Vision-language models boost food composition compilation
CoRR(2023)
摘要
Nutrition information plays a pillar role in clinical dietary practice, precision nutrition, and food industry. Currently, food composition compilation serves as a standard paradigm to estimate food nutrition information according to food ingredient information. However, within this paradigm, conventional approaches are laborious and highly dependent on the experience of data managers, they cannot keep pace with the dynamic consumer market and resulting in lagging and missing nutrition data and earlier machine learning methods unable to fully understand food ingredient statement information or ignored the characteristic of food image. To this end, we developed a novel vision-language AI model, UMDFood-VL, using front-of-package labeling and product images to accurately estimate food composition profiles. In order to drive such large model training, we established UMDFood-90k, the most comprehensive multimodal food database to date. The UMDFood-VL model significantly outperformed convolutional neural networks (CNNs) and recurrent neural networks (RNNs) on a variety of nutrition value estimations. For instance, we achieved macro-AUCROC up to 0.921 for fat value estimation, which satisfied the practice requirement of food composition compilation. This performance shed the light to generalize to other food and nutrition-related data compilation and catalyzed the evolution of other food applications.
更多查看译文
AI 理解论文
溯源树
样例
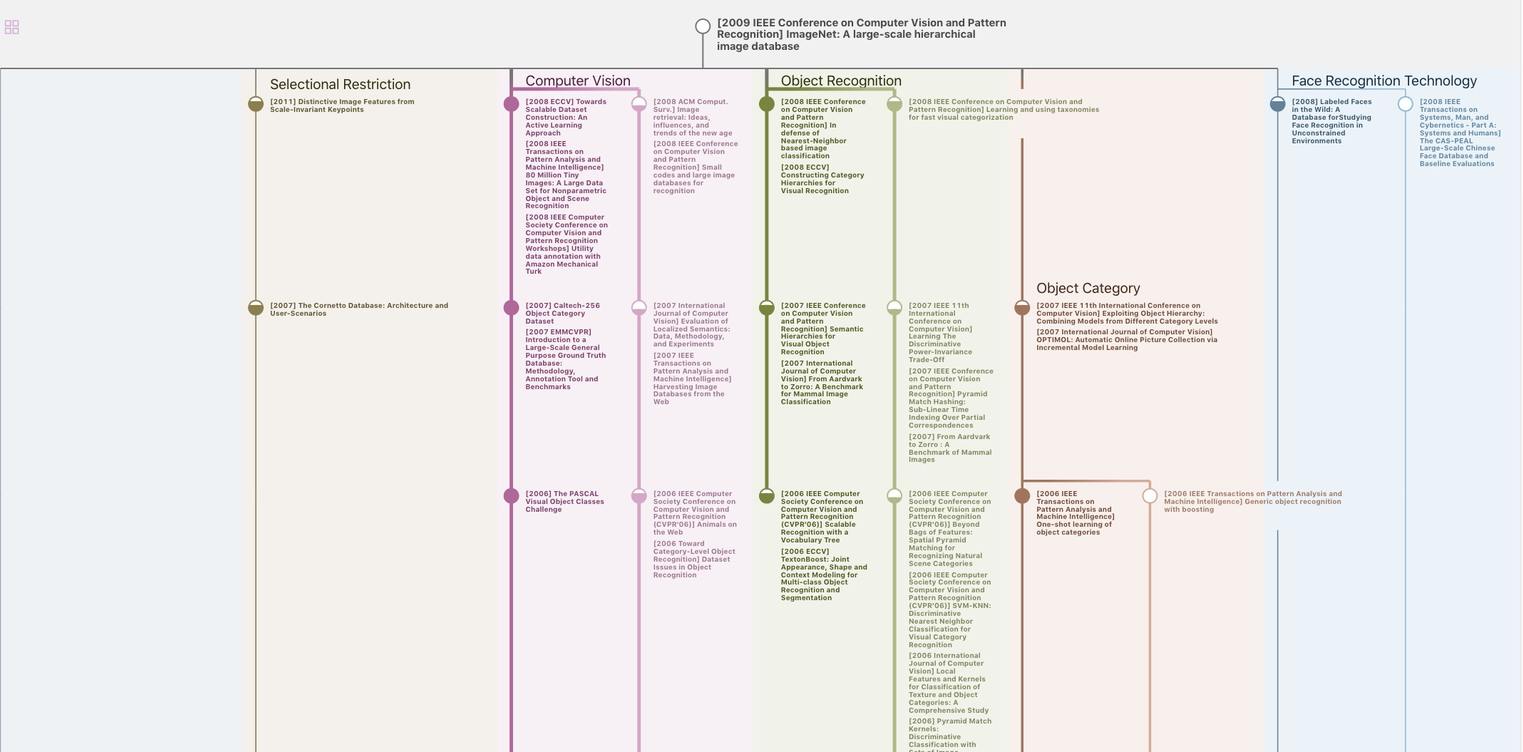
生成溯源树,研究论文发展脉络
Chat Paper
正在生成论文摘要