Frame-Recurrent Video Crowd Counting
IEEE Transactions on Circuits and Systems for Video Technology(2023)
摘要
Since video data contains temporal information, video crowd counting demonstrates more potential than single-frame crowd counting for scenarios requiring high accuracy. However, learning robust relationships among frames efficiently and cheaply is very challenging. Existing methods for video crowd counting lack explicit temporal correlation modeling and robustness, and they are complex. In this paper, we propose the Frame-Recurrent Video Crowd Counting (FRVCC) framework to solve these issues. Specifically, we design a frame-recurrent manner to recursively relate the density maps in the temporal dimension, which efficiently explores long-term inter-frame knowledge and ensures the continuity of feature map responses. FRVCC consists of three plug-in modules: an optical flow estimation module, a single-frame counting module, and a density map fusion module. For the fusion module, we propose the ResTrans network to robustly learn complementary features between visual-based and correlation-based feature maps through residual strategy and vision transformer. To constrain the output distribution to be consistent with the ground truth distribution, we introduce an adversarial loss to rectify the training process. Additionally, we release a large-scale synthetic video crowd-counting dataset, CrowdXV, to evaluate the proposed method and further improve its performance. We have conducted extensive experiments on several video-counting datasets. The results demonstrate that FRVCC achieves state-of-the-art performance and, concurrently, high generalization, high flexibility, and less complexity.
更多查看译文
关键词
Video crowd counting,feature fusion,synthetic dataset
AI 理解论文
溯源树
样例
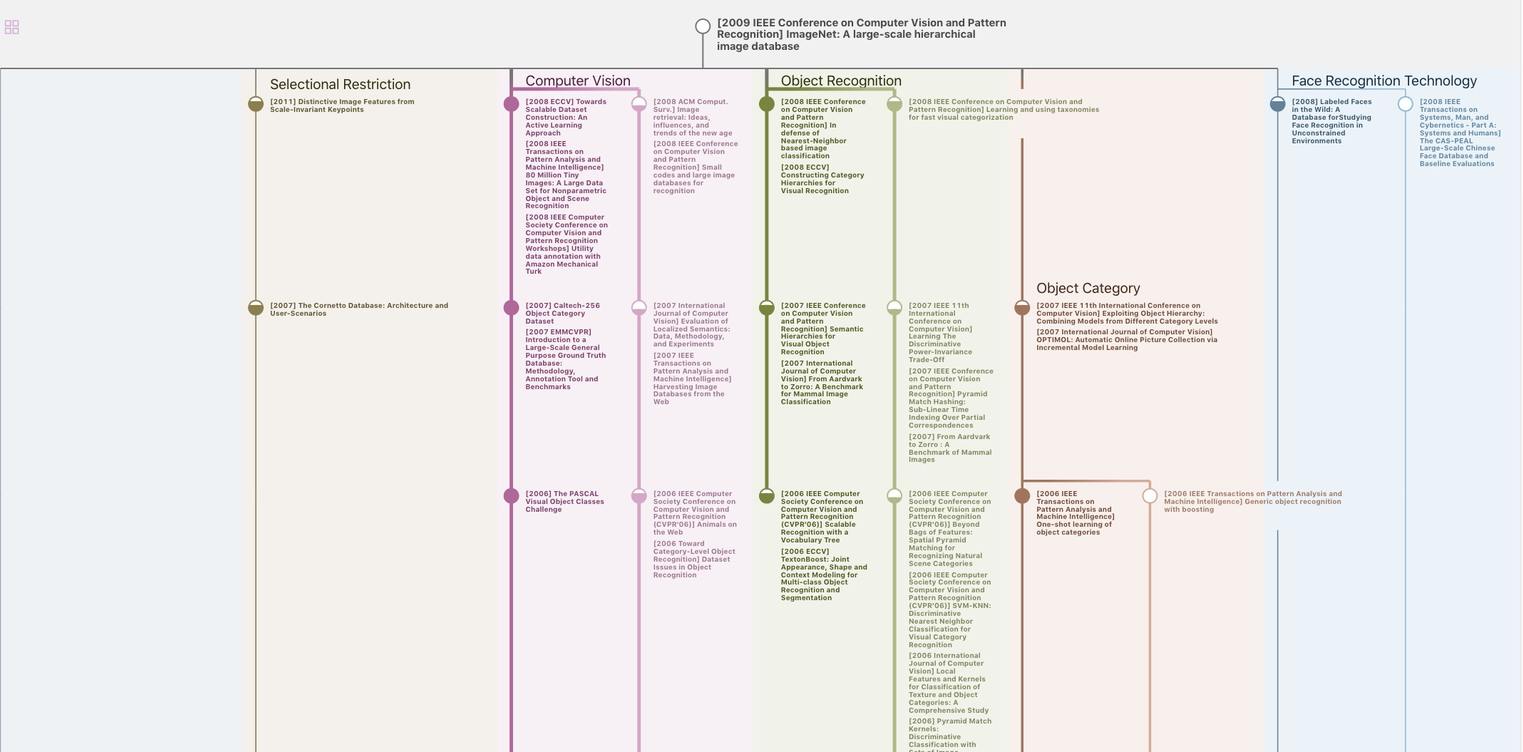
生成溯源树,研究论文发展脉络
Chat Paper
正在生成论文摘要