Understanding the Effect of the Long Tail on Neural Network Compression
CoRR(2023)
关键词
Backpropagation Learning,Recurrent Neural Networks,Deep Learning,Large-Scale Optimization,Feedforward Neural Networks
AI 理解论文
溯源树
样例
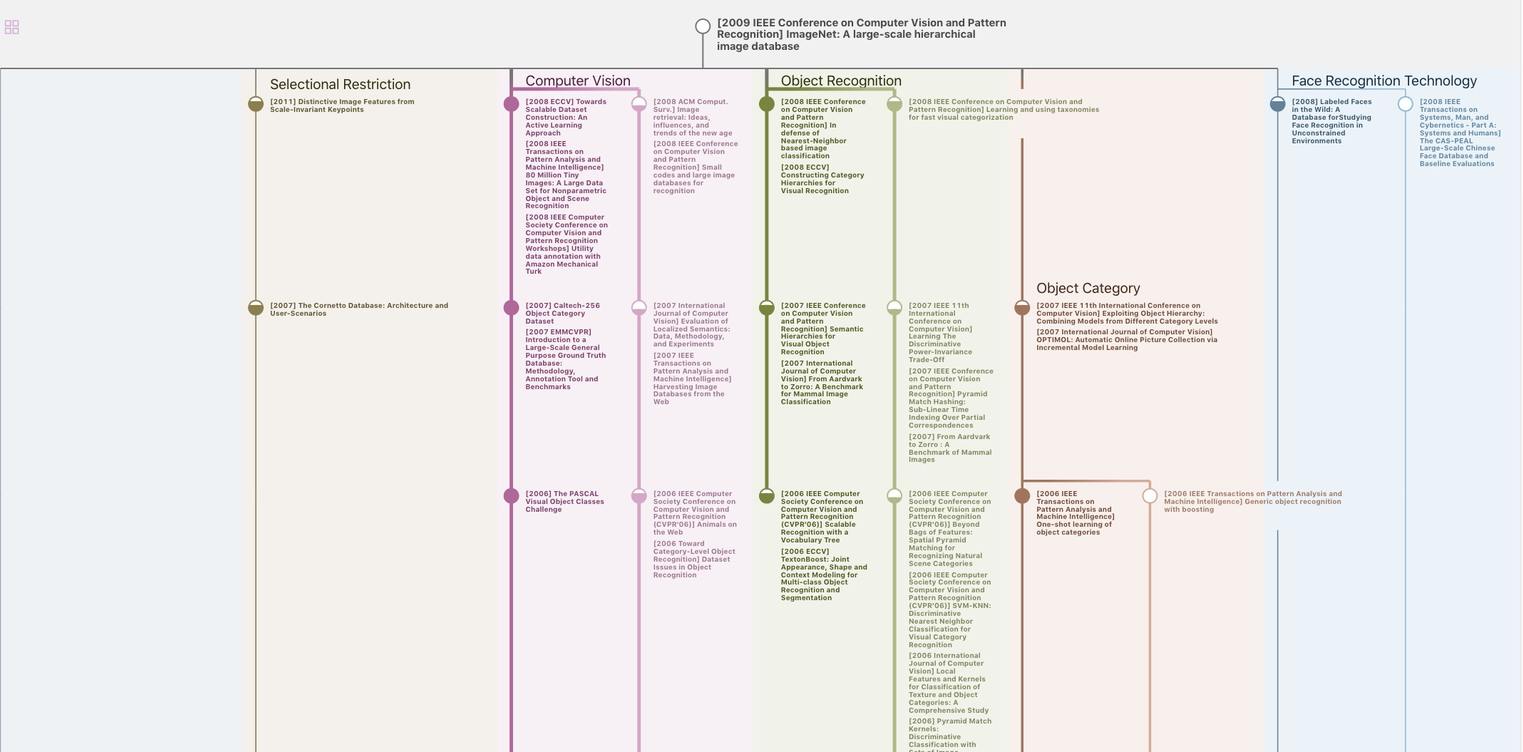
生成溯源树,研究论文发展脉络
Chat Paper
正在生成论文摘要