Expressivity Enhancement with Efficient Quadratic Neurons for Convolutional Neural Networks
CoRR(2023)
摘要
Convolutional neural networks (CNNs) have been successfully applied in a range of fields such as image classification and object segmentation. To improve their expressivity, various techniques, such as novel CNN architectures, have been explored. However, the performance gain from such techniques tends to diminish. To address this challenge, many researchers have shifted their focus to increasing the non-linearity of neurons, the fundamental building blocks of neural networks, to enhance the network expressivity. Nevertheless, most of these approaches incur a large number of parameters and thus formidable computation cost inevitably, impairing their efficiency to be deployed in practice. In this work, an efficient quadratic neuron structure is proposed to preserve the non-linearity with only negligible parameter and computation cost overhead. The proposed quadratic neuron can maximize the utilization of second-order computation information to improve the network performance. The experimental results have demonstrated that the proposed quadratic neuron can achieve a higher accuracy and a better computation efficiency in classification tasks compared with both linear neurons and non-linear neurons from previous works.
更多查看译文
关键词
efficient quadratic neurons,convolutional neural networks,enhancement,neural networks
AI 理解论文
溯源树
样例
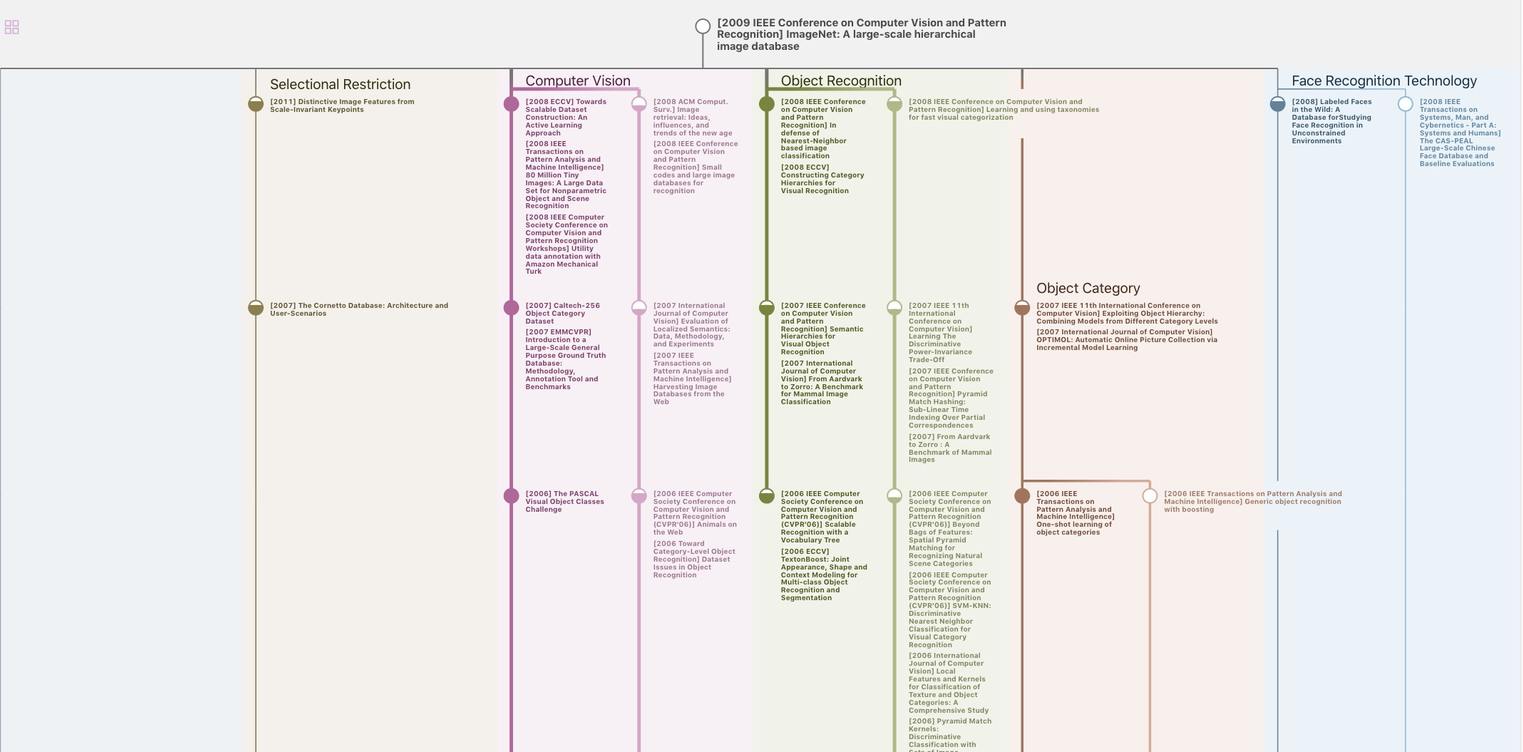
生成溯源树,研究论文发展脉络
Chat Paper
正在生成论文摘要