Demand Response Potential Day-ahead Forecasting Approach Based on LSSA-BPNN Considering the Electricity-carbon Coupling Incentive Effects
IEEE Transactions on Industry Applications(2024)
摘要
In order to address the challenges of global climate change and to achieve the goals of sustainable development, the need for a low-carbon transition of the power system is becoming more urgent. However, previous demand response (DR) potential forecasting models for load aggregators (LAs) only consider the potential for customers to adjust their loads, without fully exploiting the potential to reduce their carbon emissions. To this end, a logistic sparrow search algorithm- back propagation neural network (LSSA-BPNN) based DR potential and carbon reduction potential forecasting model for LAs is proposed in this paper, which considers the dual incentive of electricity and carbon. First, a novel home energy management system (HEMS) model is developed, which considers the dual incentive of electricity and carbon. The model's objective function consists of two components: minimizing economic costs and reducing carbon emissions. Weight coefficients are introduced to characterize the power consumption preferences of different customer types. Subsequently, simulations are conducted to generate data related to DR potential and carbon reduction potential of LAs. Second, the multiple influencing features of DR potential and carbon reduction potential are sorted according to the degree of importance by the RF model respectively, which could reduce the redundancy of input features. Finally, based on the selected features, the LSSA-BPNN model is established to obtain the forecasting results of the DR potential and carbon reduction potential for LAs. The effectiveness and superiority of the proposed model have been verified using a real dataset in Austin
更多查看译文
关键词
Demand response,Potential forecasting, Electricity-carbon Coupling,Load aggregator,Low-carbon
AI 理解论文
溯源树
样例
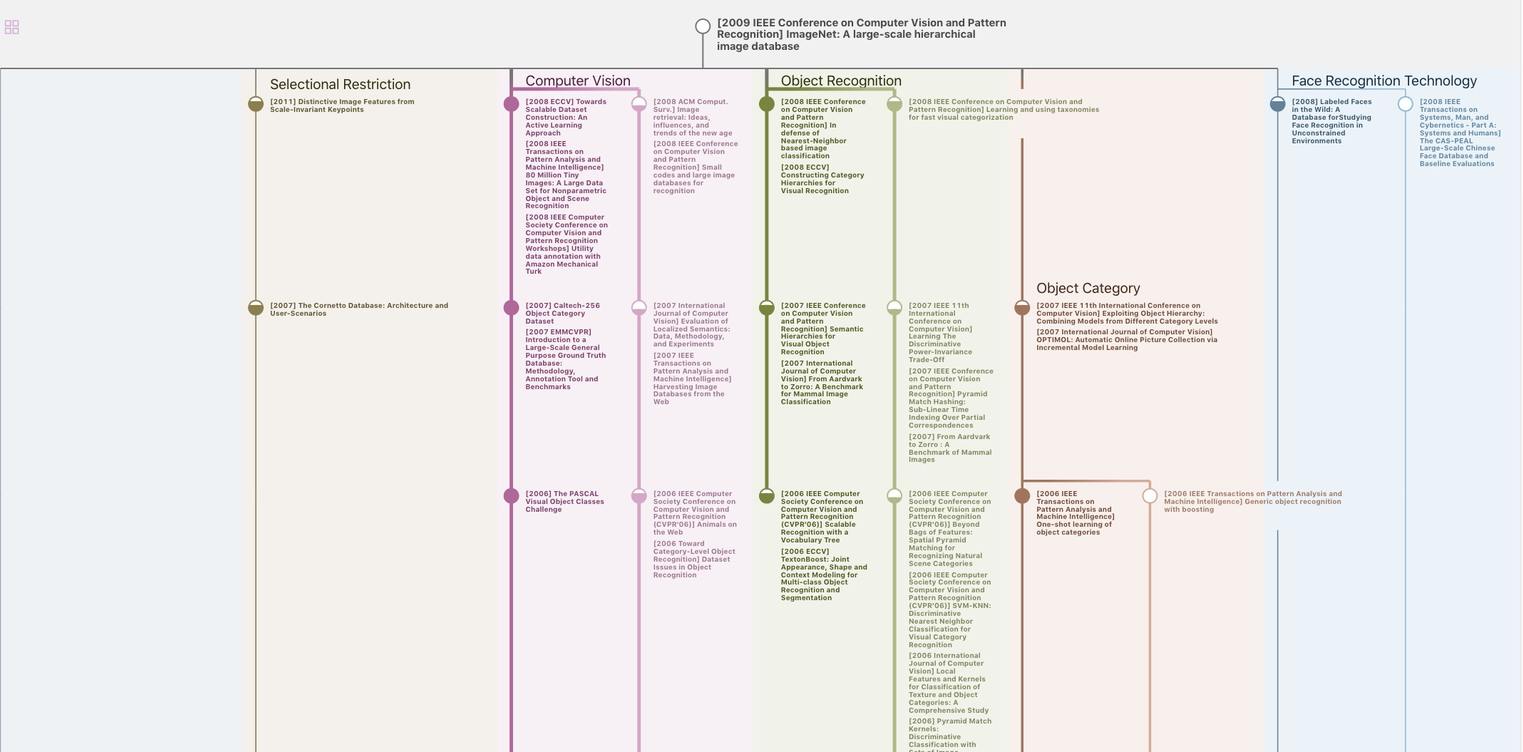
生成溯源树,研究论文发展脉络
Chat Paper
正在生成论文摘要