Dual-Modality Co-Learning for Unveiling Deepfake in Spatio-Temporal Space
ICMR '23: Proceedings of the 2023 ACM International Conference on Multimedia Retrieval(2023)
摘要
The emergence of photo-realistic deepfakes on a large scale has become a significant societal concern, which has garnered considerable attention from the research community. Several recent studies have identified the critical issue of “temporal inconsistency” resulting from the frame reassembling process of deepfake generation techniques. However, due to the lack of task-specific design, the spatio-temporal modeling of current methods remains insufficient in three critical aspects: 1) inapparent temporal changes are prone to be undermined compared to abundant spatial cues; 2) minor inconsistent regions are often concealed by motions with greater amplitude during downsampling; 3) capturing both transient inconsistencies and persistent motions simultaneously remains a significant challenge. In this paper, we propose a novel Dual-Modality Co-Learning framework tailored for these characteristics, which achieves more effectual deepfake detection with complementary information from RGB and optical flow modalities. In particular, we designed a Multi-Scale Motion Regularization module to encourage the network to equally prioritize both the significant spatial cues and the subtle temporal facial motion cues. Additionally, we developed a Multi-Span Cross-Attention module to effectively integrate the information from both RGB and optical flow modalities and improve the detection accuracy with multi-span predictions. Extensive experiments validate the effectiveness our ideas and demonstrate the superior performance of our approach.
更多查看译文
关键词
Deepfake Detection, Digital Forensics, Spatio-Temporal Analysis
AI 理解论文
溯源树
样例
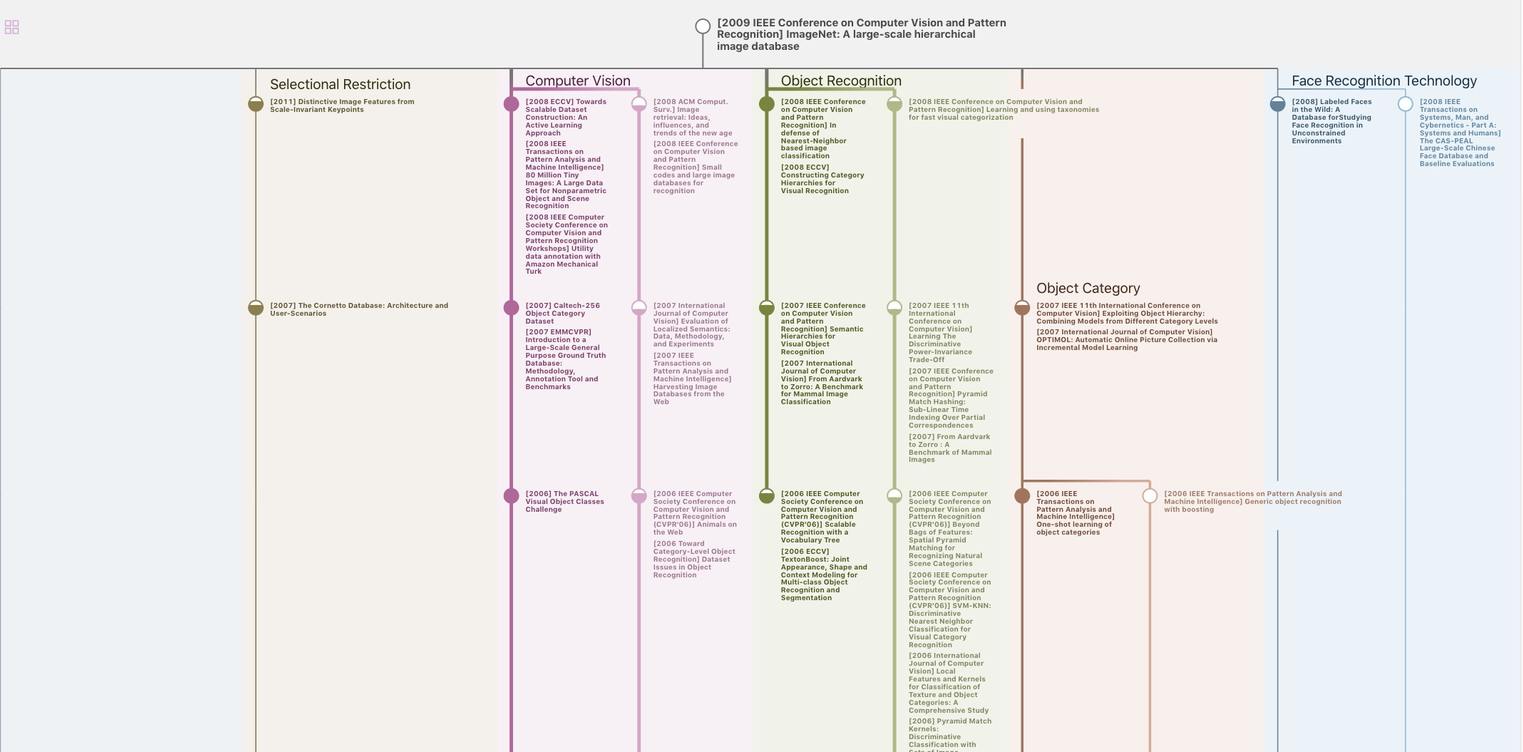
生成溯源树,研究论文发展脉络
Chat Paper
正在生成论文摘要