FAIRification, Quality Assessment, and Missingness Pattern Discovery for Spatiotemporal Photovoltaic Data
2022 IEEE 49th Photovoltaics Specialists Conference (PVSC)(2022)
摘要
Due to the fast growth of the photovoltaic (PV) market, more power plants have become available with data accessible for power forecasting and long-term reliability assess-ment. The accuracy of the modeling on this data is influenced heavily by the quality of the data and can be improved through data imputation to fill missing gaps. In this study, we introduce a FAIRification framework for ingesting data from PV power plants. This process improves the efficiency of modeling on time series data provided by different labs and companies through an automated ingestion process. We take this analysis further by investigating the use of different imputation methods for filling in large chunks of missing data. Specifically, mean interpolation, linear interpolation, and k-nearest neighbors (KNN) were used in this report to fill in missing data for module temperature and power in a PV time series. It was found that the KNN algorithm outperforms the other methods due to its ability to leverage spatial coherence from nearby systems. These results point towards the potential use of a spatio-temporal graph neural network (st-GNN) in order to impute data using spatial coherence between systems in a large data set with time series data from many PV power plants.
更多查看译文
关键词
FAIRification,Spatiotemporal GNN,Missing-ness
AI 理解论文
溯源树
样例
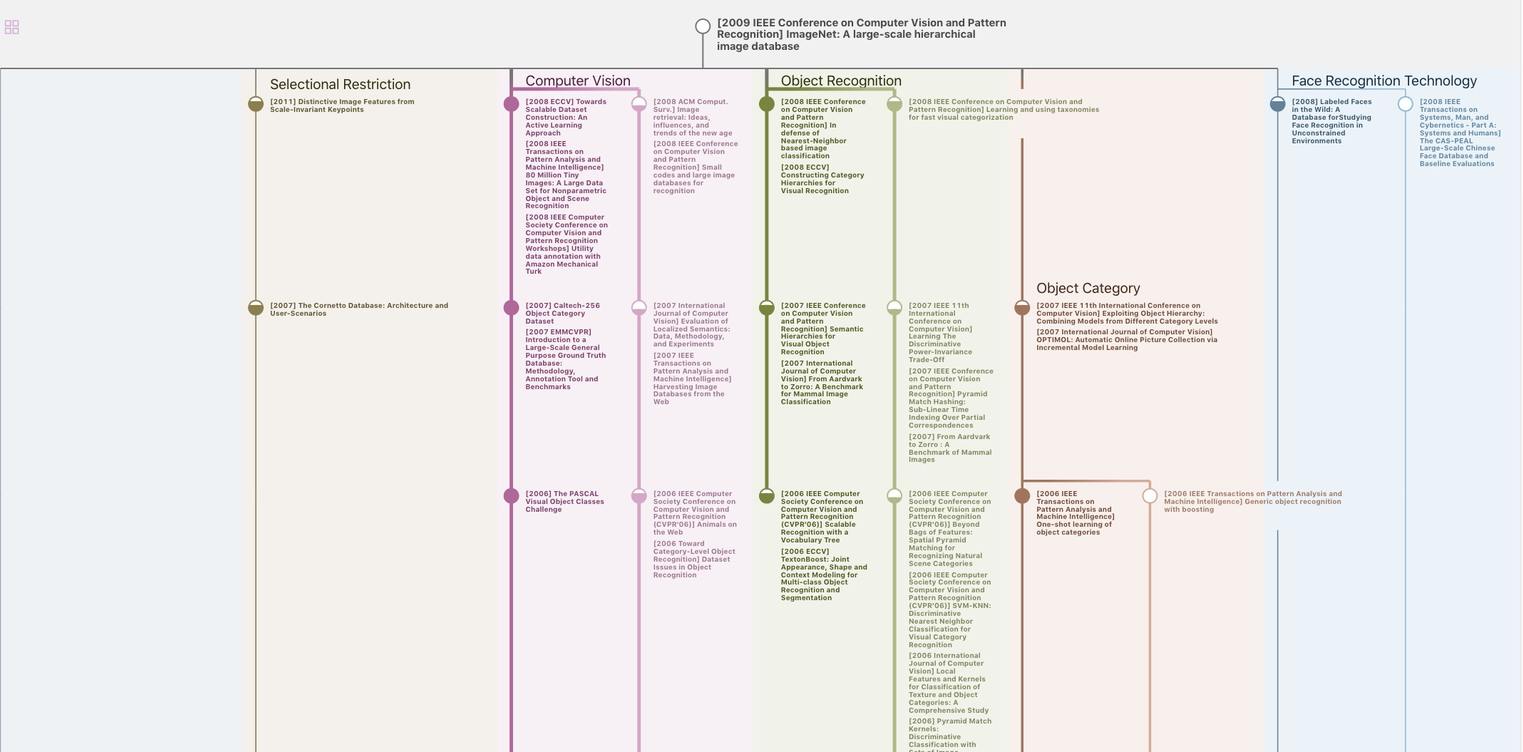
生成溯源树,研究论文发展脉络
Chat Paper
正在生成论文摘要