Monitoring and Interpreting Shanghai Maglev Deformation Using Deep Clustering on MT-InSAR Analyses
crossref(2023)
摘要
<p>Multi-temporal InSAR (MT-InSAR) technique has been widely used in the earth observation field. However, there are still challenges in the high-resolution monitoring and interpreting of urban infrastructures, especially for long-term time series analysis. We develop a data-driven post-processing method to provide a new solution to using MT-InSAR analyses in urban infrastructure health monitoring. We use a deep learning-based clustering method to classify different displacement temporal evolution patterns along Shanghai maglev from 7 years of TerraSAR-X observations (2013 to 2020). Our study region is observed by the satellite with alternating viewing angles between consecutive passes. We jointly estimate the orbital error per epoch to combine the two interweaving time series with different viewing geometries. We include spatial information of observation points for more reliable clustering. We then interpret the cluster results with maglev structural knowledge and surrounding groundwater level change. Different from previous classification methods, the deep learning-based clustering method is independent of predefined deformation models, allowing the identification of previously unknown types of deformation signals. Our preliminary results highlight the potential of applying deep clustering for MT-InSAR time series analyses for future automated structural health monitoring.</p>
更多查看译文
AI 理解论文
溯源树
样例
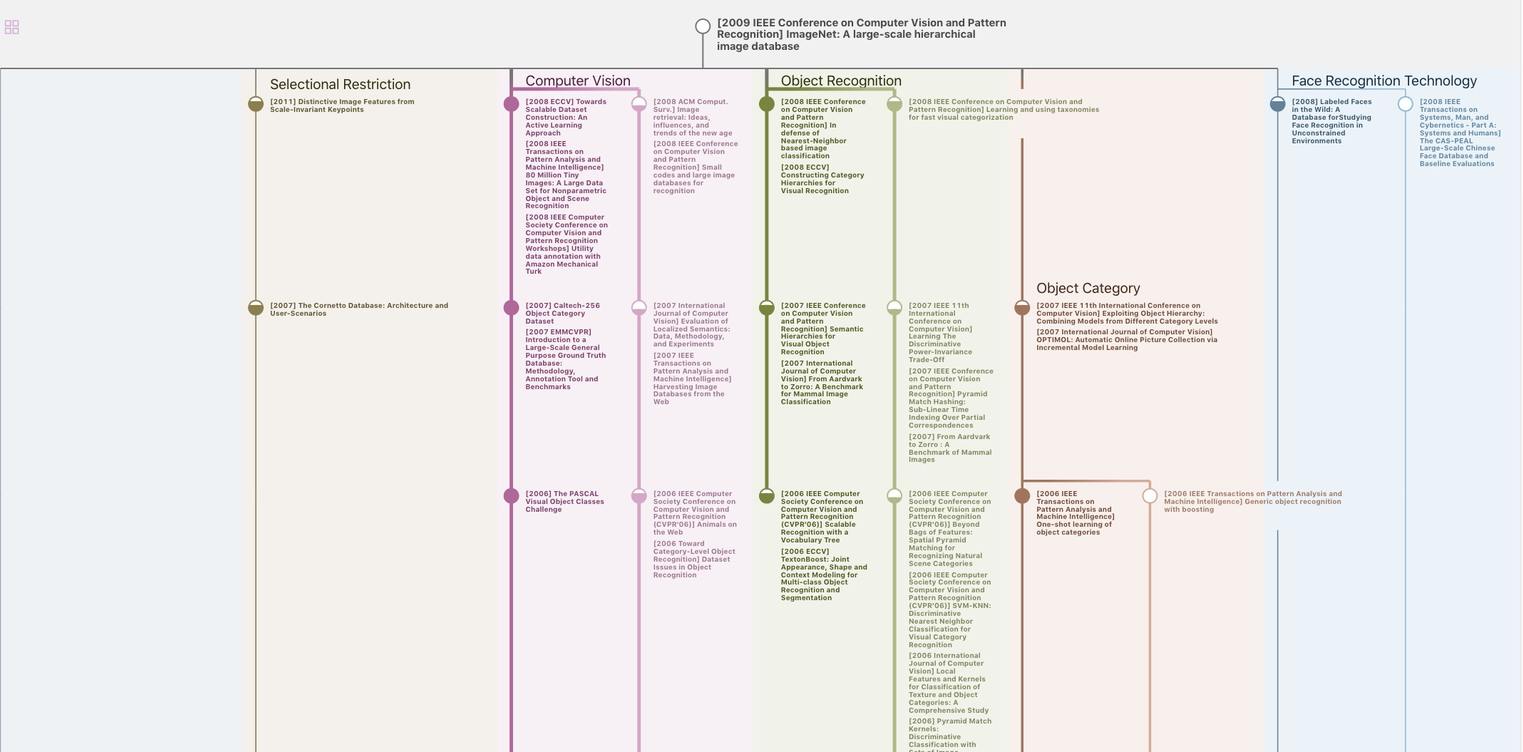
生成溯源树,研究论文发展脉络
Chat Paper
正在生成论文摘要