Adaptive methods to quickly estimate psychometric functions: the case of Psi-marg-grid and the effect of non-monotony
Journal of Vision(2022)
摘要
Introduction: Estimating psychometric thresholds can be challenging, especially stereoacuities (Chopin et al., 2019). Adaptive methods used for estimation generally assume monotonically increasing psychometric functions (Levitt, 1971), which can be incorrect, for example when testing stereoacuities (Kane et al., 2014). I wondered how adaptive methods are affected by non-monotony in psychometric functions. Methods: I performed Monte-Carlo simulations of 90-trial threshold estimations following six different methods: (1) the method of constant stimuli (MOCS), ZEST (King-Smith et al., 1991), Psi (Kontsevich & Tyler, 1999), Psi-marginal (Prins, 2013), Psi-grid (Doire, Brookes, & Naylor, 2017) and a new algorithm Psi-marg-grid, mixing Psi-Marg’s marginalization on nuisance parameters and Psi-grid’s adaptive search grid. I tested four conditions under which the simulated ideal-observer’s function and/or the algorithm’s assumed function were monotonic or non-monotonic. Parameters other than the threshold were random. The bias was measured by the median difference to the simulated threshold, the accuracy by the mean absolute error and the test-retest by the limit of agreement between two simulations of a given threshold (log units). The chance level was calculated as the probability to obtain a threshold better than a critical value while simulating a random observer. Results: When assuming monotony, no algorithms could issue meaningful estimates with non-monotonic observers (bias>11822%). When assuming non-monotony, Psi-marg-grid showed the best results (bias 0.6%, accuracy 8.92%, test-retest 0.159, chance level 1%). Only Psi-marg had a better test-retest (0.154) but it lacked accuracy with non-monotonic observers (error 29.4%). MOCS had the worst test-retest (0.6) and chance level (53.2%). Psi’s and Psi-grid’s chance levels were high (18.9% and 17.8% respectively). ZEST lacked accuracy with non-monotonic observers (error 122%). Conclusion: it is important to use methods assuming non-monotony, because none of the tested methods were otherwise robust to non-monotony in the observer’s psychometric functions. When assuming non-monotony, Psi-marg-grid was the best tested method.
更多查看译文
关键词
psychometric functions,adaptive methods,estimate,psi-marg-grid,non-monotony
AI 理解论文
溯源树
样例
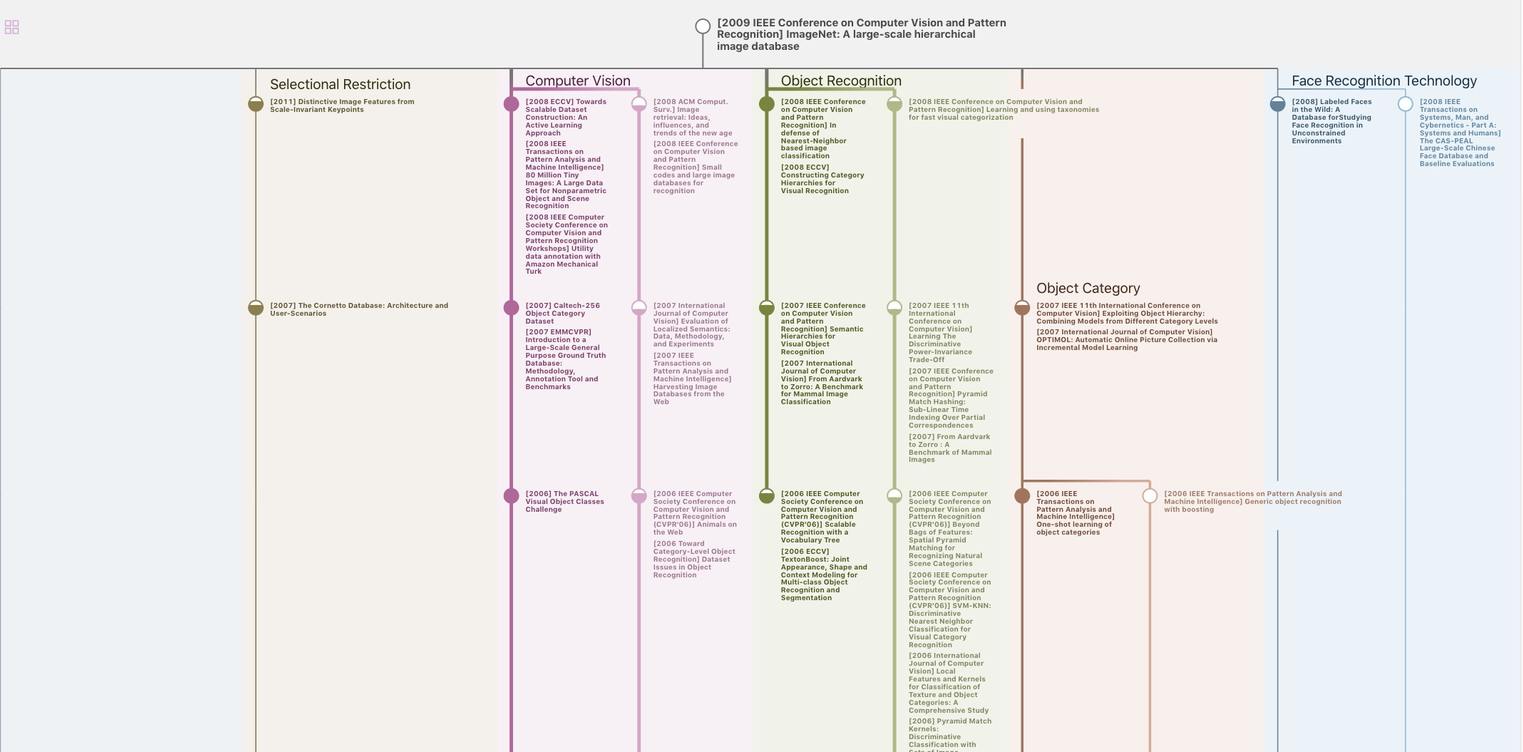
生成溯源树,研究论文发展脉络
Chat Paper
正在生成论文摘要