Identification of multiple radioisotopes through convolutional neural networks trained on 2-D transformed gamma spectral data from CsI (Tl) spectrometer
SSRN Electronic Journal(2023)
摘要
A study is conducted to improve the performance of Convolutional Neural Networks (CNNs)-based radioisotope identification (RIID). This study introduces a novel method to transform the dimensional structure of a radio-isotope (RI) mixture spectra as training inputs, for the purpose of extending the receptive field and improving RIID performance of the model. The one-dimensional (1-D) composite spectra of radioisotopic mixtures as a dataset to be used as train and test data for a CNNs model are generated by linearly combining the normalized element gamma-ray spectra of single RIs obtained experimentally with the developed CsI(Tl) spectrometer. One more dataset for training and testing another a CNNs model is produced by reshaping the same 1-D composite spectra into two-dimensional (2-D) image data with multiple columns and rows. After the structure and hyper -parameters of the model are determined, each model is trained and tested with each of the two prepared datasets to simultaneously predict the probabilities as the relative count distributions for eight RI classes. The RIID performance of the CNNs model trained with the reshaped 2-D data is compared with that of the model trained with the 1-D data. The performance of each trained model is evaluated by mean magnitude of relative error (MMRE) as performance evaluation metrics. The results show that the model trained with 2-D inputs outperforms the model trained with 1-D inputs and effectively identifies unknown multiple radioisotopes. The proposed method to transform 1-D data into 2-D data represents a promising approach for the CNNs-based RIID of a scintillator spectrometer, especially when multiple peaks or RIs need to be resolved.
更多查看译文
关键词
Radioisotope identification,RIID,Convolutional neural networks,Artificial neural network,CsI(Tl),Gamma-ray spectrum
AI 理解论文
溯源树
样例
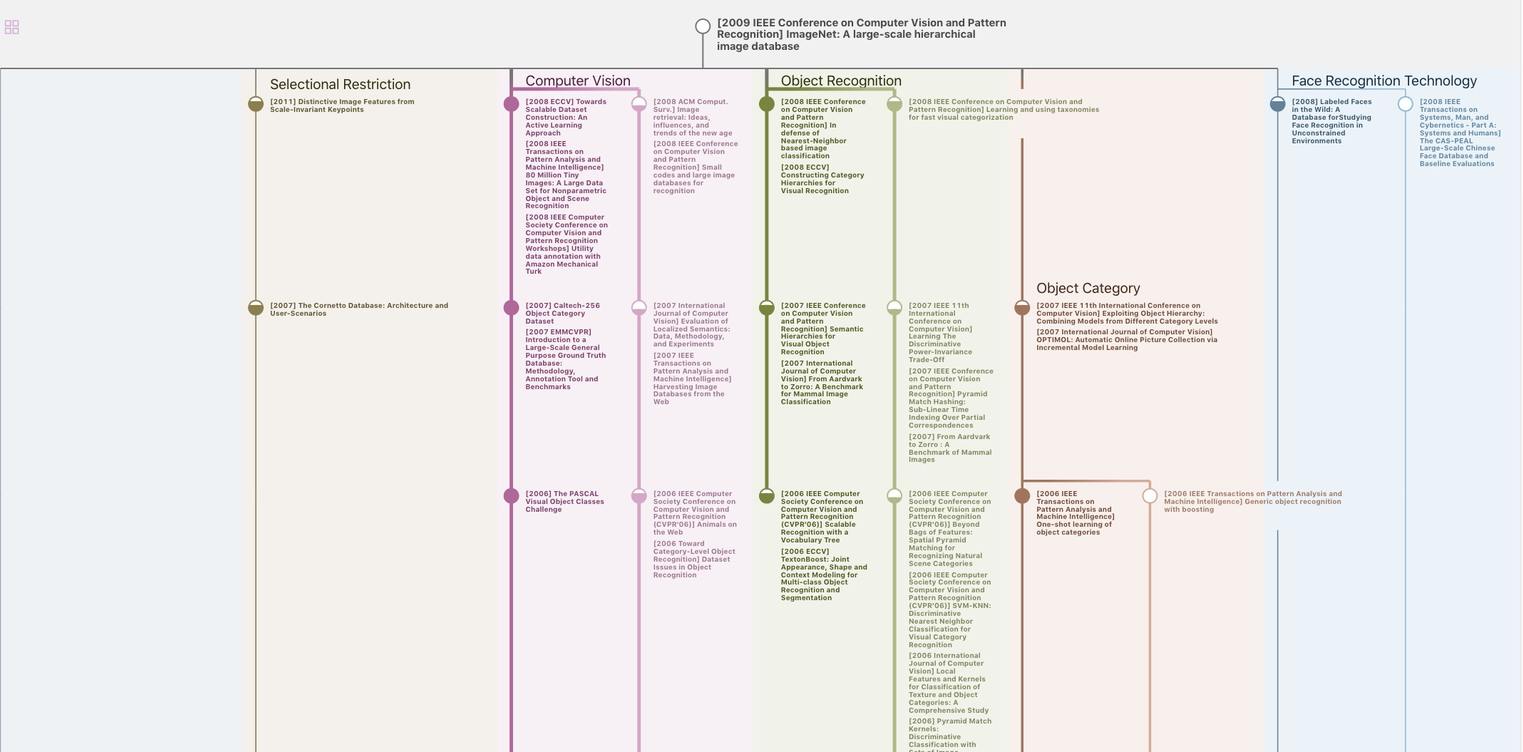
生成溯源树,研究论文发展脉络
Chat Paper
正在生成论文摘要