Wavelet Transforms and Machine Learning Methods for the Study of Turbulence
crossref(2023)
摘要
This article examines the wavelet transforms and the machine learning methods in accelerating large eddy simulations of turbulent flows. An overarching challenge of large eddy simulations is to accurately represent the cascade of kinetic energy across the cut-off length scale. Taylor hypothesis suggests that the energy cascade occurs through the process of vortex stretching. However, Richardson hypothesis assumes the self-amplification of the strain field and a hierarchical break down of large eddies lead the energy cascade. The large eddy simulations typically employs the self-amplification of the strain in formulating subgrid models. However, several studies also proposed subgrid models based on vortex stretching. The wavelet-based coherent vortex simulation of turbulence directly accounts for vortex stretching in overall forward scatter of energy, while allowing local backscatter. The wavelet-based large eddy simulation adapts the grid to capture the creation of small-scale eddies, while adopting subgrid models based on the self-amplification of strain. The advancement of artificial intelligence in turbulence modelling is currently evolving around accelerating the numerical simulations of turbulent flow. However, there is a clear connection between the application of wavelet transfroms and neural networks for directly solving the Navier-Stokes equation, indicating some potential benefits of wavelet methods over the neural networks.
更多查看译文
AI 理解论文
溯源树
样例
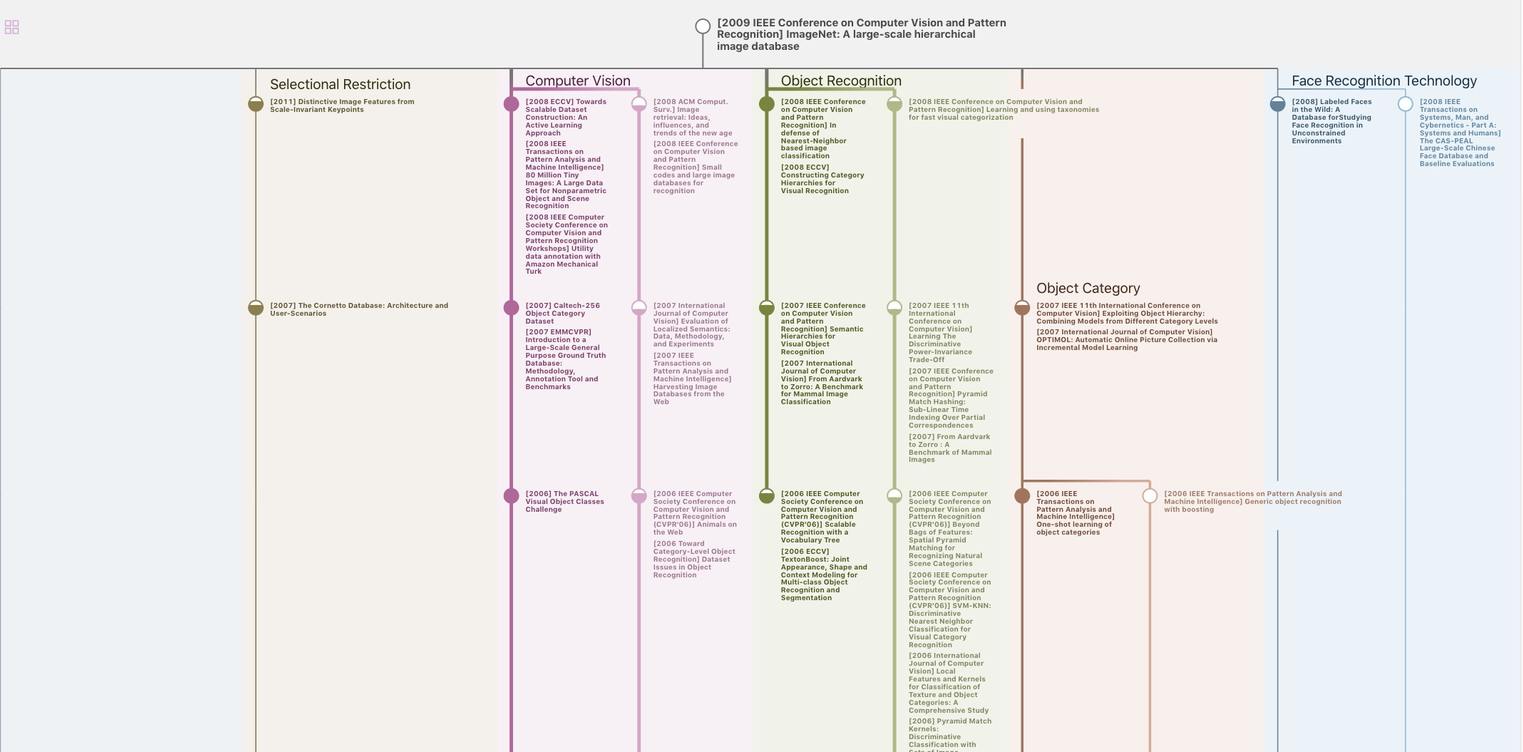
生成溯源树,研究论文发展脉络
Chat Paper
正在生成论文摘要