A new method based on YOLOv5 for remote sensing object detection
2022 China Automation Congress (CAC)(2022)
摘要
Objects on remote sensing images usually have the characteristics of complex background and extremely large scale changes. This makes most general object detection algorithms designed to cross-scale datasets unable to achieve ideal results in remote sensing images. In this article, we propose a new method based on improved YOLO network to solve the difficulties mentioned above. We introduce a new module to extract multi-scale information to enhance the ability of the network to detect objects with different scales in complex background and a new FPN named AG-PAN which use two top-down pathways to compensate for semantic information loss and attention mechanism to focus on the distinctive features of the objects. Soft-CIoU-NMS be used to replace the commonly used NMS to generate better detection result. Extensive experiments are conducted to demonstrate the effectiveness of our approach on the COCO, RSOD (Remote Sensing Object Detection) dataset. The results show that our method outperforms original YOLOv5 by 3.1% AP
50
and other state-of-the-art methods in RSOD. In COCO dataset, our method has 2.6% improvement in AP and 2.9% improvement in AP
50
. The experimental results show that our method significantly improves the performance of the object detection algorithm in remote sensing images.
更多查看译文
关键词
object detection,remote sensing images,attention mechanism,convolutional neural network
AI 理解论文
溯源树
样例
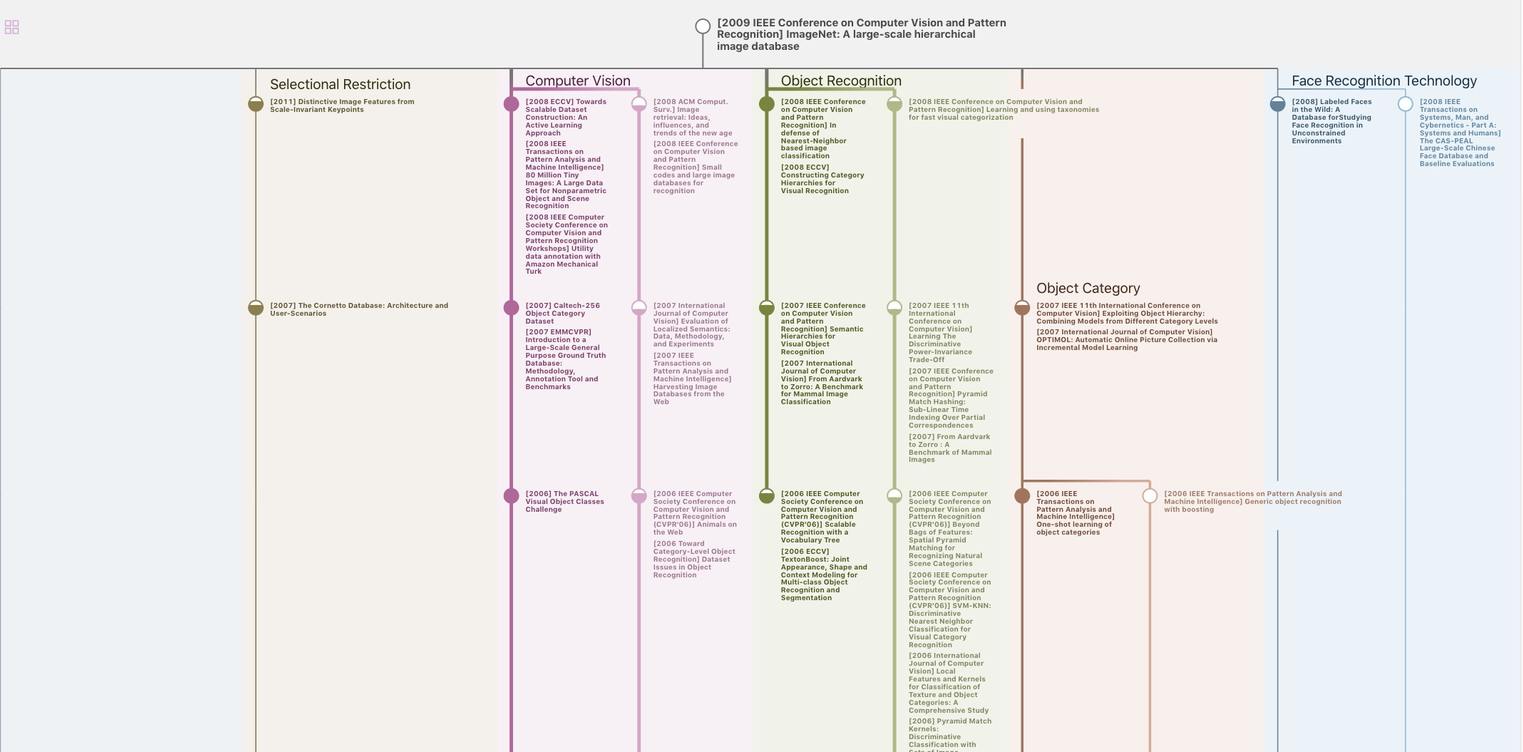
生成溯源树,研究论文发展脉络
Chat Paper
正在生成论文摘要