Measuring metrics: what biodiversity indicators are most appropriate for different forms of data bias
crossref(2023)
摘要
AbstractAimBiodiversity metrics have become a ubiquitous component of conservation assessments across scales. However, whilst indices have become increasingly widely used, their ability to perform in different conditions has remained largely untested under realistic conditions, especially recently when citizen science data are increasingly dominant; understanding how to use them effectively and circumvent new biases is essential. Data in the real world are frequently biased, so we must understand the ability of these metrics to reconcile genuine patterns in the face of prevalent biases such as small sample size, variable coverage levels and accessibility induced biases. LocationGlobalTime periodPresentMajor taxa studiedBirdsMethodsHere, we examined a suite of popular metrics under multiple simulations (based on analysis of biases within eBird data) and assess the ability of these metrics to reconstruct the original patterns based on subsampling birdlife data to simulate real biases using R. We then use predictive modelling to model bird diversity globally and overcome biasesResultsPerformance was highly variable across the different types of biases, but in many instances Simpson’s index performs best, followed by Hill numbers, whereas Pielou’s was almost universally worst. From standardised tests, we then applied these metrics to eBird data using 611,520,112 samples of 10,359 species of bird (around 88% of known species), to reconstruct global diversity patterns at five and ten km resolutions. However, when we map out richness using Maxent based on these indices, Simpson’s generally over-predicted diversity in many areas, whereas Hill numbers are more conservative. Main conclusionsBased on an average of the better metrics can map out diversity across scales and overcome biases accurately predicting richness patterns even for data-poor areas. Going forward, this workflow will enable standardized best practices for richness mapping based on a clear understanding of the performance of different metrics.
更多查看译文
AI 理解论文
溯源树
样例
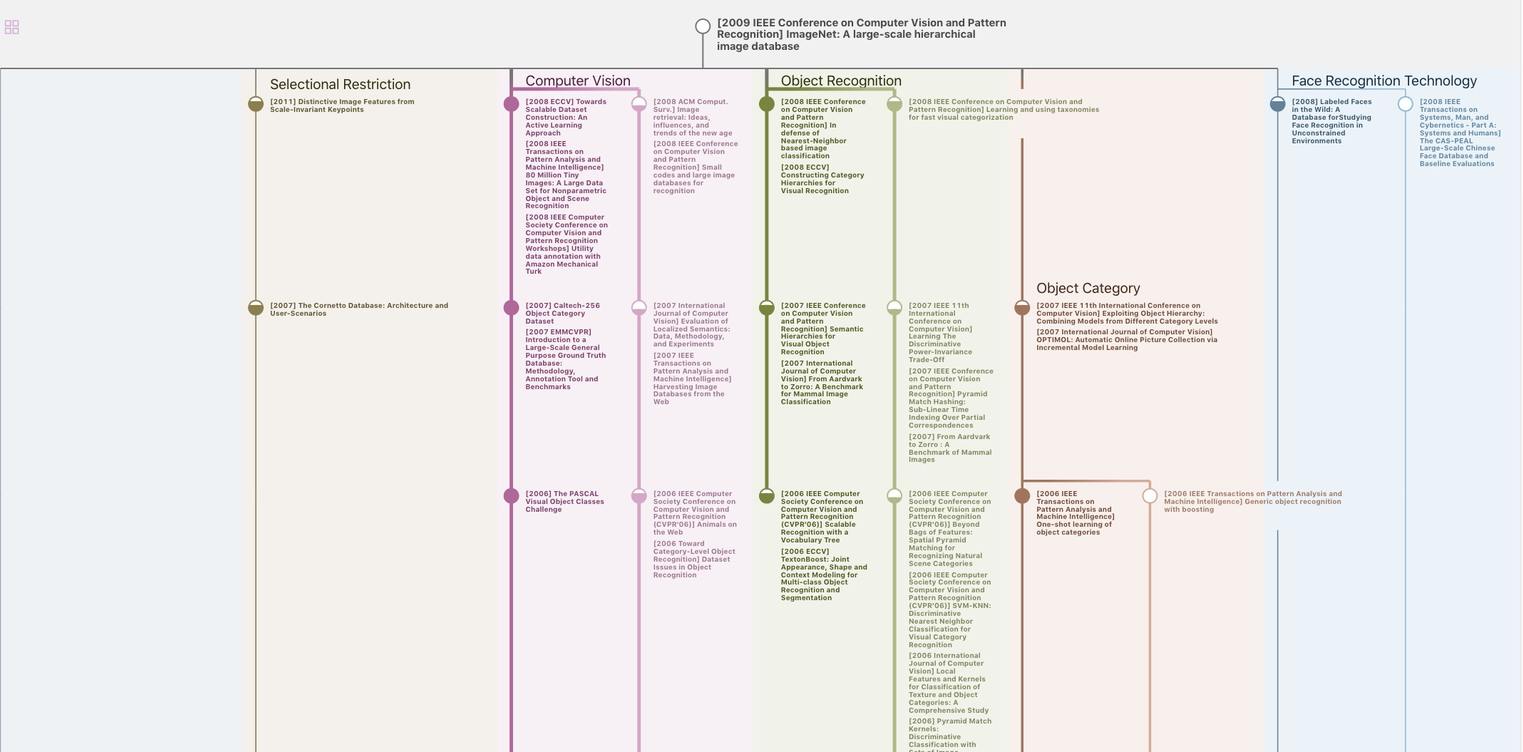
生成溯源树,研究论文发展脉络
Chat Paper
正在生成论文摘要