Multi-Label ECG Classification using Temporal Convolutional Neural Network
arXiv (Cornell University)(2023)
摘要
Automated analysis of 12-lead electrocardiogram (ECG) plays a crucial role in the early screening and management of cardiovascular diseases (CVDs). In practice, it is common to see multiple co-occurring cardiac disorders, i.e., multi-label or multimorbidity in patients with CVDs, which increases the risk for mortality. Most current research focuses on the single-label ECG classification, i.e., each ECG record corresponds to one cardiac disorder, ignoring ECG records with multi-label phenomenon. In this paper, we propose an ensemble of attention-based temporal convolutional neural network (ATCNN) models for the multi-label classification of 12-lead ECG records. Specifically, a set of ATCNN-based single-lead binary classifiers are trained one for each cardiac disorder, and the predictions from these classifiers with simple thresholding generate the final multi-label decisions. The ATCNN contains a stack of TCNN layers followed by temporal and spatial attention layers. The TCNN layers operate at different dilation rates to represent the multi-scaled pathological ECG features dynamics, and attention layers emphasize/reduce the diagnostically relevant/redundant 12-lead ECG information. The proposed framework is evaluated on the PTBXL-2020 dataset and achieved an average F1-score of 76.51%. Moreover, the spatial and temporal attention weights visualization provides the optimal ECG-lead subset selection for each disease and model interpretability results, respectively. The improved performance and interpretability of the proposed approach demonstrate its ability to screen multimorbidity patients and help clinicians initiate timely treatment.
更多查看译文
关键词
temporal convolutional neural
AI 理解论文
溯源树
样例
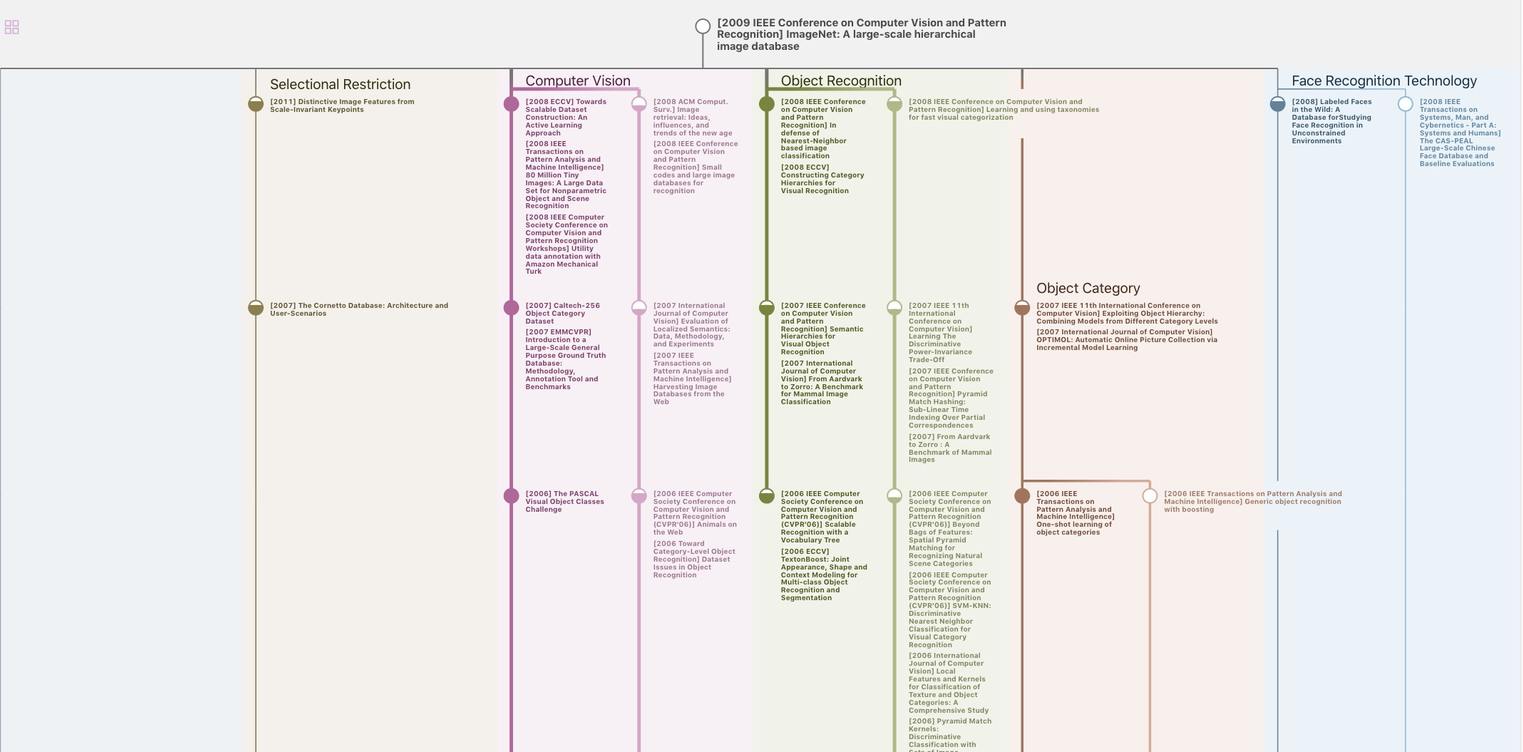
生成溯源树,研究论文发展脉络
Chat Paper
正在生成论文摘要