Deep learning for autonomous vehicles
Deep Learning and Its Applications for Vehicle Networks(2023)
摘要
Visual perception and image-based control are fundamental aspects of autonomous navigation. The first directly represents how the vehicle sees its surroundings and provides information necessary to plan optimal and safe trajectories. The second, on the other hand, ensures that the vehicle performs the desired lateral motion by providing the adequate steering actions. Uncertainties are common in real applications of autonomous vehicles, such as variations in luminosity, GPS signals, tire friction nonlinearities, and driveline behavior. Thus, this chapter presents methods for the optimal estimation of uncertainty via an evolutionary algorithm that evaluates the reward function based only on measured input and output data.This chapter presented a hybrid control method resulting from the combination of deep learning with robust control for autonomous vehicles. This framework can enhance the control performance when compared with standard deep learning control. The system was identified based on visual information available through the embedded camera. Such procedure circumvents the need for an exact description of the vehicle’s physical model. In addition, the proposed method deals with structured uncertainties, which is essential for autonomous navigation in urban environments. For uncertainty estimation, an evolutionary search method is presented to fine-tune the uncertainty matrices used by the robust controllers. Nonetheless, other methods, such as reinforcement learning and filtering techniques, can be applied to estimate the uncertainties in this architecture. At last, the designer can adopt different uncertainty estimation and robust control approaches to compose the overall architecture. This aspect highlights the flexibility and modularity featured by the vision-based control framework.
更多查看译文
关键词
deep learning,autonomous vehicles
AI 理解论文
溯源树
样例
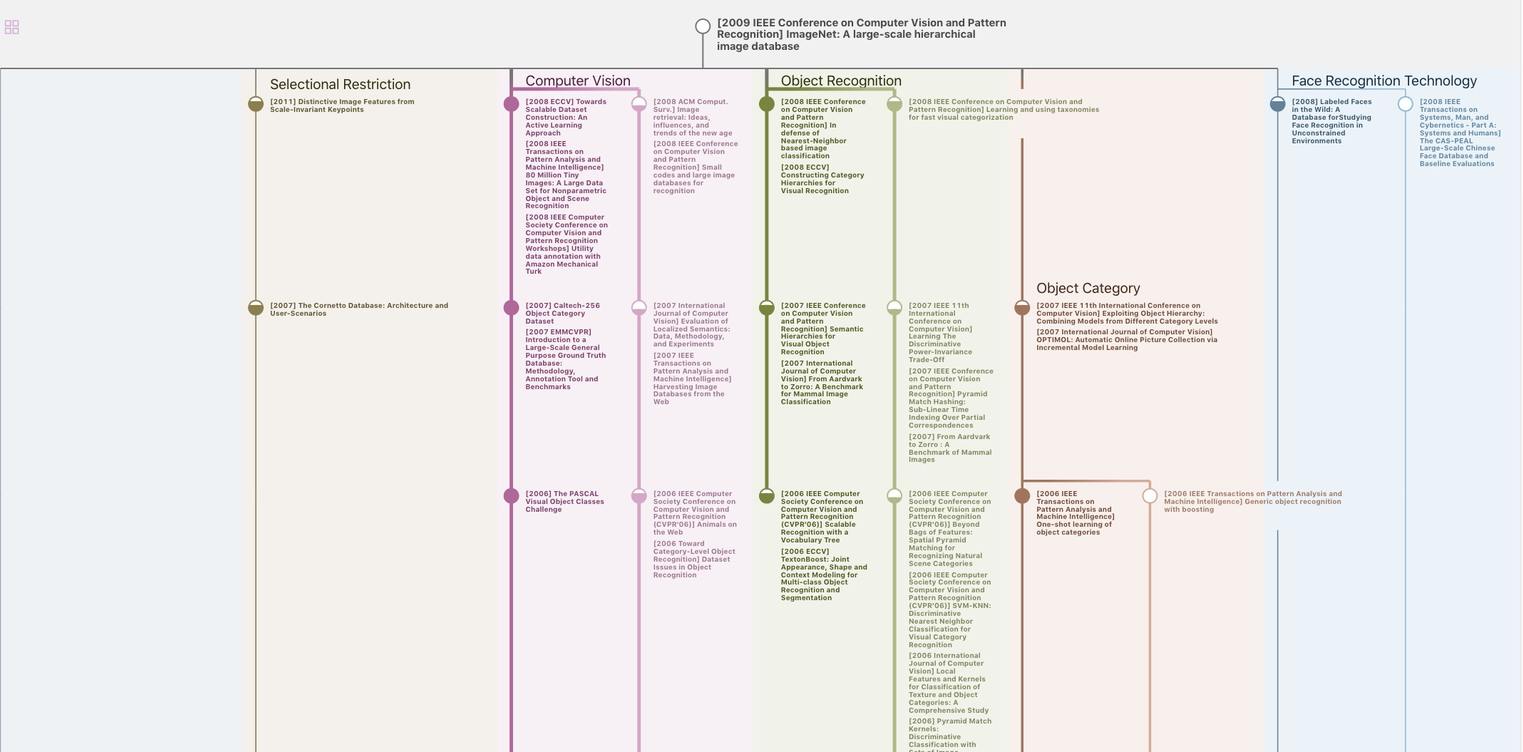
生成溯源树,研究论文发展脉络
Chat Paper
正在生成论文摘要