SDR-Implementation of a Support Vector Machine-Assisted Covariance-Based Spectrum Sensing Algorithm in the Presence of Correlated Noise
IEEE Sensors Letters(2023)
摘要
Cognitive radio (CR) is an emergent technology that is considered for more efficient spectrum utilization. Spectrum sensing (SS), i.e., detection of primary user (PU) in the frequency band, is one of the key tasks of cognitive radio systems. Recently, various works on the support vector machine (SVM)-assisted covariance-based spectrum sensing (CSS) in multireceiver systems were presented and evaluated with computer simulations. However, the real environment is different, especially since the noise is often assumed to be spatially uncorrelated, while it is correlated in reality. Therefore, we propose a four-receivers software-defined radio (SDR)-based test platform for experimental evaluation of SVM-assisted CSS techniques. Furthermore, we implement an SVM-assisted CSS technique in the proposed platform to assess its performance when two and four receivers are activated. The measurements showed that increasing the number of receiving channels does indeed enhance the detection probability. Moreover, we evaluated the algorithm in both white and correlated noise scenarios. The evaluation demonstrates how the presence of correlated noise deteriorated the performance of the SVM-assisted CSS compared with the case where only white noise is present. More specifically, the simulations showed that to reach 99% detection probability when noise correlation is present, the implemented algorithm requires an signal-to-noise ratio (SNR) value that is 10-dB greater than the one required when only white noise is present.
更多查看译文
关键词
Sensor signal processing,covariance matrix,machine learning,software-defined radio,spectrum sensing (SS),support vector machine (SVM)
AI 理解论文
溯源树
样例
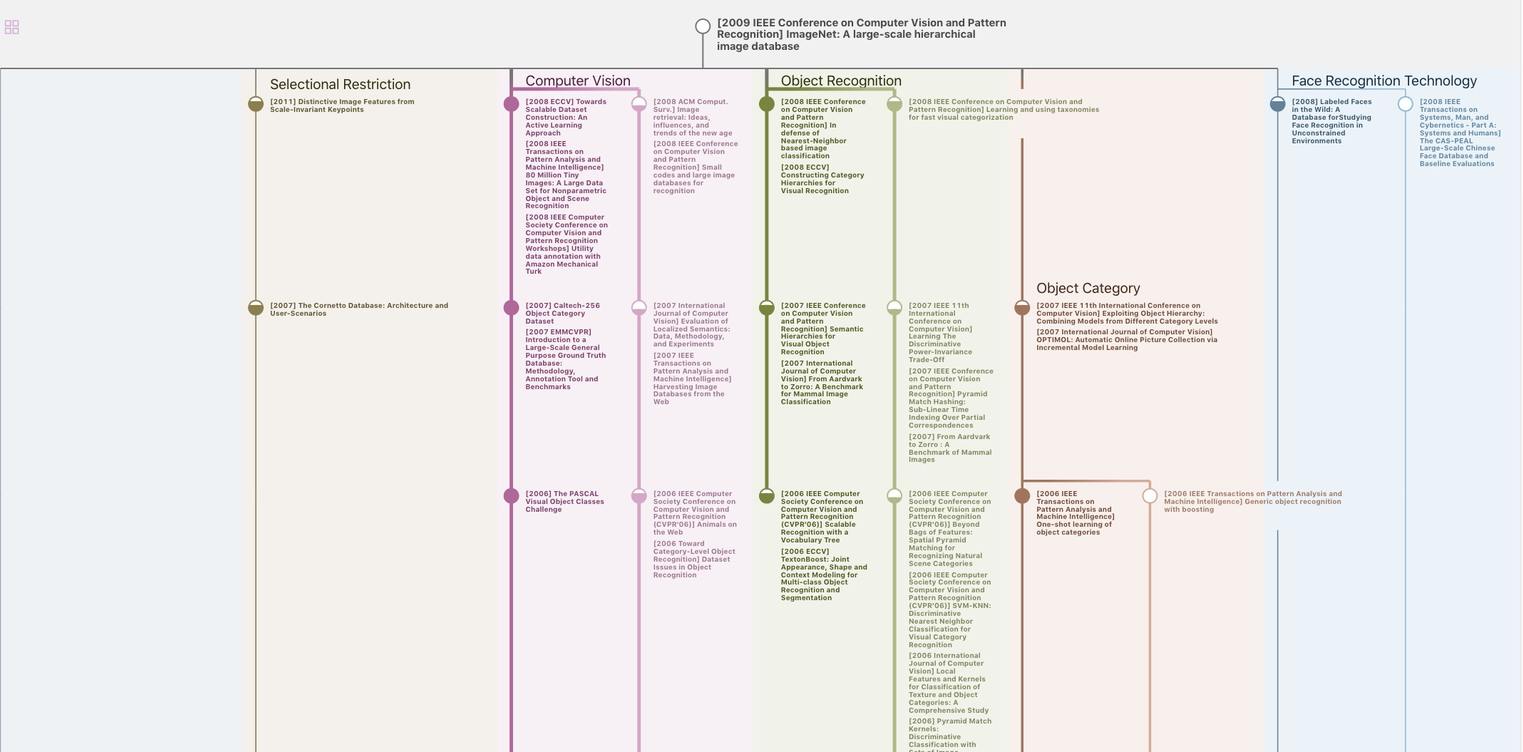
生成溯源树,研究论文发展脉络
Chat Paper
正在生成论文摘要