Recorded recurrent deep reinforcement learning guidance laws for intercepting endoatmospheric maneuvering missiles
DEFENCE TECHNOLOGY(2024)
摘要
This work proposes a recorded recurrent twin delayed deep deterministic (RRTD3) policy gradient algorithm to solve the challenge of constructing guidance laws for intercepting endoatmospheric maneuvering missiles with uncertainties and observation noise. The attack -defense engagement scenario is modeled as a partially observable Markov decision process (POMDP). Given the benefits of recurrent neural networks (RNNs) in processing sequence information, an RNN layer is incorporated into the agent's policy network to alleviate the bottleneck of traditional deep reinforcement learning methods while dealing with POMDPs. The measurements from the interceptor's seeker during each guidance cycle are combined into one sequence as the input to the policy network since the detection frequency of an interceptor is usually higher than its guidance frequency. During training, the hidden states of the RNN layer in the policy network are recorded to overcome the partially observable problem that this RNN layer causes inside the agent. The training curves show that the proposed RRTD3 successfully enhances data efficiency, training speed, and training stability. The test results confirm the advantages of the RRTD3-based guidance laws over some conventional guidance laws. (c) 2023 China Ordnance Society. Publishing services by Elsevier B.V. on behalf of KeAi Communications Co. Ltd. This is an open access article under the CC BY license (http://creativecommons.org/licenses/by/ 4.0/).
更多查看译文
关键词
Endoatmospheric interception,Missile guidance,Reinforcement learning,Markov decision process,Recurrent neural networks
AI 理解论文
溯源树
样例
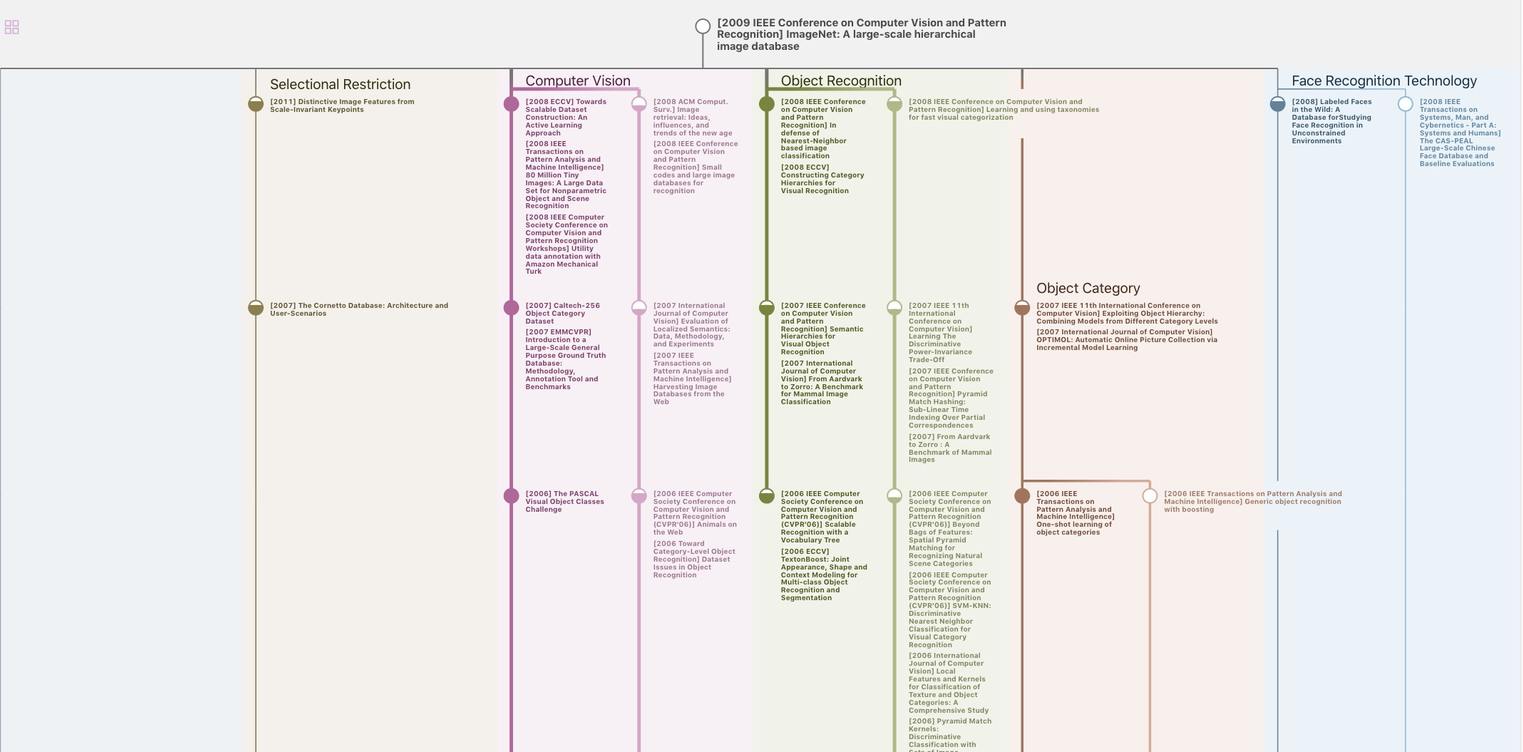
生成溯源树,研究论文发展脉络
Chat Paper
正在生成论文摘要