Complex Industrial Process Monitoring Using Multi-scale Principal Component Analysis-Kernel Entropy Component Analysis
2022 China Automation Congress (CAC)(2022)
摘要
Modern industrial processes are characterized by numerous measured points and wide operation ranges, resulting in extremely complex correlations among variables. Therefore, an effective monitoring system should balance diverse process characteristics such as nonlinearity, non-Gaussianity and multiscale simultaneously. In this paper, a new hybrid statistical modeling method, namely multi-scale principal component analysis - kernel entropy component analysis (MSPCA-KECA), is proposed for complex industrial process monitoring. Firstly, MSPCA is used to eliminate the auto- and cross- correlations among variables and extract linear features out of the raw data. Then, KECA is performed to capture nonlinear non-Gaussian features from the residual space resulting from MSPCA. With the application of MSPCA-KECA, the original data space is decomposed into three subspaces. On this basis, the combined statistic $T_c^2$ is constructed to monitor the variation in both the linear and nonlinear dominant subspaces, and the Q statistic is used to measure the amount of variation in residuals. Finally, the effectiveness of the proposed MSPCA-KECA is evaluated through the Tennessee Eastman (ТЕ) process, which presents better monitoring performance in comparison with some advanced hybrid modeling methods.
更多查看译文
关键词
process monitoring,multi-scale principal component analysis (MSPCA),kernel entropy component analysis (KECA)
AI 理解论文
溯源树
样例
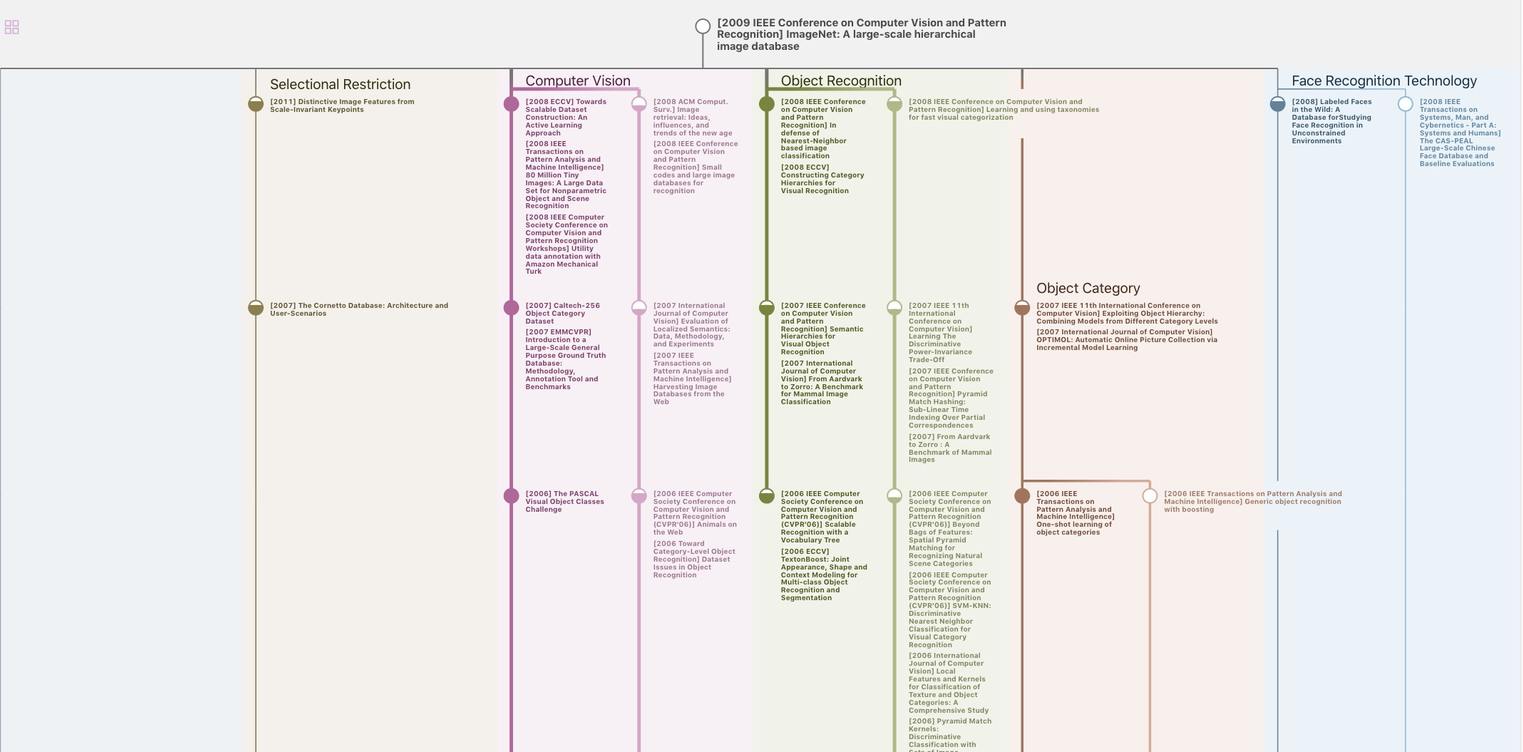
生成溯源树,研究论文发展脉络
Chat Paper
正在生成论文摘要