DeepStay: Stay Region Extraction from Location Trajectories using Weak Supervision
CoRR(2023)
摘要
Nowadays, mobile devices enable constant tracking of the user's position and location trajectories can be used to infer personal points of interest (POIs) like homes, workplaces, or stores. A common way to extract POIs is to first identify spatio-temporal regions where a user spends a significant amount of time, known as stay regions (SRs). Common approaches to SR extraction are evaluated either solely unsupervised or on a small-scale private dataset, as popular public datasets are unlabeled. Most of these methods rely on hand-crafted features or thresholds and do not learn beyond hyperparameter optimization. Therefore, we propose a weakly and self-supervised transformer-based model called DeepStay, which is trained on location trajectories to predict stay regions. To the best of our knowledge, this is the first approach based on deep learning and the first approach that is evaluated on a public, labeled dataset. Our SR extraction method outperforms state-of-the-art methods. In addition, we conducted a limited experiment on the task of transportation mode detection from GPS trajectories using the same architecture and achieved significantly higher scores than the state-of-the-art. Our code is available at https://github.com/christianll9/deepstay.
更多查看译文
关键词
Weak Supervision,Location Trajectory,Deep Learning,Hyperparameters,Hyperparameter Tuning,User Trajectory,Time Series,Training Set,Training Data,Final Results,Convolutional Neural Network,Local Point,Action Recognition,Transformer Model,Consecutive Points,Latent Representation,Self-supervised Learning,Trajectory Data,OpenStreetMap,Sine And Cosine,Weak Labels,Raw Trajectories,Transformer Encoder,Time Series Segments,Feed-forward Layer,Density-based Clustering,Pretext Task,Autoencoder
AI 理解论文
溯源树
样例
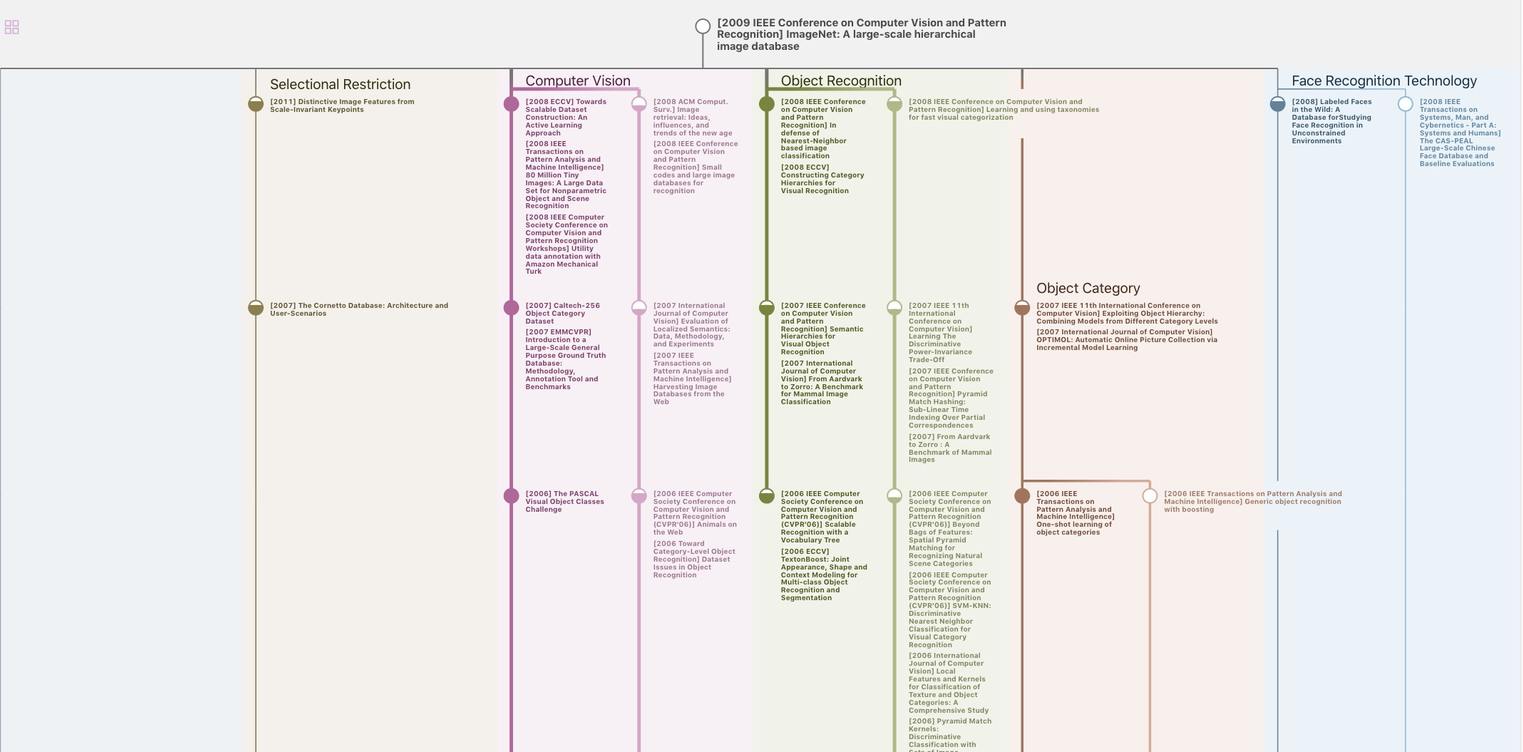
生成溯源树,研究论文发展脉络
Chat Paper
正在生成论文摘要