Real-time Personalized Guest Experiences using Product Attribute Information on Vanilla Hardware
2023 IEEE 39th International Conference on Data Engineering Workshops (ICDEW)(2023)
摘要
User experience in digital retail critically depends on high-quality personalized recommendations. A large pool of guests, a large catalog of items (millions of items) and real-time low latency requirements for recommendations make such systems difficult to design and implement. In this paper, we discuss our early experiences in building and deploying a real-time inferencing framework (with 95 percentile latency ≤50ms) by deploying microservices framework on vanilla hardware and serving thousands of recommendations per second. Our recommendation framework builds on the general knowledge that purchase of a product by a guest is ultimately driven by its attributes such as color, brand, flavor, size or price. While this information is highlighted in product pages and all personalization algorithms tend to use implicit attributes and attribute similarities to recommend products to the guests, this information is not directly utilized to empower personalized guest experiences. We built a Guest’s Attribute Preferences (GAP) model that scores a guest’s preferences to attributes to improve product recommendations in app and website. Several AB tests reveal that this results in significant improvement in incremental interaction and revenue (upto 3x improvements) over hadoop-based production system recommendations in different use cases. We also discuss system aspects of GAP, where a single pod can execute hundreds of recommendation requests per second with 50 millisecond latency. Today, systems backed by GAP contribute to significant user engagement with product recommendations in Target’s website and App.
更多查看译文
关键词
personalization,product attributes,real-time recommendations
AI 理解论文
溯源树
样例
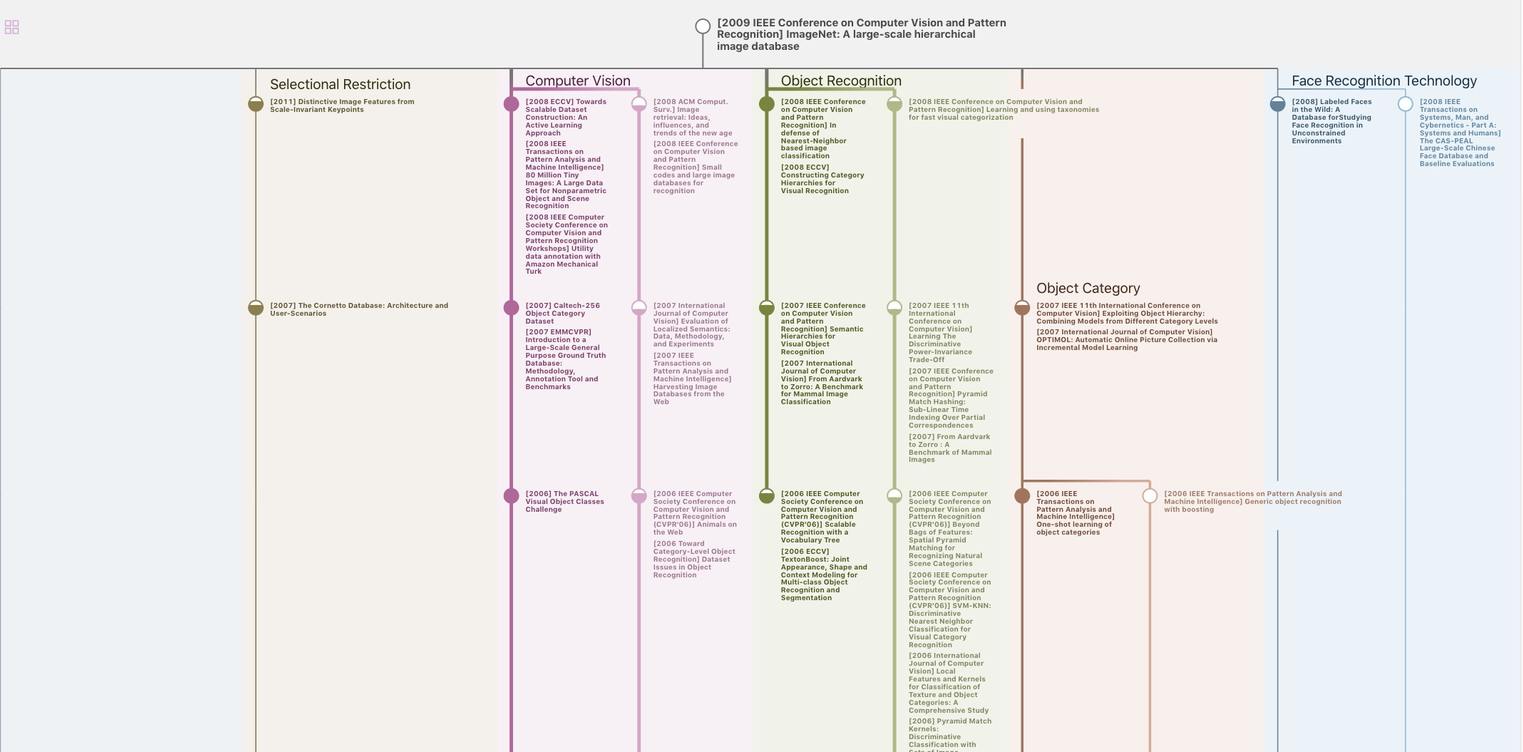
生成溯源树,研究论文发展脉络
Chat Paper
正在生成论文摘要