Toward Realistic 3D Human Motion Prediction With a Spatio-Temporal Cross- Transformer Approach
IEEE Transactions on Circuits and Systems for Video Technology(2023)
摘要
Human motion prediction intends to predict how humans move given a historical sequence of 3D human motions. Recent transformer-based methods have attracted increasing attentions and demonstrated their promising performance in 3D human motion prediction. However, existing methods generally decompose the input of human motion information into spatial and temporal branches in a separate way and seldom consider their inherent coherence between the two branches, hence often failing to register the dynamic spatio-temporal information during the training process. Motivated by these issues, we propose a spatio-temporal cross-transformer network (STCT) for 3D human motion predictions. Specifically, we investigate various types of interaction methods (i.e., Concatenation Interaction, Msg token interaction, and Cross-transformer) to capture the coherence of the spatial and temporal branches. According to the obtained results, the proposed cross-transformer interaction method shows its superiority over other methods. Meanwhile, considering that most existing works treat the human body as a set of 3D human joint positions, the predicted human joints are proportionally less appropriate to the realistic human body due to unreasonable bone length and non-plausible poses as time progresses. We further resort to the bone constraints of human mesh to produce more realistic human motions. By fitting a parametric body model (i.e., SMPL-X model) to the predicted human joints, a reconstruction loss function is proposed to remedy the unreasonable bone length and pose errors. Comprehensive experiments on AMASS and Human3.6M datasets have demonstrated that our method achieves superior performance over compared methods.
更多查看译文
关键词
3d,motion,prediction,spatio-temporal,cross-transformer
AI 理解论文
溯源树
样例
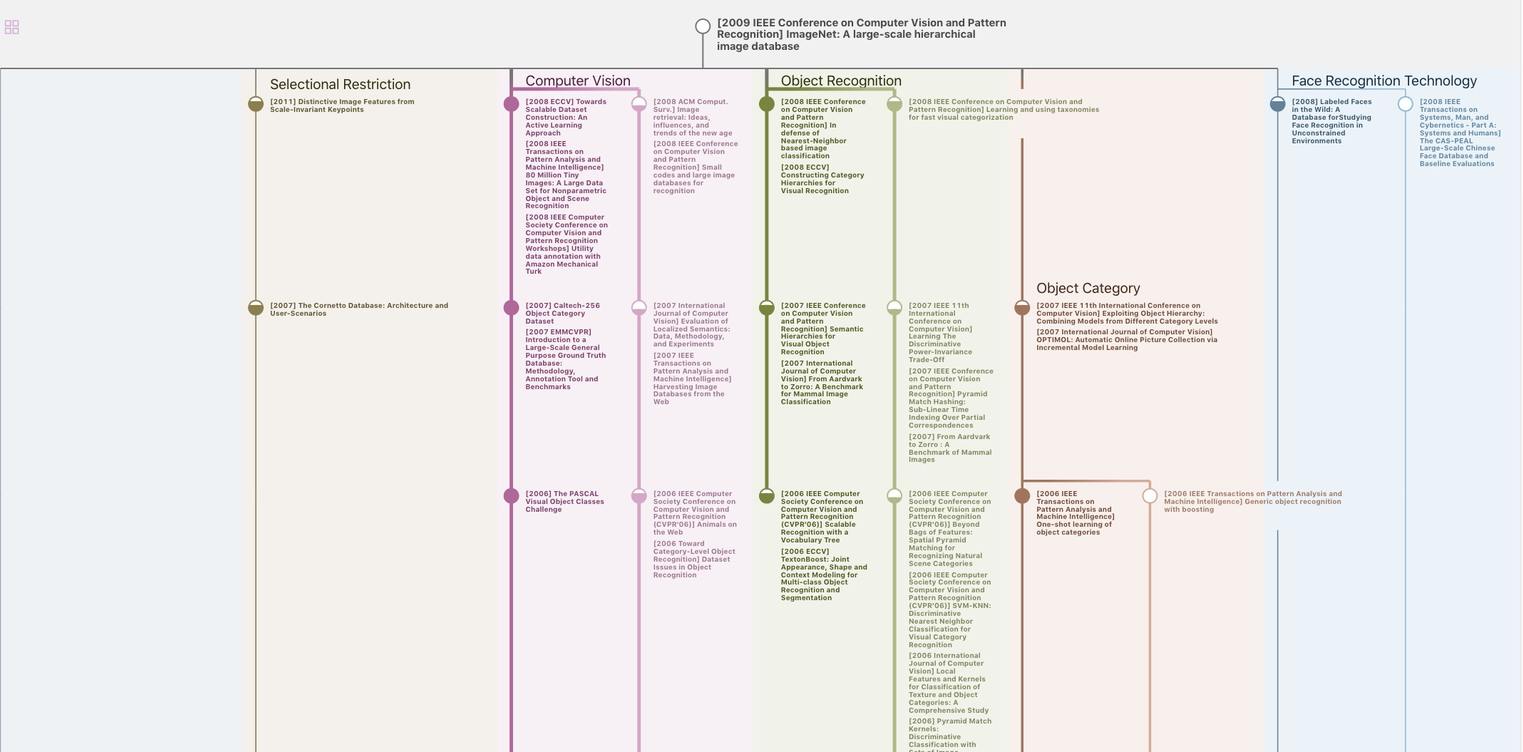
生成溯源树,研究论文发展脉络
Chat Paper
正在生成论文摘要