Hybrid machine learning model of coupled carbon and water cycles 
crossref(2023)
摘要
<div class="page" title="Page 1"> <div class="layoutArea"> <div class="column"> <p>There is evidence for a strong coupling between the terrestrial carbon and water cycles and that these cycles should be studied as an interconnected system (Humphrey et al. 2018). One of the key methods to numerically represent the Earth system is process based modelling, which is, however, still subject to large uncertainties, e.g., due to wrong or incomplete process knowledge (Bonan and Doney 2018). Such models are often rigid and only marginally informed by Earth observations. This is where machine learning (ML) approaches can be advantageous, due to their ability to learn from data in a flexible way. These methods have their own shortcomings, such as their “black-box” nature and lack of physical consistency.</p> <div class="page" title="Page 1"> <div class="layoutArea"> <div class="column"> <p>Recently, it has been suggested by Reichstein et al. (2019) to combine process knowledge with ML algorithms to model environmental processes. The so-called hybrid modelling approach has already been used to model different components of terrestrial water storage (TWS) in a global hydrological model (Kraft et al. 2022). This study follows-up on this work with the objective to improve the parameterization of some processes (e.g., soil moisture) and to couple the model with the carbon cycle. The coupling could potentially reduce model uncertainties and help to better understand water-carbon interactions.</p> <div class="page" title="Page 1"> <div class="layoutArea"> <div class="column"> <p>The proposed hybrid model of the coupled water and carbon cycles is forced with reanalysis data from ERA-5, such as air temperature, net radiation, and CO<sub>2 </sub>concentration from CAMS. Water-carbon cycle processes are constrained using observational data products of water-carbon cycles. The hybrid model uses a long short-term memory (LSTM) model—a member of the recurrent neural networks family—at its core for processing the time-series Earth observation data. The LSTM simulates a number of coefficients which are used as parameters in the conceptual model of water and carbon cycles. Some of the key processes represented in the conceptual model are evapotranspiration, snow, soil moisture, runoff, groundwater, water use efficiency (WUE), ecosystem respiration, and net ecosystem exchange. The model partitions TWS into different components and it can be used to assess the impact of different TWS components on the CO<sub>2</sub> growth rate. Moreover, we can assess the learned system behaviors of water and carbon cycle interactions for different ecosystems.</p> <p>References:</p> <div class="page" title="Page 2"> <div class="layoutArea"> <div class="column"> <div class="page" title="Page 2"> <div class="layoutArea"> <div class="column"> <p>Bonan, Gordon B, and Scott C Doney. 2018. “Climate, Ecosystems, and Planetary Futures: The Challenge to Predict Life in Earth System Models.” Science 359 (6375): eaam8328.</p> <p>Humphrey, Vincent, Jakob Zscheischler, Philippe Ciais, Lukas Gudmundsson, Stephen Sitch, and Sonia I Seneviratne. 2018. “Sensitivity of Atmospheric CO<sub>2</sub> Growth Rate to Observed Changes in Terrestrial Water Storage.” Nature 560 (7720): 628–31.</p> <p>Kraft, Basil, Martin Jung, Marco Körner, Sujan Koirala, and Markus Reichstein. 2022. “Towards Hybrid Modeling of the Global Hydrological Cycle.” Hydrology and Earth System Sciences 26 (6): 1579–1614.</p> <p>Reichstein, Markus, Gustau Camps-Valls, Bjorn Stevens, Martin Jung, Joachim Denzler, Nuno Carvalhais, et al. 2019. “Deep Learning and Process Understanding for Data-Driven Earth System Science.” Nature 566 (7743): 195–204.</p> </div> </div> </div> </div> </div> </div> </div> </div> </div> </div> </div> </div> </div> </div> </div>
更多查看译文
AI 理解论文
溯源树
样例
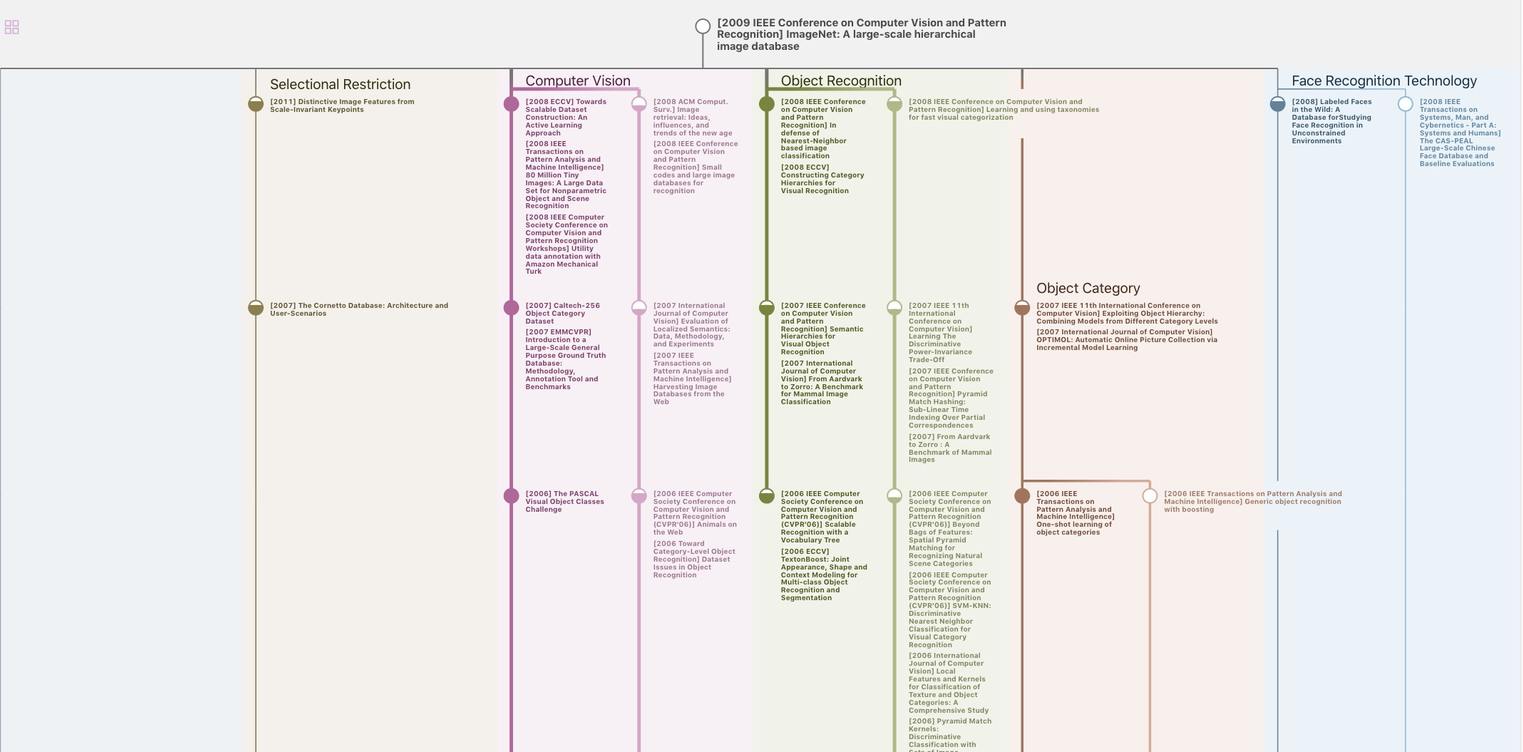
生成溯源树,研究论文发展脉络
Chat Paper
正在生成论文摘要