Towards breastfeeding self-efficacy and postpartum depression estimation based on analysis of free-speech interviews through natural language processing
18th International Symposium on Medical Information Processing and Analysis(2023)
摘要
Edinburgh Postpartum Depression (EPDS) and Breastfeeding Self-Efficacy (BSES) scales are standardized questionnaires to screen for postpartum depression and breastfeeding performance self-perception. On the other hand, Natural Language Processing (NLP) is a machine learning technique that analyses the human language to extract relevant and computer-interpretable information. In this work we proposed the application of an NLP toolchain that includes a typical preprocessing stage and the probabilistic topic modeling performed through the Latent Dirichlet Allocation (LDA) to find out the two most relevant topics within each of six study groups (low, medium, and high scores of BSES and EPDS). Each topic LDA-modeled consisted of 30-word/terms (tokens) which are organized in Venn diagrams, contrasting the mutually exclusive tokens within the low and high scores on each scale. Coherence and log-Perplexity topic modeling performance metrics, were computed. We found that LDA-models have distinguishable tokens between low and high scores of the BSES and EPDS. However, the most remarkable findings were two subset of tokens, one related to newborn care and another to newborn intake, respectively correlated to low and high postpartum depression risk according to EPDS.
更多查看译文
关键词
Exclusive Breastfeeding, Natural Language Processing, Edinburgh Postpar-tum Depression Scale, Breastfeeding Self-Efficacy Scale, Latent Dirichlet Allocation
AI 理解论文
溯源树
样例
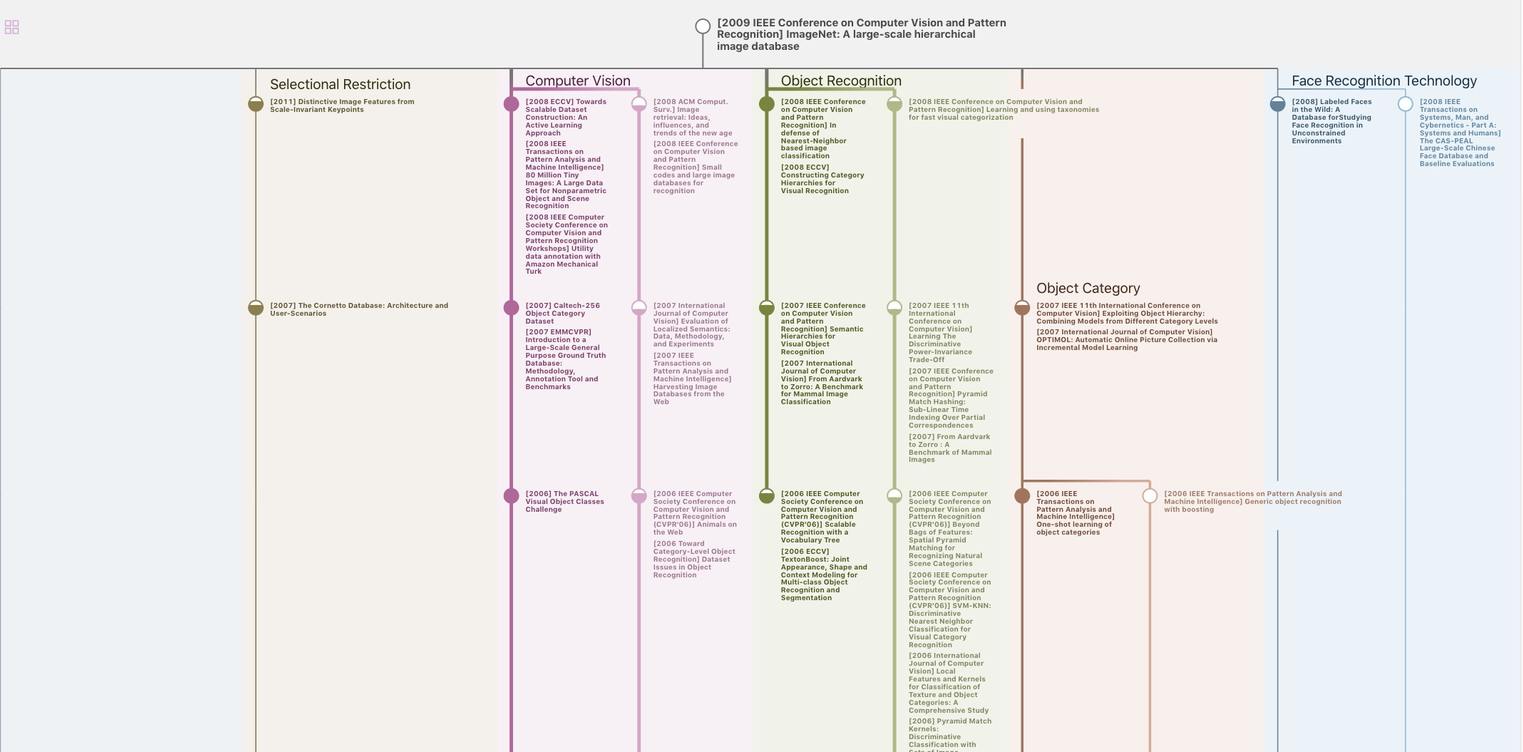
生成溯源树,研究论文发展脉络
Chat Paper
正在生成论文摘要