Data-Driven Methods for Predicting the State of Health, State of Charge, and Remaining Useful Life of Li-Ion Batteries: A Comprehensive Review
International Journal of Precision Engineering and Manufacturing(2023)
摘要
Lithium-ion batteries are widely used in electric vehicles, electronic devices, and energy storage systems owing to their high energy density, long life, and outstanding performance. However, various internal and external factors affect the battery performance, leading to deterioration and ageing. Accurately estimating the state of health (SOH), state of charge (SOC), and remaining useful life (RUL) of batteries is challenging owing to complex operating characteristics and changing internal physical parameters. With the increasing availability of shared battery data and improved computer performance, the use of data-driven methods for battery health estimations and RUL predictions has gained popularity. We provide a comprehensive review of several studies in which data-driven methods were used for SOC and SOH estimation and RUL prediction. Specifically, we focus on the importance of open battery-cycling databases, various prediction methods used, and results obtained using each of these methods. Moreover, we aim to facilitate further research by providing a comprehensive description of the current state-of-the-art methods employed in battery health estimation and RUL prediction using open databases and machine-learning algorithms. Thus, we hope that this review will help researchers to develop accurate and reliable predictive models for battery health assessment in the future.
更多查看译文
关键词
Lithium-ion batteries,State of charge,State of health,Remaining useful life,Data-driven method
AI 理解论文
溯源树
样例
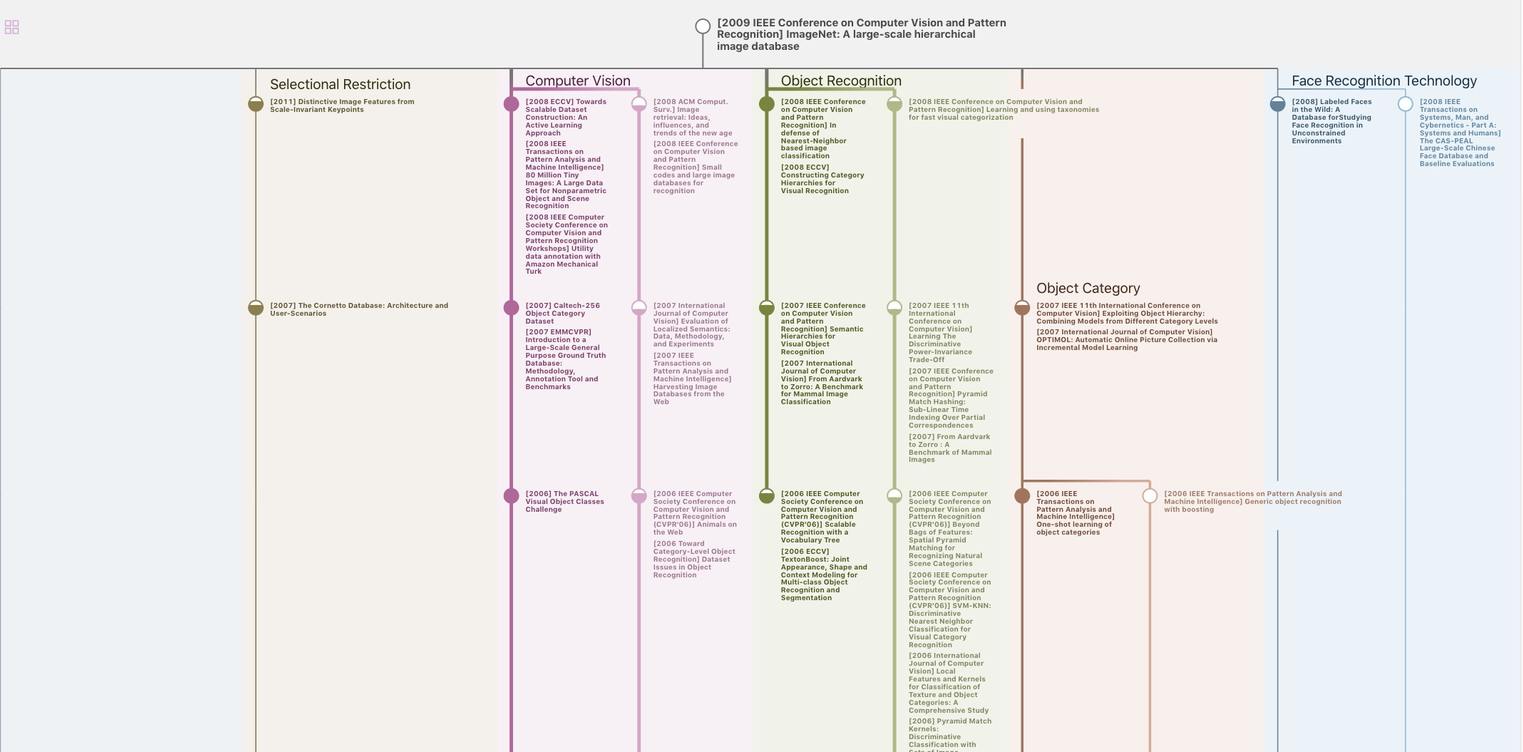
生成溯源树,研究论文发展脉络
Chat Paper
正在生成论文摘要