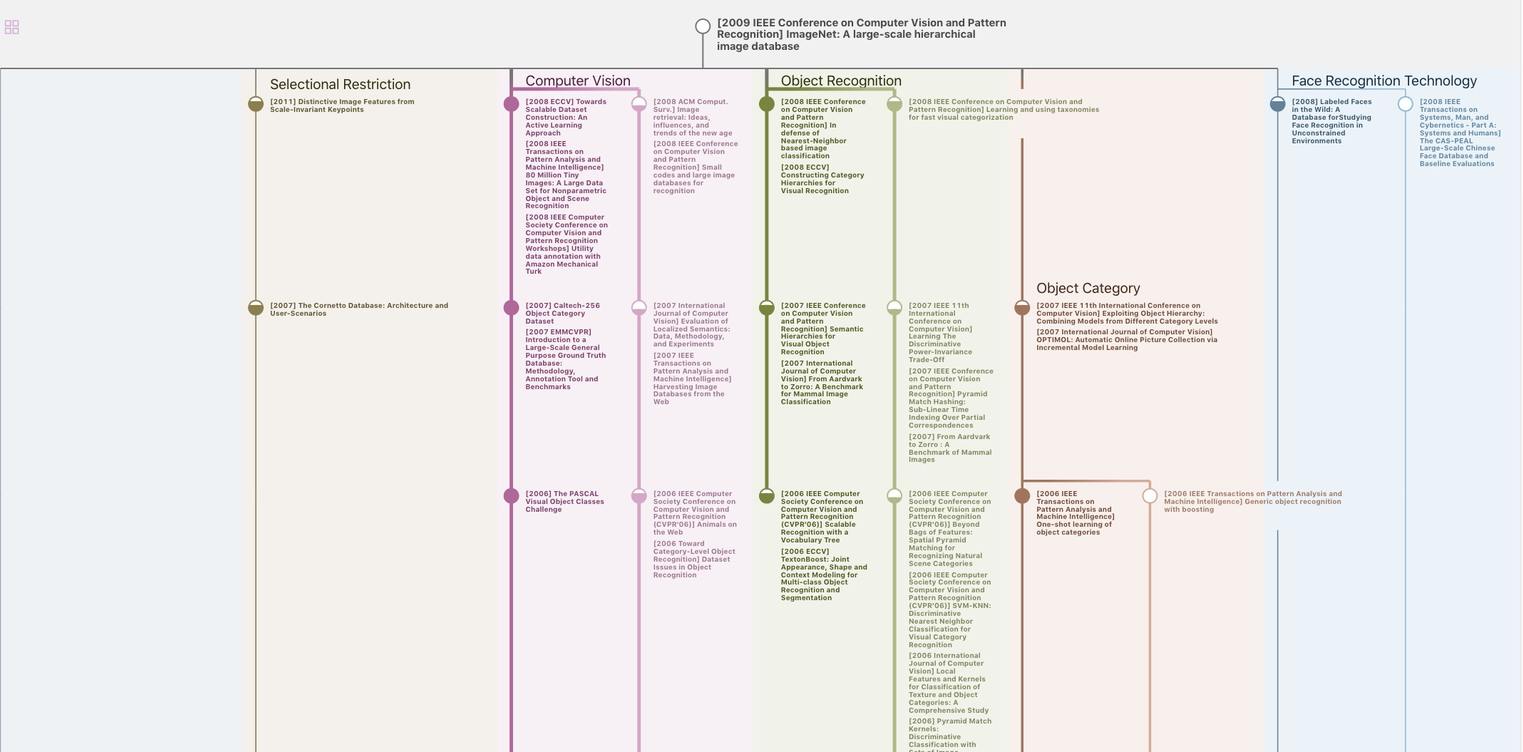
生成溯源树,研究论文发展脉络
Chat Paper
正在生成论文摘要