Facial expression recognition network with slow convolution and zero-parameter attention mechanism
OPTIK(2023)
摘要
Facial expression recognition (FER) has been successfully applied to the fields of automatic driving, classroom education, and many other industries. However, a lot of existing problems induced by different lighting, posture, and skin color make many difficulties in extracting effective features, resulting in low accuracy of FER. to address these issues, a novel slow evolution and zero parameter attention mechanism (SC-ZAM) is proposed to reliably extract discriminative feature from facial images for FER. In the proposed SC-ZAM, Slow convolution module is used to reduce the speed of image dimensionality reduction, extract considerably reliable facial features, and enhances the representation of these features to extract more effective global information of facial expressions. Meanwhile, zero-parameter attention modules are applied to enhance the focus on the local discriminative feature areas. The improved attention module focuses on highly distinguishable facial features and restrains the weight of non-facial areas to locate more detailed local facial areas in the global information extracted from the network. Experimental results demonstrate that our SC-ZAM method is robust against different FER tasks on our infant dataset and several public datasets, and is superior to other state-of-the-art methods.
更多查看译文
关键词
Facial expression recognition,Infant,Deep learning,Attention module
AI 理解论文
溯源树
样例
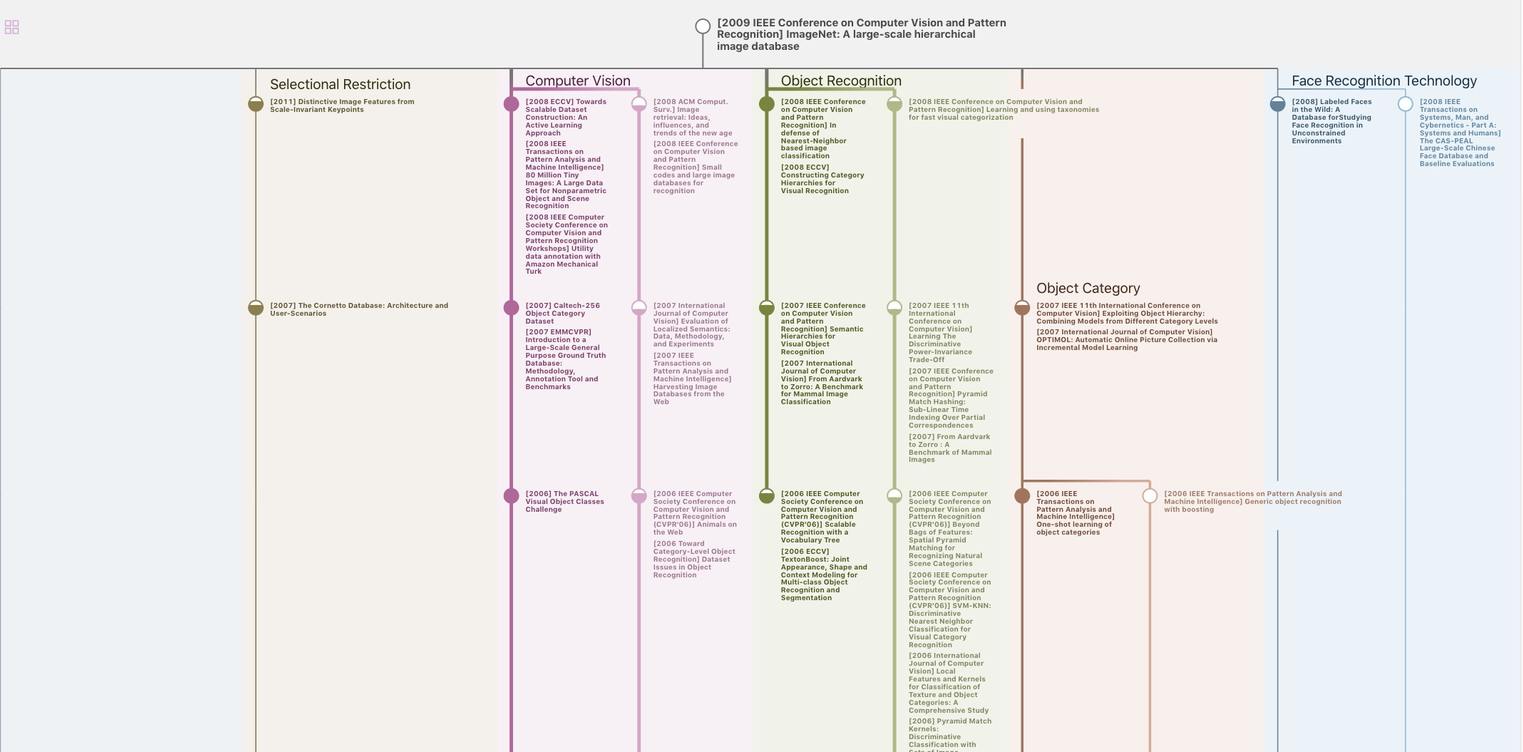
生成溯源树,研究论文发展脉络
Chat Paper
正在生成论文摘要