Epitopological Sparse Ultra-Deep Learning: A Brain-Network Topological Theory Carves Communities in Sparse and Percolated Hyperbolic ANNs
crossref(2023)
摘要
Sparse training (ST) aims to improve deep learning by replacing fully connected artificial neural networks (ANNs) with sparse ones, akin to the structure of brain networks. Therefore, it might benefit to borrow brain-inspired learning paradigms from complex network intelligence theory. Epitopological learning (EL) is a field of network science that studies how to implement learning on networks by changing the shape of their connectivity structure (epitopological plasticity). One way to implement EL is via link prediction: predicting the existence likelihood of nonobserved links in a network. Cannistraci-Hebb (CH) learning theory inspired the CH3-L3 network automata rule for link prediction which is effective for generalpurpose link prediction. Here, starting from CH3-L3 we propose Epitopological Sparse Ultra-deep Learning (ESUL) to apply EL into sparse training. In empirical experiments, we find that ESUL learns ANNs with sparse hyperbolic topology in which emerges a community layer organization that is ultra-deep (meaning that also each layer has an internal depth due to power-law node hierarchy). Furthermore, we discover that ESUL automatically sparse the neurons during training (arriving even to 30% neurons left in hidden layers), this process of node dynamic removal is called percolation. Then we design CH training (CHT), a training methodology that put ESUL at its heart, with the aim to enhance prediction performance. CHT consists of 4 parts: (i) correlated sparse topological initialization (CSTI), to initialize the network with a hierarchical topology; (ii) sparse weighting initialization (SWI), to tailor weights initialization to a sparse topology; (iii) ESUL, to shape the ANN topology during training; (iv) early stop with weight refinement, to tune only weights once the topology reaches stability. We conduct experiments on 6 datasets and 3 network structures (MLPs, VGG16, Transformer) comparing CHT to sparse training SOTA method and fully connected network. By significantly reducing the node size while retaining performance, CHT represents the first example of parsimony sparse training.
更多查看译文
AI 理解论文
溯源树
样例
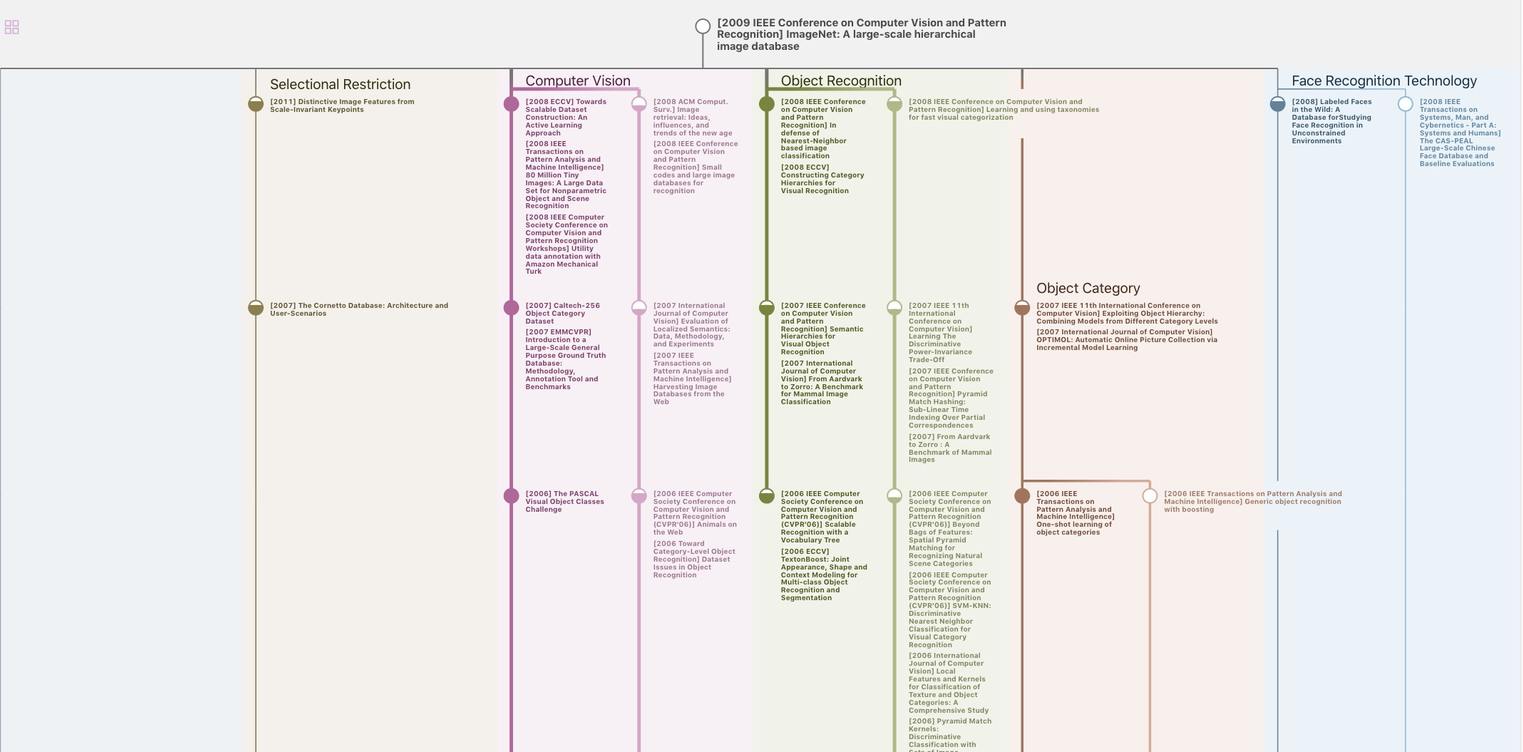
生成溯源树,研究论文发展脉络
Chat Paper
正在生成论文摘要